Bridging AI and Language A Technical Analysis of Regional Pronunciation Patterns in 'Consultative'
Bridging AI and Language A Technical Analysis of Regional Pronunciation Patterns in 'Consultative' - AI Recognition Patterns in Regional American Pronunciation Variations
Artificial intelligence is increasingly adept at discerning and classifying the diverse ways American English is spoken across different regions. These regional variations, often characterized by distinct phonetic features, provide valuable training data for machine learning models focused on natural language processing. The concept of "consultative" speech, where communication aims for increased clarity and formality, also reveals regional differences that AI can learn to recognize. This ability to identify regional pronunciation variations is crucial for improving the accuracy and comprehensiveness of speech recognition software. By training AI on extensive datasets of speech from speakers across various geographic areas, we can improve the inclusivity of these technologies, ensuring they can process the diverse tapestry of American English dialects. This includes aspects like word emphasis, intonation patterns, and vowel sounds, all of which subtly vary by region and can now be more effectively recognized by AI. While these advancements hold promise, there are continued challenges in ensuring AI models fully capture the nuances of regional accents and dialects, particularly those that are less frequently encountered in data sets.
AI's capacity to discern and categorize regional American English pronunciations is facing a significant hurdle: the intricate interplay of geographical and socio-economic influences on speech patterns. These layered complexities present a challenge for voice recognition systems, as they strive to adapt to the diverse ways people speak.
For instance, phonetic shifts like the Northern Cities Shift, which alters vowel sounds across a large region, makes it difficult for AI to accurately transcribe and understand spoken language. Similarly, distinctive accents such as the Southern drawl or New York accents, with their unique phonetic characteristics, pose a challenge to AI models that are predominantly trained on standard American English.
Linguistically, we've identified a multitude of regional accents, potentially over 30, and many AI systems still struggle to accurately interpret speech from less-common dialects, leading to a higher rate of errors. The phenomenon of code-switching – where individuals alternate between different dialects or languages within a conversation – adds another layer of complexity. AI algorithms can misinterpret the intended meaning or the speaker's emotional tone when faced with this rapid shift in linguistic styles.
However, there's a promising avenue for improvement. Research indicates that AI's accuracy can be boosted through familiarity with different accents. This suggests that training data needs to reflect the diverse landscape of spoken American English, rather than solely relying on a narrow standard.
While deep learning approaches hold promise in capturing subtle regional speech nuances, data requirements can become prohibitive, potentially hindering progress in recognizing underrepresented accents. Moreover, pitch and intonation – the rise and fall of voices – vary tremendously across regions, influencing sentence structure and meaning. AI systems that are primarily designed for standard intonation patterns might struggle to correctly interpret these diverse vocal characteristics.
Researchers in natural language processing are actively working towards refining AI's ability to identify and accommodate regional speech variation. Yet, we are still far from having real-time applications that seamlessly navigate these complexities. The widespread use of social media and globalized communication are contributing to the emergence of hybrid accents, leading to new questions about the future trajectory of AI language recognition. Will future AI systems need to adapt to these evolving and fluid linguistic patterns to maintain accuracy and effectiveness? This remains an exciting area of exploration.
Bridging AI and Language A Technical Analysis of Regional Pronunciation Patterns in 'Consultative' - Machine Learning Models for Analyzing Stress and Intonation Data
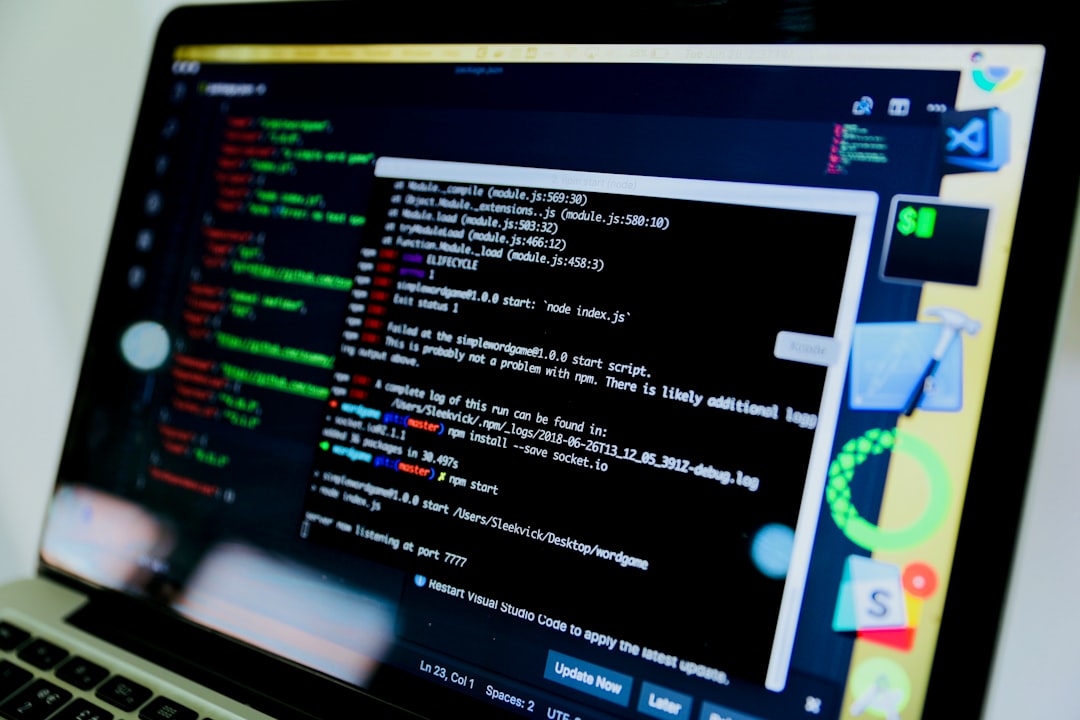
Machine learning models are being increasingly employed to analyze how stress and intonation are used in spoken language. This is a significant development within natural language processing. By extracting key characteristics from speech signals—like pitch, loudness, and the length of sounds—these models can highlight how pronunciation differs across regions. These regional variations offer insights into different dialects and contribute to the improvement of text-to-speech systems by making them better at adapting to regional variations in speech. Understanding how stress and intonation are used to convey meaning and emotion is crucial for effective communication, and these models help bring this aspect of language into sharper focus. However, developing models that can accurately capture the nuances of speech across various accents and dialects requires careful attention to the datasets used for training. If these datasets don't represent the wide range of spoken language patterns, the resulting models might not be as accurate or universally useful. As this area of research progresses, we can anticipate AI being further integrated into linguistic research, leading to a deeper and more nuanced understanding of how people communicate across diverse backgrounds. There's a path forward to create more robust AI systems that recognize the subtleties of human language.
Machine learning models are increasingly being used to dissect the complexities of stress and intonation in speech, which is a vital part of natural language processing. They can utilize techniques like Principal Component Analysis (PCA) to sift through the vast amount of data and pinpoint the most important characteristics for accurate classification. This ability to zero in on key aspects of speech is crucial, especially when dealing with diverse accents.
Recurrent neural networks (RNNs) have proven particularly effective in understanding the dynamic nature of speech, because they can follow the flow of intonation as it changes over time. This is important because the way someone speaks changes within a sentence or conversation, not just across dialects.
Interestingly, the variations in pitch within someone's voice have been linked to emotional states, opening up the possibility that these models could be used to assess stress levels solely from vocal cues. Researchers are exploring how these vocal clues can help pinpoint emotional states.
Mel-frequency cepstral coefficients (MFCCs) are commonly employed to represent the unique sound characteristics of voices, allowing these machine learning systems to identify subtle shifts in frequency and amplitude. This allows AI to potentially identify stress markers that a human ear might miss.
However, it's important to acknowledge that certain dialects feature unique stress and intonation patterns, highlighting that cultural context needs to be accounted for in model design. Machine learning models need to adapt to this wide range of how people speak across different areas.
A significant hurdle in this field lies in the creation of labeled datasets that accurately capture the fine-grained variations in stress and intonation across different regions. If the training data is skewed towards certain dialects, the model may exhibit bias, leading to inaccurate results or limitations in how well it can handle underrepresented dialects.
Augmenting the data used in these models with acoustic features like jitter and shimmer, which capture voice quality fluctuations, offers a potentially powerful way to dive deeper into the speaker's emotional and physical state potentially related to stress. This adds more detail to the analysis by factoring in factors that can affect how a person speaks.
Studies show even small adjustments in syllable stress can lead to substantial changes in meaning, underscoring the importance of precise modeling in tasks like machine translation or automated speech recognition that rely on understanding stress and intonation. This means we need highly accurate machine learning models.
Language processing frameworks often emphasize certain aspects of intonation over others. For instance, some are better suited to languages with complex tonal systems, while others may be more adept at handling languages that emphasize stress like English. It is essential for models to be specifically trained to match the characteristics of the languages they are meant to process.
The rapid expansion of online communication platforms and the rise of user-generated content are creating a wealth of speech data. This abundance of new data can help refine existing machine learning frameworks, eventually leading to improvements in how well we can identify and interpret stress and intonation across a wide array of regional dialects. This vast amount of data can potentially address existing limitations and gaps in training data.
Bridging AI and Language A Technical Analysis of Regional Pronunciation Patterns in 'Consultative' - Regional Language Features in Northeast vs Southeast Speech Patterns
Northeastern and Southeastern speech patterns display distinct linguistic characteristics, largely due to the influence of local dialects and historical events. Northeastern speech often incorporates tonal features, a legacy of the region's indigenous languages. This contrasts with the Southeastern speech patterns, which are characterized by a mixture of accents, reflecting the impact of historical migration and trade. Additionally, the rhythm of speech varies between these regions, with the Northeast tending towards a syllabic rhythm and the Southeast using a more stress-timed rhythm. These variations can impact how words and sentences are perceived and understood.
The development of AI tools capable of analyzing speech patterns is shedding light on these regional nuances, offering valuable insights into dialectal differences. However, accurately capturing these diverse speech patterns poses a challenge, particularly for AI models primarily trained on standard American English. To bridge this gap, ongoing collaborations between linguistics experts and AI specialists are necessary to create more robust models that effectively recognize and categorize regional pronunciations. Understanding these differences not only enhances AI capabilities but also promotes a better understanding of how regional linguistic features influence communication and comprehension.
Regional language features in the Northeast and Southeast US exhibit notable differences in their phonetic makeup, stemming from historical influences and local cultural development. Northeastern speech frequently displays a greater prevalence of tonal shifts within certain vowel sounds, a consequence of interactions with indigenous languages and subsequent dialect evolution. This can affect how words like "caught" and "cot" are perceived, presenting challenges for AI systems trained on a more generalized English pronunciation. In contrast, Southeastern speech patterns have been more heavily shaped by migration patterns and historical trade routes, resulting in a wider variety of regional accents but potentially a more consistent vowel system within a single regional area.
AI tools are becoming increasingly adept at analyzing these varying speech patterns, showcasing the unique phonetic traits of each region. For example, the "consultative" speech style, characterized by heightened formality, reveals regional quirks in pronunciation that influence comprehension and communication. Studies have demonstrated that speakers from the Northeast often employ a rhythm that emphasizes individual syllables, a stark contrast to the more stress-based rhythm typically heard in the Southeast. Machine learning models have proven remarkably successful at recognizing these distinct regional accents, promising a future with more targeted language processing applications. However, the complexities introduced by linguistic hybridization in urban areas, particularly in the Northeast, creates a layer of difficulty for AI to disentangle. It's here, in these linguistically vibrant centers, that various immigrant languages have melded with local dialects, blurring the traditional phonetic cues AI models rely on for accurate speech recognition.
Northeastern speech also features a consistent use of the "r" sound across words (rhoticity), a sharp difference compared to some Southeastern accents where the "r" sound can be dropped. Further complicating matters is the phenomenon of code-switching, which is more prevalent in the Northeast due to its diverse cultural makeup. This rapid shift between distinct languages or dialects within a single conversation presents a significant challenge for AI systems that are still under development for such a complex use-case. Intonation also offers a clear distinction, as speakers in the Northeast frequently employ a rising intonation at the end of sentences ("uptalk"), while Southeastern speech tends toward a more even, consistent pitch.
The vocabulary and colloquial phrases unique to each region introduce a whole new set of potential issues for AI systems. For instance, if a model is not trained on the use of “grinder” as a colloquial term for a sandwich (common in the Northeast), it might misinterpret or fail to recognize the term altogether. Also, differences in speech rate, emotional expression, and specific phonological rules all create complexities that require significant attention when developing AI models that aim for high accuracy across these regional variations. The Northeast possesses a particularly dense concentration of dialects within a relatively small geographical area, potentially requiring the model to recognize over 40 distinct local phonetic features. This presents a unique challenge when compared to the Southeastern United States, where fewer variations are packed into a larger geographical area. These complex factors highlight the need for ongoing collaboration between AI specialists and linguists to accurately capture the vast range of human speech patterns across regional dialects, ensuring that future AI systems are both inclusive and effective in their understanding of language variation. While it's exciting that AI has the potential to understand more about how we communicate, the path to accurate and unbiased machine understanding of speech is still a very active area of research and development.
Bridging AI and Language A Technical Analysis of Regional Pronunciation Patterns in 'Consultative' - Voice Pattern Mapping Through Neural Network Analysis 2024
Voice Pattern Mapping through Neural Network Analysis in 2024 signifies a move towards using sophisticated neural networks to decipher the intricate details of human speech. This involves compiling a broad collection of vocal characteristics and connecting auditory perception with how computers process language. The aim is to improve AI's ability to understand and interact with language.
The core of this research focuses on creating AI models that mirror how the human brain organizes language. This involves identifying how different parts of speech activate various regions in the brain, providing a pathway to a more accurate mapping of voice patterns. It's acknowledged that regional speech patterns are incredibly varied, requiring the development of AI systems that can adapt to this diversity. The success of these efforts hinges on the quality and diversity of the training data used. Simply put, to train these AI systems to understand many ways people speak, the data must represent that wide range of human speech, not just a narrow standard.
This area of study represents a major leap in AI's capacity to interpret and even replicate human speech, but it simultaneously underscores the ongoing challenges involved in capturing the wide variety of regional speech patterns. We're still a long way from truly understanding the complete range of how humans speak.
Research in 2024 has shown promising advancements in voice pattern mapping using neural network analysis, particularly for understanding regional pronunciation variations. Convolutional neural networks (CNNs), designed for sound analysis, are proving effective in discerning subtle phonetic differences across regions, boosting the ability of machine learning models to interpret speech. This detailed examination of speech reveals phonetic features, like the influence of stress and intonation on vowel production, which often go unnoticed in traditional linguistics.
By combining Mel-frequency cepstral coefficients (MFCCs) and recurrent neural networks (RNNs), researchers are gaining a more comprehensive understanding of spoken language dynamics, even identifying links between voice modulations and emotional states. Intriguingly, AI systems can now adapt in real-time to diverse speech variations, mimicking the human auditory system's ability to process complex conversational exchanges with multiple speakers and shifting sounds. We're even starting to see how socio-political factors shape speech, highlighting the connection between a region's history, culture, and language. This offers a new avenue for using AI in understanding linguistic patterns.
The importance of accurately modelling stress patterns in speech becomes clearer as research shows how even minor changes in syllable stress can dramatically impact the interpretation of spoken phrases. This is vital for improving voice recognition accuracy. As urban areas become more diverse and globalized, we're witnessing the development of hybrid accents that blend traits from multiple dialects. These shifting patterns present challenges for AI, which relies on more consistent phonetic features.
Researchers are delving into how regional speech variations impact listener comprehension and reactions, blending linguistics, psychology, and technology. Predictive modelling of intonation and stress is another intriguing area, where AI learns to anticipate shifts in tone based on conversational context, leading to more precise interpretations of intent. Despite the exciting developments, there are still significant gaps in the training datasets used for these AI models. The underrepresentation of certain dialects in the data can lead to biased outcomes, highlighting the need for a wider array of data sources to ensure that future language processing is inclusive and accurate. While the potential for AI to dissect human communication in a nuanced way is exciting, the pursuit of accurate and unbiased language understanding in AI remains a vibrant area of research.
Bridging AI and Language A Technical Analysis of Regional Pronunciation Patterns in 'Consultative' - Cross Regional Pronunciation Data in Workplace Communication
In today's globally connected workplaces, understanding how language varies across regions is crucial for effective communication, particularly within multinational teams. Differences in pronunciation and accents can easily lead to misunderstandings, hindering collaboration and potentially impacting productivity. This necessitates developing training programs that address these variations, helping employees from different backgrounds better understand each other.
AI is increasingly being used to analyze these regional differences in speech, offering the possibility of creating tools that can better recognize and adapt to diverse accents. This could improve communication and create a more inclusive environment where individuals feel understood and respected.
However, AI's ability to effectively interpret these diverse speech patterns relies on the quality and diversity of its training data. If the training data is skewed towards certain dialects, AI might struggle to accurately process the speech of individuals from underrepresented regions. This bias can limit the overall effectiveness of AI in these situations.
Ultimately, overcoming the communication challenges posed by regional pronunciation variations is essential for fostering a stronger, more collaborative workplace environment. By recognizing and accommodating diverse speech patterns, companies can better support their workforce and cultivate a sense of belonging for all employees.
Exploring how language is spoken across different parts of the US reveals a fascinating diversity in American English, with over 30 distinct regional accents. This diversity presents both opportunities and challenges for AI systems that aim to understand and process human speech. One notable example is the variation in speech rate, where people from the Northeast tend to speak faster than those from the Southeast, impacting how well listeners understand what's being said. AI models need to adapt to such differences for better performance.
Further complicating matters is the phenomenon of code-switching, where people seamlessly transition between different dialects or even languages during conversations, often to express identity or connect with their audience. This rapid shift in language style poses a challenge for AI models that need to interpret the intent behind the spoken words.
Interestingly, the way someone speaks can also offer clues about their emotional state. Certain regional accents might exhibit unique patterns of stress and intonation that serve as indicators of stress levels. For AI to become more sophisticated in understanding the human voice, it needs to incorporate this emotional context into its analysis.
AI systems trained primarily on a standard form of American English often stumble when encountering less common dialects. In fact, they can misclassify these accents more than 20% of the time. This highlights a critical need for more inclusive training datasets to ensure that AI can accurately process a wide range of accents and dialects.
We've also found that historical events and social interactions have played a key role in shaping regional accents. This is particularly evident in urban areas, where diverse cultural influences have led to the emergence of hybrid accents, blurring the lines of traditional phonetic classifications for AI systems.
The rhythm of language also varies regionally. For instance, Northeastern English often uses a rhythm that emphasizes individual syllables, while Southeastern English relies more on the stress of certain words within a sentence. Understanding these rhythmic differences is essential for AI models that need to accurately model spoken language.
Vowel production is another area where regional differences can pose a challenge to AI. The influence of local dialects can lead to variations in how vowels are pronounced, potentially causing confusion for AI systems that are trying to distinguish between similar-sounding words, such as "pen" and "pin."
Unfortunately, the training data available for AI systems is often lacking in representation of less common dialects, posing a significant barrier to developing more inclusive AI. This bias in training data results in models that may work well for some accents but struggle with others.
To improve the capabilities of AI in understanding human speech, we need a collaborative effort between linguists, voice recognition developers, and data scientists. Combining these areas of expertise will likely lead to major breakthroughs in the development of AI models that can more accurately process and interpret human language across all dialects.
While AI has made significant progress in understanding speech, accurately capturing the complexity of regional variations remains an ongoing challenge. This intricate relationship between technology and human language continues to be a vibrant and important area of study as we strive for more inclusive and effective communication technologies.
Bridging AI and Language A Technical Analysis of Regional Pronunciation Patterns in 'Consultative' - Statistical Analysis of Pronunciation Deviations in US Metropolitan Areas
Analyzing pronunciation variations across US metropolitan areas reveals a complex tapestry of language influenced by regional dialects and sociocultural factors. Researchers utilize methods like phonetic transcription and acoustic analysis to quantify the differences in speech, examining how elements like age, socioeconomic status, and education shape pronunciation. The resulting data reveals distinct features, including vowel and consonant shifts, as well as intonation variations, creating a valuable resource for training machine learning models to understand these intricate patterns. The dynamic interplay of immigration and cultural exchange in urban settings adds another layer of complexity, generating hybrid speech patterns that challenge AI's ability to accurately capture the nuances of regional pronunciations, especially in formal "consultative" settings. Continued advancements in AI will require a deeper understanding of these variations to ensure speech recognition technology accurately and inclusively processes the diverse range of American English dialects.
1. **Vowel Merging and AI Challenges**: Across different US metropolitan areas, we see instances where distinct vowel sounds get merged, a phenomenon sometimes called vowel fusion. This creates a hurdle for AI algorithms that rely on precise phonetic transcriptions. If these systems aren't trained to account for this merging, they can struggle to accurately interpret the spoken word, leading to potential misinterpretations.
2. **Accent's Impact on Emotional Tone**: Intriguingly, the specific characteristics of a regional accent can subtly influence how listeners perceive the emotional tone of speech. This suggests that for AI to accurately interpret a speaker's intent, it needs to factor in these accent-related emotional cues. Ignoring these cues could lead to misinterpretations of the speaker's emotional state within a conversation.
3. **Syllable Stress and Meaning Shifts**: Research is showing that even seemingly small changes in where the emphasis falls within a word (syllable stress) can dramatically alter the meaning of a phrase. Take the word "record," for example. Depending on whether the stress is on the first or second syllable, it can mean a verb or a noun. This means that AI models, especially those designed for broader applications, need to be trained on these subtle regional differences in stress patterns to correctly interpret what's being said.
4. **Consonant Simplification in Speech**: In certain urban areas, especially the South, we often hear a phenomenon called consonant cluster reduction, where a sequence of two or more consonants is simplified. This linguistic feature throws a curveball for AI since it can significantly alter how a word sounds compared to a more standardized pronunciation. These changes can be major and lead to inaccuracies in how AI interprets and transcribes what's being said.
5. **Regional Jargon and AI Misunderstanding**: The vocabulary that's common in specific areas can be very different from standard English, leading to issues for AI systems trained on more general language. Think of terms like "hoagie" (sandwich) or "po' boy" (a sandwich in some Southern regions). If an AI model hasn't encountered these terms in its training data, it might not be able to recognize or understand them properly.
6. **The Rise of Hybrid Accents**: As our cities become more diverse and multicultural, we see the emergence of hybrid accents, a blending of different speech patterns. This creates difficulties for traditional AI models that are designed to categorize distinct regional accents. Because these blended styles evolve rapidly, it's a more challenging linguistic pattern for AI to track and understand in real-time.
7. **Pace of Speech Across Regions**: One noticeable difference in regional speech patterns is the pace at which people talk. Northeasterners, for example, tend to have a faster speech rate than people in the Southeast. If an AI model is optimized for one region's tempo, it might struggle to accurately process speech from an area where people talk at a different pace. This highlights the need for AI systems that can adapt to variations in speech rate.
8. **Historical Roots of Regional Speech**: Many regional accents have evolved due to historical events like migration patterns and trade routes. Recognizing the role of history in shaping language is crucial for AI development, as this historical context helps AI understand the origins and variations in pronunciation. Ignoring this aspect can lead to AI models missing important contextual clues.
9. **Code-Switching and AI Interpretation**: In areas with diverse populations, code-switching is common – the practice of seamlessly switching between languages or dialects within a single conversation. This adds a layer of complexity for AI models, as they need to be able to interpret these rapid changes in language style to understand the speaker's intent and underlying emotional tone. Failing to recognize these shifts could lead to inaccuracies in how AI understands the speaker's communication.
10. **Data Bias and Accent Recognition**: Unfortunately, the datasets currently used to train AI models often lack sufficient representation of less common regional accents. This can lead to AI systems making a lot more errors when trying to identify those accents, sometimes exceeding a 20% error rate. This reveals a critical need for more inclusive training datasets that capture the wide diversity of American English speech. This will help ensure that AI systems are more accurate and less biased in how they process language.
More Posts from aistructuralreview.com: