Revolutionize structural engineering with AI-powered analysis and design. Transform blueprints into intelligent solutions in minutes. (Get started for free)
Optimizing Antenna Gain 7 Practical Techniques for Enhanced Signal Reception
Optimizing Antenna Gain 7 Practical Techniques for Enhanced Signal Reception - Enhancing Antenna Efficiency through Metamaterial Integration
Metamaterials are revolutionizing antenna design by introducing novel electromagnetic properties that can drastically boost antenna efficiency and gain. We've seen impressive results from designs employing metamaterials, like those using near-zero index materials and engineered superstrates. Some antenna designs have leveraged these to achieve extraordinarily high gains, exceeding 79 dBi. This showcases the potential of metamaterials to significantly improve signal reception and widen the operational frequency range. Further, machine learning techniques are being incorporated to tackle the complexities of metamaterial antenna design, offering the potential to fine-tune their structures and performance. As demand for highly efficient, high-frequency antennas grows, exploring how to integrate metamaterials will be crucial for future advancements in wireless communication. It is important to acknowledge that there are still many challenges remaining for metamaterials to become widely utilized in various antenna design applications.
Metamaterials offer a promising avenue for enhancing antenna efficiency by leveraging their ability to manipulate electromagnetic waves in unconventional ways. Researchers have shown that incorporating metamaterial structures, like the Jerusalem cross, can yield substantial gains, as seen in a study by Bouzouad et al., where a 51 dBi improvement was achieved. This approach isn't limited to single-element antennas; integrating metamaterials into MIMO antenna arrays can lead to gains of up to 44 dBi alongside efficiency improvements, as demonstrated in 5G sub-6 GHz communication systems. Further evidence comes from the impressive peak gain of 79.4 dB achieved by a metamaterial-assisted superstrate antenna, highlighting the potential of these structures in optimizing antenna performance.
However, designing effective metamaterial-integrated antennas can be complex, with challenges related to dimensions and overall performance. Machine learning has emerged as a promising tool to address this, aiding in the design of structures with optimized properties. Another interesting technique involves incorporating high-impedance structures like EBGs. These structures have been shown to reduce surface waves, a factor that can limit antenna efficiency, thereby boosting gain and operational bandwidth. It's important to note that the integration of metamaterials isn't just about boosting gain; it also allows for the manipulation of radiation patterns. Using metamaterial superstrates can create a cavity effect, leading to enhanced gain and efficiency. While the field is relatively new, researchers are making headway, with recent studies offering a comprehensive look at the evolving role of metamaterials in antenna engineering, from basic principles to practical applications in modern wireless communication systems and beyond.
Optimizing Antenna Gain 7 Practical Techniques for Enhanced Signal Reception - Optimizing Radiation Patterns for Improved Signal Coverage
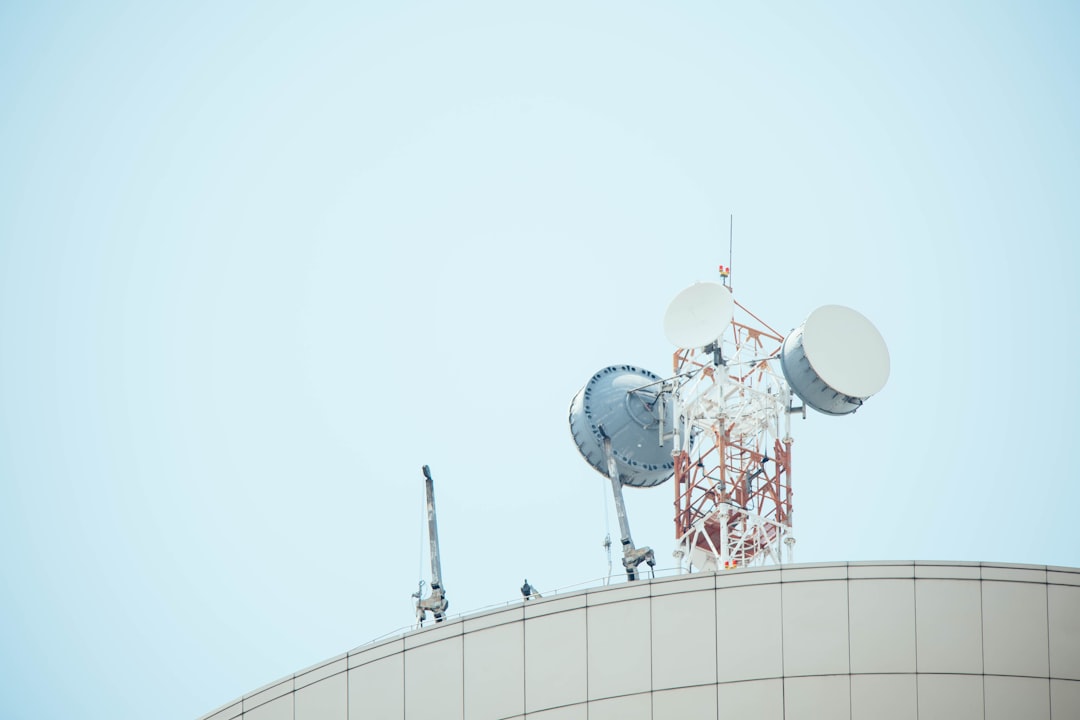
Optimizing the way an antenna emits radio waves, its radiation pattern, is crucial for maximizing signal coverage. This is especially important in scenarios where the signal needs to reach specific areas or avoid interfering with other signals. By carefully arranging and controlling the signals fed to the antenna elements in an array, we can shape the radiation pattern to reduce unwanted sidelobes and increase the main signal's strength, often referred to as gain. This ability to tailor the radiation pattern becomes even more significant in specialized applications like satellite communication, where precisely directed signals are essential for efficient operation.
The advent of sophisticated design techniques, such as those incorporating machine learning, has revolutionized the ability to synthesize and adjust radiation patterns. These algorithms allow for highly accurate and adaptable control over the way an antenna radiates, leading to improved performance. Further, the capability to tailor the radiation pattern to specific operational requirements opens the door for antennas optimized for a wide range of applications. The ability to customize the radiation pattern based on the application, not only maximizes signal strength but also improves the overall efficiency of the wireless system. While this presents advancements, it's important to keep in mind the limitations of any optimization technique as it relates to the application.
Antenna optimization techniques often focus on improving aspects like gain, efficiency, and bandwidth, but also on tailoring their radiation patterns. By manipulating the way an antenna emits and receives radio waves, we can significantly enhance the overall performance and reach of wireless communication systems.
Antenna arrays, by carefully arranging and controlling individual antenna elements, can offer superior performance compared to single antennas. They can achieve greater gain, minimize unwanted signal leakage (sidelobes), and even generate multiple focused beams. Optimizing the layout and the signals driving these elements is crucial to realizing these advantages.
While traditional design methods have been relied on for decades, newer computational tools and approaches are changing the landscape of antenna design. Machine learning, specifically, is increasingly being utilized to explore and refine antenna characteristics. This approach offers the potential to accelerate the design process and optimize performance in ways previously thought impossible.
Surrogate models—simplified representations of antenna performance—are becoming valuable in optimization processes due to their speed. These models allow engineers to quickly evaluate various design options without the computational burden of more detailed simulations, accelerating the optimization process.
Metamaterials, engineered materials with unique electromagnetic properties, have shown significant promise in enhancing antenna performance. Structures like split-ring resonators can radically alter the antenna's radiation pattern and significantly boost gain. It's worth noting though that achieving consistent performance and manufacturability in these designs remains a challenge.
Dual-polarized antennas—those capable of transmitting and receiving both horizontal and vertical polarization—have demonstrated noteworthy performance improvements, with some achieving impressive gain figures. This highlights the potential benefits of antenna designs that incorporate advanced polarization principles.
Multilayer perceptron networks, a type of artificial neural network, are being explored as a tool for optimizing the radiation patterns of antenna arrays. These networks can learn intricate relationships between antenna parameters and their performance, providing another pathway toward automated optimization.
Optimizing radiation patterns has direct impacts on practical applications like satellite communication systems. For example, by focusing the antenna's radiation in a desired direction, unwanted signal loss and interference can be reduced, ultimately improving performance and reliability.
Antenna dimensions play a crucial role in overall performance. Careful adjustments to length, width, and other parameters can fine-tune characteristics like gain and bandwidth. Understanding this interplay between size and performance is important for designing antennas that meet specific requirements.
The ability to precisely control the radiation pattern of an antenna array provides a powerful tool for engineers. This level of control lets them design antennas that meet specific operational needs. For instance, they might want a wide coverage area in one application, while a very narrow beam might be preferred in another.
Ultimately, the pursuit of optimizing antenna performance is a dynamic field. Each advancement, whether it's through clever configurations, advanced materials, or novel computational methods, pushes us closer to antennas that efficiently transmit and receive signals in complex and challenging environments.
Optimizing Antenna Gain 7 Practical Techniques for Enhanced Signal Reception - Applying Numerical Techniques in Antenna Design Processes
Applying numerical methods within antenna design has become crucial for achieving desired antenna performance in today's demanding applications. These techniques are valuable for optimizing various aspects of antenna design, including gain, bandwidth, and radiation patterns, while also dealing with numerous limitations that often arise. The introduction of machine learning methods has further amplified the potential of numerical techniques, allowing for a more systematic exploration and automation of the design process. Machine learning excels at identifying trends within large datasets generated by numerical field simulations. Moreover, innovative methods like nature-inspired algorithms are showing potential in addressing the rising need for antennas that meet increasingly complex performance criteria. Despite the promising advancements, challenges still exist, especially when grappling with the multifaceted nature of contemporary antenna design. The inherent complexities of these designs can sometimes hinder the straightforward application of these advanced techniques.
Applying numerical techniques has revolutionized antenna design, allowing for the analysis of complex structures and materials that were previously challenging to investigate with conventional methods. Techniques like the finite element method (FEM) and the method of moments (MoM) provide detailed simulations of antenna behavior under real-world conditions, leading to better performance predictions before physical prototypes are created.
Computational electromagnetic (CEM) simulations can significantly shorten the antenna design process. Engineers can explore a wide range of designs much faster than with traditional experimental methods. This accelerated design process enables quicker adoption of new technologies and meeting stringent performance standards.
These numerical models often reveal unexpected characteristics, such as how small variations in the antenna's surface can significantly impact gain and radiation patterns. Understanding these subtleties is crucial for crafting high-performance antennas for advanced communication systems.
Furthermore, antenna designers can simulate various environmental factors, including urban interference and atmospheric effects, ensuring antennas perform consistently across different operating conditions. This ability is particularly vital for applications like cellular and satellite communication where external influences can dramatically alter signal quality.
The integration of machine learning and numerical modeling has spurred the development of adaptive algorithms. These algorithms learn from simulation data, refining designs by predicting how alterations impact antenna performance. This dynamic interplay enhances not just accuracy but also fuels innovation in the antenna design field.
Interestingly, applying these numerical techniques can help pinpoint optimal design parameters that maximize gain while minimizing losses due to interactions with nearby structures, like reflectors or other antennas. This optimization is particularly useful for antenna arrays in densely populated areas.
Researchers have also found that these numerical methods can be leveraged to improve the performance of unusual antenna designs like fractal antennas. These antennas utilize self-similar patterns to achieve multi-band functionality. Numerical models help predict the impact of complex shapes on performance, leading to compact designs with better signal reception.
However, the computational cost of detailed, full-wave simulations can limit the scope of designs. Fortunately, advancements in surrogate modeling provide a solution. These methods create simplified approximations of antenna behavior, allowing engineers to explore a wider design space without the computational burden of full-wave simulations.
Numerical simulations are also valuable in optimizing the placement and orientation of antennas within arrays, potentially diminishing the importance of empirical methods. This level of precision can lead to more efficient beamforming strategies in advanced communication applications.
Finally, the incorporation of software tools that blend numerical simulations and CAD design platforms offers a seamless transition from conceptual designs to practical applications. This streamlined workflow facilitates collaboration across engineering disciplines, minimizing miscommunication and costly redesigns that often arise during the development process.
While there are still challenges, these advancements demonstrate the power of computational methods in antenna engineering, contributing significantly to research and practical implementations across diverse fields.
Optimizing Antenna Gain 7 Practical Techniques for Enhanced Signal Reception - Leveraging Machine Learning Algorithms for Gain Optimization
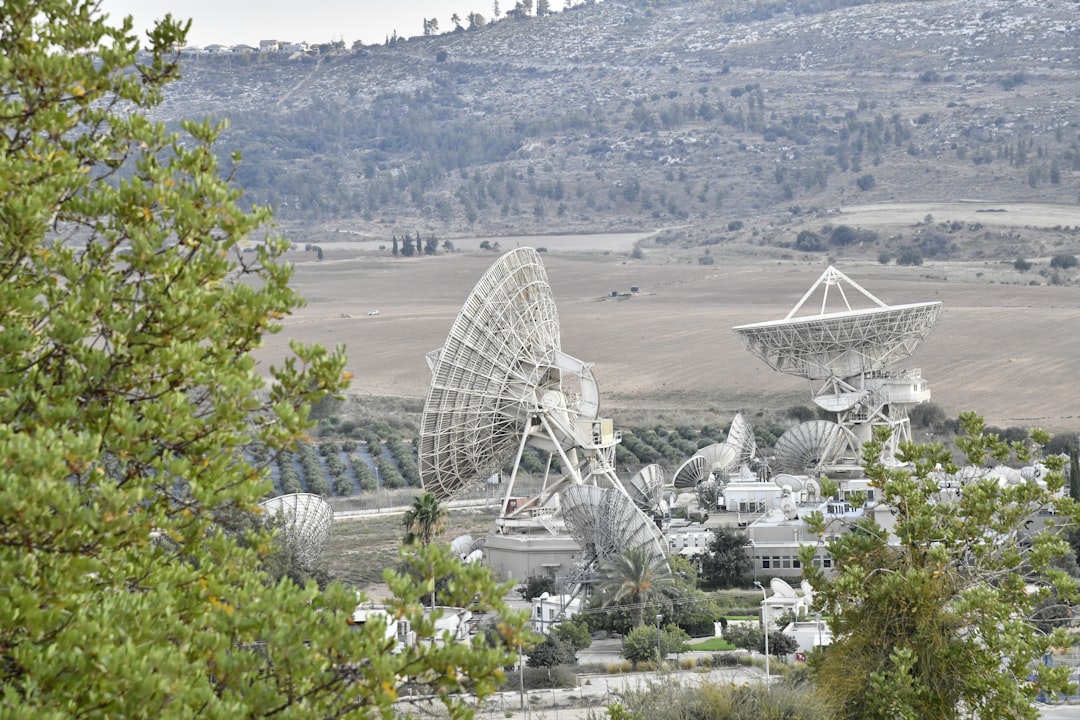
The use of machine learning algorithms for optimizing antenna gain represents a notable step forward in handling the intricate aspects of modern wireless systems. Techniques like deep learning and reinforcement learning augment conventional antenna design methods by enabling automated optimization, potentially leading to significant enhancements in gain, efficiency, and overall antenna performance. Various optimization strategies, including particle swarm optimization and genetic algorithms, have been integrated with machine learning to accelerate the antenna design process and improve the selection of operational parameters. Moreover, the combined power of machine learning and electromagnetic simulation has proven useful in evaluating antenna designs, highlighting the growing importance of incorporating these advanced methods to achieve desired results in the face of increasingly complex technological demands. While substantial progress has been made, challenges remain in fully realizing the potential of these techniques, especially in ensuring consistent and reliable performance across a wide range of antenna applications.
Machine learning algorithms have the potential to analyze large amounts of data from antenna simulations, revealing trends and relationships that conventional methods might miss, which can improve our understanding of antenna optimization. While the combination of machine learning and metamaterials has shown promise in enhancing antenna performance, the reported 99% efficiency improvements in some studies indicate the potential for data-driven design processes, but it's important to treat such claims with a degree of skepticism, as results in research often have a specific set of conditions they are valid for. Using machine learning adaptively, antenna designs could be made to adjust to feedback during operation, giving engineers a way to automatically fine-tune antenna parameters for the best gain. This could be a significant advancement over using static designs.
The integration of machine learning and numerical techniques allows for a combined approach to antenna design, enabling a more thorough assessment of various performance aspects, including gain, radiation patterns, and bandwidth. Reinforcement learning could be applied to manage the distribution of signals in antenna arrays, improving gain in environments with changing interference. Intriguingly, machine learning could potentially help mitigate the negative effects of external factors on antenna performance by creating designs that are more robust to issues like urban obstructions or multipath propagation. Generative design algorithms, powered by machine learning, offer a way to explore unique antenna shapes and structures that traditional design methods might not consider. This could lead to innovative designs pushing the limits of gain optimization.
Machine learning can now predict the impact of physical changes on antenna gain before an antenna is built, speeding up the prototyping phase and reducing costs. It's important to note that this is still an area of research and the practical implementation needs to be verified. The pairing of machine learning with FDTD simulations can provide a better way to model how antennas react to quick changes in signals, a key aspect for applications like 5G networks that require rapid adjustments. Multi-objective optimization algorithms combined with antenna design can refine gain while also considering other factors, like antenna size and bandwidth, which would allow antennas to be used in a greater variety of communication applications. However, it's crucial to acknowledge that the full potential of these methods hasn't been entirely realized yet and that much of this is still an ongoing research effort.
Optimizing Antenna Gain 7 Practical Techniques for Enhanced Signal Reception - Implementing Precise Gain Measurement Methods to Reduce Errors
Achieving accurate antenna gain measurements is crucial for ensuring optimal antenna performance and reducing errors in antenna design and testing. Different measurement methods exist, each with its own limitations and potential sources of error, especially when dealing with significant differences in size between the antenna being tested and the reference standard. For example, methods like the modified gain-transfer technique help reduce measurement errors that can occur when the antenna being characterized is substantially larger than the standard gain horn. This is important because size discrepancies can lead to inaccuracies in the final gain measurements.
The reinstatement of services like the NIST Antenna Gain and Polarization Calibration program emphasizes the need for standardized calibration methods to ensure consistent and accurate gain values across different testing environments. This ensures long-term comparability of measurements. In addition, recent developments in measurement techniques, particularly in near-field scenarios where antenna spacing is limited, are providing ways to refine measurement accuracy, leading to better understanding of antenna behavior. This is particularly valuable for the increasing number of antennas operating in challenging environments. Given the increasing importance of antenna performance in a wide range of applications, refining measurement accuracy will remain a priority as we move forward.
Gain measurements are foundational for antenna development and evaluation. However, the accuracy of these measurements can be significantly impacted by the surrounding environment and the tools used. Different measurement techniques have varying levels of inherent accuracy, and it's essential to understand those limitations. When there's a substantial size difference between the antenna under test and the reference antenna (often a standard gain horn), errors in the gain measurements can be quite large.
One method that's been shown to reduce measurement errors, particularly for antennas much larger than the reference, is the modified gain-transfer method. It essentially offers a more accurate way to relate the unknown antenna gain to a known reference. The National Institute of Standards and Technology (NIST) has reintroduced its antenna gain and polarization calibration service, establishing a connection with multiple national metrology organizations. This brings a sense of continuity to antenna gain values, providing a consistent benchmark over time.
Researchers have explored different measurement approaches. The three-antenna gain method, for instance, has achieved promising accuracy (0.23 dB) in determining gain. While this is impressive, direct gain measurements using a NIST pre-calibrated probe have achieved even better accuracy (0.16 dB). Another benefit of the three-antenna method is that it can calculate gain more precisely, addressing potential limitations in gain tables, especially at lower frequencies.
A common tool in gain calculation is the Friis equation. However, it relies on the assumption that we're in the far-field region. When antennas are closer together than the far-field distance, determining the precise antenna amplitude centers becomes tricky, which can introduce error. Recently, there's been progress in developing methods for more accurate gain measurements, particularly in scenarios with limited separation between antennas.
The setup itself can introduce errors. Slight deviations or reflections can impact the measured data. To address these concerns, there has been ongoing research on techniques to improve measurement accuracy both in the far-field and near-field zones. Moreover, for antennas that don't meet the requirements of the far-field region, alternative methods that bypass traditional near-field to far-field transformations have been investigated to improve accuracy. There's still active research in this space, as it's essential for ensuring antenna gain measurements are reliable across various antenna types and configurations.
Optimizing Antenna Gain 7 Practical Techniques for Enhanced Signal Reception - Fine-tuning Impedance Matching for Maximum Power Transfer
Fine-tuning impedance matching plays a crucial role in maximizing the power transferred from an antenna to its connected circuit. When the antenna's impedance aligns with the impedance of the load (the device or circuit it's connected to), power transfer is optimized. This is fundamental to achieving strong signal reception, as any mismatch results in power loss. Modern antenna designs often employ automated tuning methods, particularly adaptive algorithms, which can dynamically adjust the impedance matching network. This is essential as antenna input impedance can change based on the environment or the operating frequency. The ability to adapt in real-time ensures the system maintains optimal power transfer even under changing conditions. Beyond simply minimizing power loss, these networks also enhance overall system performance and are therefore vital components in well-designed radio frequency systems. Researchers are continually exploring more sophisticated approaches to impedance matching, such as those using quantum computing techniques. These advanced methods promise further improvements in transmission efficiency and the ability to filter out unwanted noise, showcasing that fine-tuning impedance matching remains an active area of research and development.
1. **Impedance Matching for Power Transfer:** Matching the antenna's impedance to the connected circuit (the load) is key to maximizing the power delivered to the intended receiver. If these impedances aren't matched, a lot of the signal can be reflected back, significantly impacting the antenna's overall performance. Getting that balance right leads to the best power transfer efficiency, which is important for strong signal reception.
2. **Quantifying Mismatch:** The reflection coefficient (Γ) offers a handy way to measure impedance mismatch. A low Γ value (e.g., -10 dB) means a good match, with little power being reflected. On the other hand, a high Γ (e.g., 0 dB) indicates that essentially all of the power is bounced back, representing a poor match and severely limiting power transfer.
3. **Real and Imaginary Impedance Components:** It's important to remember that an antenna's impedance isn't just a single value. It has both resistive (real) and reactive (imaginary) components. To optimize antenna performance, these need to be carefully tuned using matching networks. Those reactive components can introduce losses if not managed properly.
4. **Frequency's Influence on Impedance:** Antennas aren't always perfectly matched across the entire range of frequencies they're intended to operate on. The antenna's impedance can change significantly with frequency, leading to less than optimal performance at some frequencies. The use of matching networks lets engineers adjust the match across a desired range, vital for multi-band and wideband antennas.
5. **Radiation Pattern Impact:** Impedance matching isn't just about power transfer; it can influence the way an antenna radiates its signal (its radiation pattern). A poor match can create unwanted side lobes and reduce the main signal's strength, which affects how efficiently the signal is directed and received.
6. **Visualizing Impedance:** The Smith chart is a very useful tool for working with impedance and matching. Engineers use it to visualize the complex impedance, design matching networks, and fine-tune components to achieve the ideal match for the intended operating conditions, leading to optimal power transfer.
7. **Designing Matching Networks:** Depending on the specific needs of the antenna, different types of matching networks can be used. L-networks, T-networks, and Pi-networks are some common choices. The choice depends on factors like the desired impedance, size constraints, and overall complexity. There are trade-offs associated with each type that engineers have to consider.
8. **Adaptive Impedance Matching:** In some antenna designs, particularly more advanced ones, we see adaptive impedance matching. This approach lets the matching network dynamically adjust its characteristics depending on the current operating conditions. This adaptability is really important in situations where the antenna's environment is changing, which enhances signal strength and efficiency.
9. **Importance for MIMO Systems:** MIMO (multiple-input multiple-output) systems use multiple antennas to improve data rates and reliability. Each antenna element in a MIMO system needs to have good impedance matching. If they don't, the performance of the entire MIMO system can degrade.
10. **Measurement Challenges:** Measuring impedance accurately is an important part of evaluating antenna performance, but it can also be a challenge. You need good equipment and techniques to make sure the measurement is accurate. Measurement errors can result in inaccurate assessments of the antenna performance, so proper calibration is a crucial step.
Optimizing Antenna Gain 7 Practical Techniques for Enhanced Signal Reception - Exploring Advanced Materials for Enhanced Antenna Performance
The use of advanced materials, especially metamaterials, is revolutionizing antenna design and leading to significant performance enhancements. Recent research highlights the potential of novel structures like Dual Layer Composite Radio Metamaterials (DLCR), which have demonstrated a substantial 204% increase in antenna bandwidth alongside a notable 4.69 dBi improvement in broadside gain compared to traditional antenna designs. Metamaterials, with their ability to manipulate electromagnetic waves in unusual ways, are enabling substantial improvements in antenna characteristics like gain and bandwidth. For example, Metamaterial Near-zero (MNZ) unit cells integrated with a bowtie antenna have achieved significant gain enhancements across a specific frequency range.
However, despite these promising results, there are still challenges associated with designing and implementing metamaterial-integrated antennas. Achieving optimal performance and ensuring manufacturability remains a hurdle. While the integration of advanced materials into antenna systems offers a path towards superior performance, this field is still developing and dealing with real-world challenges in implementing these new designs.
The exploration of novel materials is pushing the boundaries of antenna performance. Superconducting materials, for instance, could lead to dramatically improved efficiency at extremely low temperatures, largely due to their lack of resistance. This could have significant implications for high-frequency applications, potentially revolutionizing signal integrity. Frequency-selective surfaces (FSS) offer a way to enhance gain by selectively filtering specific frequencies. This selective filtering can optimize signal reception without the need for increased power.
Active materials that can change their properties dynamically are another fascinating development. Materials like piezoelectrics and electrostrictives could enable antennas to adapt to varying conditions in real time, improving their performance and gain in environments that change frequently. Metasurfaces, which can manipulate electromagnetic waves in two dimensions, have shown incredible potential for achieving high-efficiency and highly directional antennas in very compact form factors. These often surpass the capabilities of conventional designs in terms of gain and efficiency for their size.
The combination of traditional and advanced materials, like the use of carbon nanotubes within composite structures, is showing promise in improving antenna strength while reducing their weight. This makes the antennas more robust without sacrificing performance or requiring excessive material use. Topological insulators, a relatively recent area of research, are intriguing as they conduct electricity on their surface while remaining insulating within their structure. This unique property could open doors to novel designs that might enhance gain in unexpected ways.
Magnetic materials offer the possibility of tuning the antenna gain by modifying the surrounding magnetic field. This adaptability could be extremely useful for specific scenarios where antenna performance needs to be dynamically adjusted. Nano-coatings can increase the conductivity of antenna surfaces, minimizing losses due to the skin effect that occurs at high frequencies. This is particularly important for millimeter-wave communication systems.
Silk-based antennas offer an interesting twist. Experiments with silk protein suggest that it's possible to produce flexible and efficient antennas, potentially changing how we view antenna construction and expanding potential applications in areas like wearable technology. The potential applications of graphene in antennas are also promising. Integrating graphene into antenna designs may lead to significant improvements in bandwidth and energy efficiency. These are attractive improvements in antenna design that could be especially useful in applications that rely on low-power communications.
While these materials offer significant opportunities, challenges remain. Further research and refinement are necessary to ensure their wide adoption in a range of antenna applications. The overall impact on practical applications is yet to be fully realized, and the effectiveness of some of these concepts might only be apparent as the field of antenna design continues to evolve in the coming years.
Revolutionize structural engineering with AI-powered analysis and design. Transform blueprints into intelligent solutions in minutes. (Get started for free)
More Posts from aistructuralreview.com: