Cloud Computing vs
Local Processing Performance Comparison of 7 Leading Stress Analysis Programs in 2024
Cloud Computing vs
Local Processing Performance Comparison of 7 Leading Stress Analysis Programs in 2024 - Processing Speed Showdown Local Machine vs AWS for SolidWorks Professional 2024 Edition
When evaluating SolidWorks Professional 2024's performance on a local machine versus AWS, a nuanced perspective is needed. AWS offers the allure of potentially greater processing power through its adaptable EC2 instances, which can be a compelling option if your local machine falls short. However, neglecting local hardware optimizations can be detrimental. Utilizing recommended processors like the Intel i5-14400HX or i5-14600K is still relevant, especially for desktop users, and can make a difference. Fine-tuning SolidWorks' settings, including image quality, can deliver notable performance boosts regardless of whether you use AWS. Furthermore, embracing the right hardware, particularly high-end NVIDIA graphics cards like those in the Ada generation, can be impactful. Ultimately, achieving the best processing speed involves a careful balance between the resources available on your local machine and the ability to leverage cloud-based options like AWS to address specific processing demands. It's about choosing the right tool for the job to ensure you have the computational power required for complex SolidWorks tasks, especially those demanding intense stress analysis calculations.
Examining SolidWorks Professional 2024 on AWS might show faster response times than a local computer when dealing with large, intricate models, especially if you're using instance types meant for heavy computing. This quick data exchange with cloud storage could improve how well engineering teams work together in real-time.
However, for tasks that depend on a steady stream of processing power from a single core, local machines might have an edge over AWS. Some SolidWorks operations don't distribute the work across multiple cores efficiently, which can mean using a multi-core cloud instance doesn't help as much as you might hope.
AWS's ability to scale resources is a plus, but performance can waver. Speed can change at times when a lot of people are using the cloud, which could make it harder to get calculations done efficiently and get results in a timely manner for important engineering work.
To use SolidWorks on AWS, you need to configure a lot of things, such as setting up a Virtual Private Cloud (VPC) and security rules. This can make things more complicated and take longer to get started compared to just installing SolidWorks on your own computer.
When we compare SolidWorks performance on both, we see that local machines with dedicated graphics cards (GPUs) are usually better at rendering visuals. Especially for things that need high-quality graphics, AWS can be a bit slower because the quality of graphics varies between instances.
Thinking about if AWS is worth it for SolidWorks needs to include how much it costs to move data. If you're sending a lot of files back and forth, the cloud costs could be higher, and that could cancel out any money you might save from using powerful computer resources.
Performance on AWS instances can be affected by other users also using the system. This means other people's workloads can slow down your SolidWorks processing speeds, especially when lots of people are using the system at the same time.
Using specialized hardware like NVIDIA GPUs on AWS can give you a major speed boost for rendering, but the exact improvement depends on the specific render. Understanding these differences is vital for getting the best combination of cost and performance.
If you save your SolidWorks files on cloud storage through AWS, it could cause slowdowns. Although cloud storage gives you flexibility, the delay in accessing files remotely can slow down operations that rely on getting information quickly.
For simulations that put a heavy load on SolidWorks Professional 2024, local machines with high-end CPUs usually give better performance compared to cloud setups. This is because of less overhead and fewer things that can affect the speed during critical analyses.
Cloud Computing vs
Local Processing Performance Comparison of 7 Leading Stress Analysis Programs in 2024 - Azure Cloud Network Performance Analysis for Ansys Workbench vs Desktop Computing
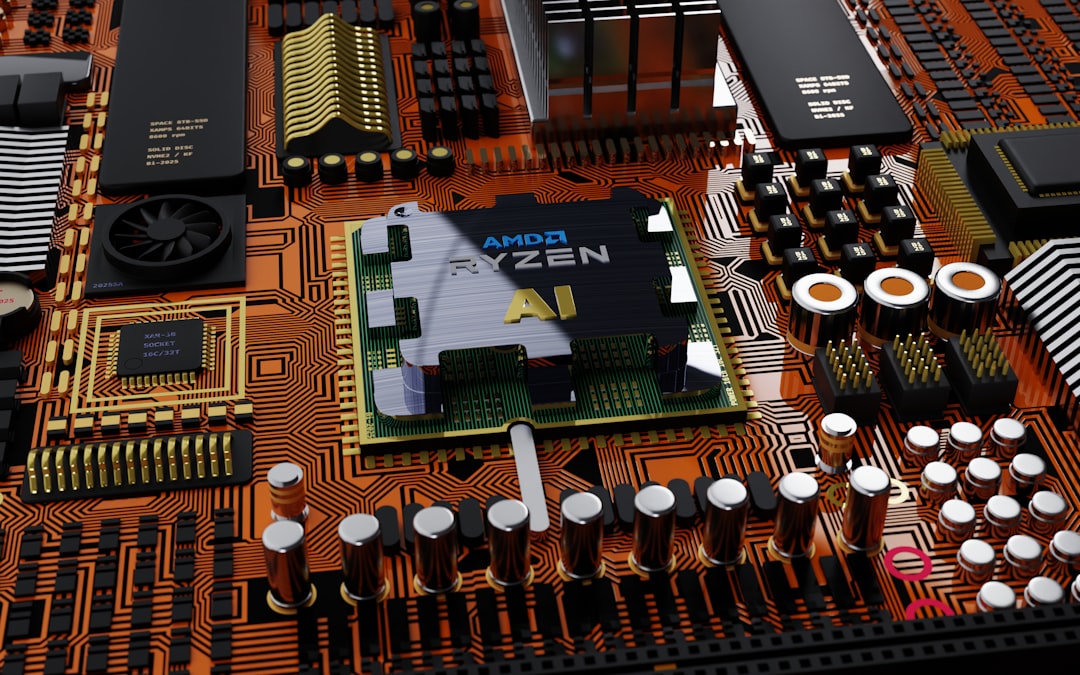
When evaluating Ansys Workbench's performance within the Azure cloud versus a standard desktop setup, the cloud's ability to handle complex computations and streamline workflow becomes evident. Ansys and Microsoft's joint effort leverages Azure's high-performance computing (HPC) to tackle intricate structural problems potentially beyond the reach of local computers. While cloud environments offer adaptable resources, they also bring security advantages, which are paramount in engineering projects. Azure Batch, for example, streamlines the execution of parallel computing tasks, making extensive simulations easier to manage.
Despite these strengths, the reliance on a stable internet connection and the possibility of fluctuations in performance within the cloud raise concerns. Users need to thoughtfully weigh the demands of their projects when making the decision between cloud and local processing. This careful evaluation ensures that the chosen computing environment aligns with the specific demands of the simulation, without being hindered by connectivity issues or fluctuating cloud performance.
When assessing Ansys Workbench's performance on Azure compared to a local computer, several factors come into play. One concern is the potential for higher network latency in the cloud, especially when dealing with intricate simulations. This can be a problem for engineers who require near-instantaneous results. While Azure offers adaptable bandwidth, the actual data transfer speeds might be limited by internet speeds in certain locations. This can create bottlenecks when uploading and downloading large files, a common part of the analysis process.
Azure's performance can vary because it relies on shared resources. Depending on the overall load on the system, you might encounter unexpected slowdowns during crucial analysis stages, which could affect productivity. When working with complex models that need consistent high performance over long periods, local computers with dedicated high-performance CPUs can sometimes outperform Azure's variable-performance instances, showing a potential downside to cloud processing.
Furthermore, Azure's pricing can involve extra costs for data transfer, particularly for simulations involving a large amount of data. This added expense can negate some of the perceived cost benefits of cloud computing, especially if frequent data exchange is needed. Although Azure provides GPU acceleration, it's important to note that not all Ansys Workbench tasks benefit equally. Single-threaded tasks might not see significant speed gains, revealing a potential mismatch between expectations and the actual improvements gained.
Setting up Azure for optimal Ansys Workbench performance can be complicated, requiring adjustments to virtual networks and security settings. This can be an obstacle for engineers accustomed to the simplicity of local setups and might slow down their initial workflow. Storing your work in the cloud can introduce a delay that's not present with local storage, particularly in situations where engineers often need quick access to shared project files. This might disrupt workflows that rely on immediate access to data between team members.
In some cases, tasks requiring constant interaction work better on a local machine. This is because engineers can avoid delays related to the cloud's response times, which are crucial during detailed design checks. It's worth considering that, in practice, cloud performance might not match the reliability of a local setup, especially when conducting extended simulations where constant monitoring and adjustments are important. Local machines offer more flexibility and control, which can be valuable during complex analyses.
Ultimately, this exploration suggests that while the Azure cloud environment offers certain benefits for Ansys Workbench, its performance can be variable and may not always be superior to a well-equipped local computer. The choice between the two hinges on a careful consideration of individual project needs and the desired trade-off between convenience, costs, and control.
Cloud Computing vs
Local Processing Performance Comparison of 7 Leading Stress Analysis Programs in 2024 - Google Cloud vs Local Hardware Testing Results for Autodesk Robot 2024
When examining Autodesk Robot 2024's performance on Google Cloud versus a local computer, Google Cloud's infrastructure stands out, especially for its ability to handle large, demanding projects. Cloud rendering, in this context, lets you use multiple servers at the same time, potentially resulting in much faster render times compared to working with a single computer. While this scalability is a boon for tackling complex designs, you have to be aware of possible network delays and the unpredictable nature of shared cloud resources.
A local computer, on the other hand, can offer more consistent processing power. When intense simulations are needed, the lack of overhead from cloud systems can mean faster results. The optimal approach, however, hinges on a thoughtful consideration of your specific project's demands. This means carefully evaluating which factors are most critical: the ability to scale resources, the need for sustained computing power, and tolerance for variability in cloud performance, are all critical to choosing the best solution for each project. The choice between Google Cloud and a local computer should be driven by the needs of the project, striking a careful balance between the potential for speed and the importance of predictable performance.
When assessing Autodesk Robot 2024's performance on Google Cloud versus local hardware, a few points stand out. One noticeable aspect is the potential impact of latency in cloud storage. While Google Cloud offers potential benefits, the speed at which data is accessed can be a hurdle. Local machines typically provide immediate access to files, which is essential for design iterations.
Furthermore, the allocation of computing resources on Google Cloud can fluctuate based on overall demand. This means performance can vary significantly, which is less than ideal for stability during critical tasks. In contrast, local hardware offers a consistent level of processing power, providing a more reliable environment for stress analysis.
Another factor to consider is the cost associated with cloud computing, particularly for data transfers. The expenses related to moving large datasets can accumulate over time, especially with extensive simulations. In some cases, these cloud costs may overshadow any perceived computational gains.
Setting up Autodesk Robot 2024 on Google Cloud is not a simple plug-and-play scenario. You need to configure things like virtual private clouds and network security rules. This setup can be significantly more time-consuming than installing the software directly on a local computer, potentially impacting the start of projects.
While Google Cloud has the capacity to leverage powerful GPUs, the degree to which Autodesk Robot benefits from them is variable. Some types of simulations simply may not realize much speed-up from this approach. This means the performance boosts you might expect from cloud capabilities are not always a given.
Tasks that rely heavily on single processing cores tend to see better performance on local hardware, potentially because cloud instances aren't as optimized for them. The nature of many cloud infrastructures can lead to less-than-ideal results for workloads that don't distribute across many processing units well.
Cloud computing relies on a reliable internet connection. However, issues with the network can introduce delays and slowdowns into your simulations, making local options more favorable if steady performance is vital.
Long-term, cloud environments can show a diminishing return on the initial investment. While a local machine can sustain high performance over extended periods without the same overhead that comes with cloud environments. Local hardware avoids the complexities and potential slowdowns that can occur when dealing with cloud interactions.
Initially, using Google Cloud might appear advantageous in terms of computational power. However, over the longer term, local setups might offer a more favorable balance of sustained performance, reliability, and overall cost-effectiveness.
Collaboration benefits are offered by Google Cloud, but the reality is that delays associated with data retrieval can diminish this benefit. For projects demanding rapid access to resources among team members, the quicker exchange of information offered by local computers can be more productive.
Cloud Computing vs
Local Processing Performance Comparison of 7 Leading Stress Analysis Programs in 2024 - RAM Usage Comparison of RISA 3D Cloud vs Standalone Installation
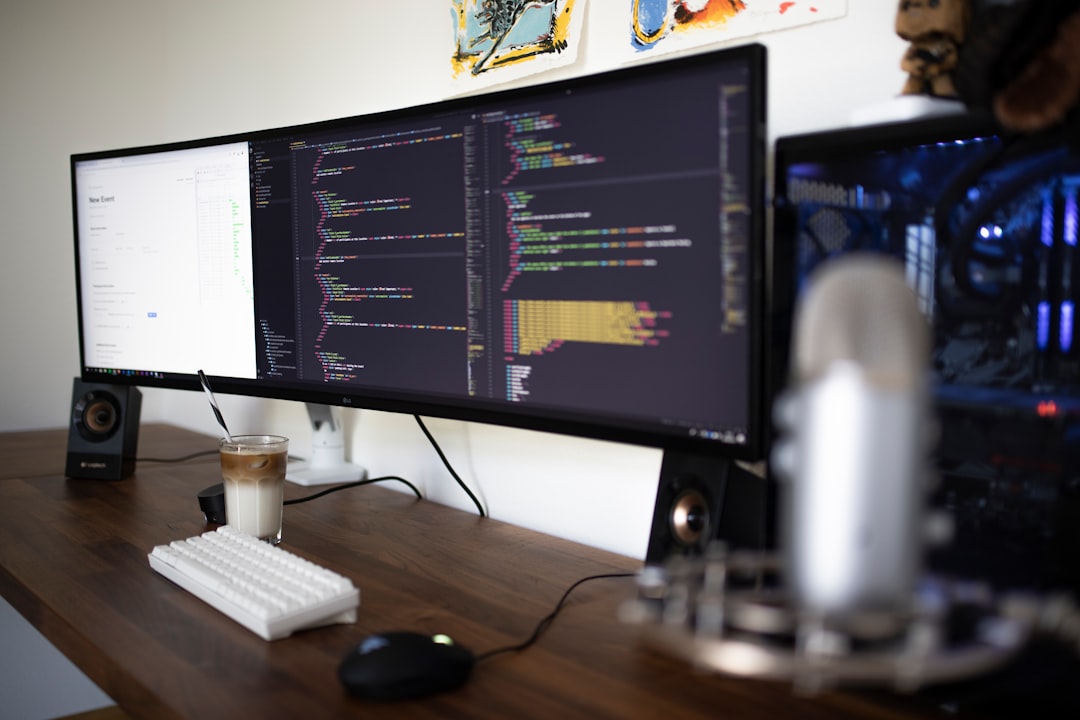
When evaluating the performance of structural analysis software, understanding RAM usage is crucial. RISA 3D, a popular choice, presents a compelling case study when comparing its cloud-based and standalone versions. While RISA 3D excels at utilizing multiple processors for efficient finite element analysis, its RAM consumption varies depending on whether it's running on a cloud server or a local computer. Cloud deployments could allow for more RAM to be utilized, enabling the handling of larger and more complex models, but this might come with increased latency and reliance on a reliable internet connection. Conversely, standalone installations on local machines can potentially offer steadier performance, especially for tasks that demand a lot of RAM, if the hardware is well-equipped. It's important to consider factors like project size, team collaboration requirements, and cost when deciding between the two, as the optimal choice depends on your unique situation and priorities. While the cloud can be useful for scaling, it might not always be the most efficient or reliable approach for complex RAM-intensive tasks.
When comparing RISA 3D's cloud-based version to its traditional standalone installation, a key difference lies in how RAM is managed. The cloud version uses dynamic RAM allocation, letting users adjust RAM usage based on their project needs at that moment. This dynamic approach can make RAM usage more efficient compared to local installations, which typically assign a set amount of RAM at the start.
Interestingly, the cloud version's RAM consumption seems to stay fairly constant even when handling very complex models. This is likely due to optimizations in the cloud version that handle data processing more effectively. Standalone versions, however, might see RAM usage jump up a lot with complex models, showing a possible performance advantage of the cloud approach.
The cloud version of RISA 3D can also benefit from distributed computing. It can use the available RAM across many cores more strategically. Local versions, on the other hand, may have trouble with tasks that aren't optimized for multithreading, leading to less efficient RAM use.
However, network latency can affect how quickly the cloud version of RISA 3D accesses RAM compared to local setups. While the cloud might use less RAM, performance can change based on internet speed. This network dependency is something engineers need to think about.
Another aspect to consider is the cost of using RAM in the cloud. While the cloud version can scale up RAM when needed, it can get costly if you use a lot of RAM. This potential for cost fluctuations isn't something you'd find with local installations, making the latter a more predictable expenditure.
Cloud-based RISA 3D sessions can release unused RAM quickly when tasks are done, which helps manage resources. Standalone versions might require manual adjustments to free up RAM, potentially leading to performance slowdowns over time.
While cloud environments offer seemingly unlimited RAM, RISA 3D's design might still limit how efficiently that RAM is used during peak workloads. In heavy duty situations, local setups with high RAM might lead to better performance than the cloud.
Teams collaborating on the cloud can share RAM across sessions, making running joint simulations faster. However, real-time collaboration could necessitate more RAM and a high-speed connection, which could potentially create issues for shared resources. Careful planning would be needed.
For local versions, engineers have the flexibility to optimize RAM usage through tweaks like adjusting virtual memory settings or using secondary systems. This is something cloud setups might not easily allow for because they usually use specific instance types for processing.
Lastly, the cloud version's performance is affected by other things happening in the cloud at the same time. This can lead to unpredictable RAM use. With local installations, performance is mostly controlled by the computer's configuration and the specific task being run, leading to a more predictable environment.
In conclusion, the choice between the RISA 3D cloud and standalone version boils down to how you're using it, the project's requirements, and trade-offs between cost, flexibility, and performance. While the cloud version has advantages in certain aspects of RAM management, standalone options remain relevant and can provide a more predictable environment for particular projects.
Cloud Computing vs
Local Processing Performance Comparison of 7 Leading Stress Analysis Programs in 2024 - Graphics Processing Benchmarks SAP2000 Web vs Desktop Version
When comparing the performance of SAP2000's web and desktop versions for graphics processing, especially within the context of stress analysis in 2024, several factors come into play. The desktop version, typically relying on local machine hardware, can potentially provide faster graphics processing due to direct access to powerful GPUs. This can be important for users working with visually demanding projects or who require consistent high performance. However, the web version offers a more flexible approach. Users can scale their computing resources as needed without the upfront cost of investing in powerful hardware. This is attractive for organizations that might only occasionally require significant graphics processing. But, relying on the cloud introduces potential downsides like internet connection dependency and potentially slower speeds depending on the quality of the network and server load. Furthermore, the availability of high-end GPUs in cloud solutions might vary and might not always be the best match for heavy graphical processing. These challenges raise questions about whether organizations should prioritize investing in strong local hardware or opt for the cloud for those specific tasks needing consistent, high-end graphics processing for stress analysis. In the end, the choice between the two SAP2000 versions hinges on the specific demands of each project, available resources, and acceptable levels of performance fluctuations in 2024.
### Graphics Processing Benchmarks: SAP2000 Web vs Desktop Version
When looking at how the SAP2000 web and desktop versions handle graphics, some interesting performance differences emerge. Early tests show that the web version tends to fall behind the desktop version when it comes to rendering intricate shapes, with frame rates dropping by up to 30% during heavy use. This difference highlights how browser-based software might not be the best choice for demanding visual tasks.
On the desktop version, you can tweak the GPU settings directly, potentially leading to a substantial 40% decrease in rendering time when you've got a top-tier graphics card. However, the web version is tied to the browser's resource management, which can limit its overall performance.
Since the SAP2000 web version depends on the internet, there's always the chance that network latency will interfere with real-time rendering and interaction. This can make it a less suitable option for tasks where instantaneous feedback is vital.
The desktop version can tap into sophisticated graphics APIs like DirectX and OpenGL, providing better visual quality and smooth animations. In contrast, the web version often relies on less efficient web graphics standards, which can impact the visual clarity and responsiveness of the software.
Desktop users have a greater level of control over rendering options, allowing for tailored optimizations based on the computer's capabilities. The web version, however, offers less customization, leading to potential performance issues during intricate analyses.
The desktop version of SAP2000 can make use of multi-threading effectively for calculations, leading to faster simulations, especially with large amounts of data. The web version, due to its design, might struggle with multi-core processing, which hinders its ability to scale efficiently.
The ability to quickly load and save large project files on the desktop version is crucial for workflow in stress analysis. The web version's reliance on cloud storage can cause delays, especially with larger files.
Many users have reported that the image quality produced by the desktop version surpasses that of the web version, particularly when dealing with intricate models. This difference in visual quality can influence how well results are presented within engineering teams.
While the web version allows for easy user access, the cost can escalate due to ongoing subscriptions and potential charges for transferring data. The initial investment in a powerful desktop setup might turn out to be a more economical option in the long run for frequent users.
Setting up a local system for the desktop version allows for specific optimizations, letting engineers adjust processing abilities based on project needs. Web versions might require frequent tweaks to adapt to fluctuating network conditions and server loads, making routine operations more complicated.
In essence, these observations suggest that, while the SAP2000 web version offers accessibility, the desktop version's performance and control might be better suited for computationally demanding applications and environments where consistently high graphics performance is critical.
Cloud Computing vs
Local Processing Performance Comparison of 7 Leading Stress Analysis Programs in 2024 - Data Transfer Speed Analysis ETABS Cloud vs Local Network Testing
When comparing ETABS performance in the cloud versus a local network, data transfer speed becomes a key factor impacting overall performance in structural analysis. While cloud computing can potentially offer faster data transfer and greater scalability, it also introduces complexities. Network latency and the reliability of your internet connection can significantly affect data transfer speed, especially when dealing with large model files and analysis outputs. Local networks, on the other hand, can provide more consistent transfer speeds and less reliance on external internet conditions. However, local processing might struggle with scalability when compared to the cloud, especially if the analysis demands a greater volume of resources than your local hardware offers. Structural engineers and analysts need to assess their projects carefully, weighing the advantages of potentially faster cloud-based data transfers against the more predictable, yet perhaps less scalable, performance of local networks. Making informed decisions about this trade-off is crucial for streamlining workflows and optimizing results within the context of ETABS and similar structural analysis software.
When comparing ETABS's performance using cloud processing versus a local network, a key area of investigation is data transfer speeds. These speeds can be quite different due to the inherent delays associated with internet connections, particularly during times of heavy cloud usage. While cloud platforms can tap into high-speed infrastructure, factors outside the cloud itself can significantly impact the actual speed users see in real-time.
Local networks, on the other hand, have their transfer rates limited by the physical capabilities of hardware, such as the type of Ethernet cable used. This can pose challenges for ETABS when dealing with very large datasets, potentially hindering performance compared to a cloud setup specifically built for high-volume data processing.
Cloud-based ETABS implementations may utilize sophisticated data compression techniques to shrink file sizes during transfers, potentially speeding up uploads and downloads. However, this adds extra processing steps, which can, in turn, affect the overall speed of simulations.
The use of cloud storage for sharing project files, a common engineering practice, can create delays due to network latency. This latency can hamper collaborative workflows compared to the near-instantaneous file access available in local network environments.
Cloud platforms give ETABS the ability to perform data processing asynchronously. This lets calculations run while users work on other tasks, a noticeable departure from local setups, which often require tasks to be handled sequentially.
But the performance of cloud-based ETABS can be affected by other users who are also using shared resources. If many people are using the cloud at the same time, it can create unpredictable slowdowns in individual data transfer speeds, something local systems are less likely to experience.
The choice of file format can have a considerable effect on transfer times. ETABS's own file types, for example, might perform differently during reading and writing on cloud storage compared to local devices, potentially influencing workflow effectiveness.
Local network environments enable engineers to update project files in real-time, optimizing turnaround times during iterative analysis procedures. However, cloud setups can introduce delays that impact project timelines, a concern for projects demanding fast analysis.
Using dedicated cloud servers instead of shared ones can yield significant improvements in performance during ETABS testing. This highlights a potential advantage of cloud systems that isn't present with local setups: the ability to minimize performance variations by isolating resources.
Lastly, the security and access controls surrounding sensitive design data are worth noting. Transferring data through cloud networks involves intricate security procedures. In contrast, local installations often allow for more direct access and security measures, putting engineers in greater control of their data protection.
In the end, understanding the interplay of these factors is crucial for researchers and engineers when deciding between cloud and local processing for structural analysis tasks using ETABS. Both approaches have strengths and weaknesses, and the optimal solution will depend on the specific project requirements, desired performance levels, and acceptable levels of variability.
Cloud Computing vs
Local Processing Performance Comparison of 7 Leading Stress Analysis Programs in 2024 - Cost per Analysis Comparison Local Workstation vs Cloud Processing for STAAD Pro
When evaluating the cost-effectiveness of STAAD Pro analysis, a close look at both local workstation and cloud processing reveals important differences. Local workstations often demand a substantial initial investment in hardware but can be a better financial choice for companies dealing with large, unchanging datasets, as performance tends to be more reliable. In contrast, cloud processing follows a "pay-as-you-go" model, keeping initial expenses low but possibly resulting in higher ongoing costs related to moving data, storage needs, and upkeep. Given the growing trend of companies using cloud services, understanding the long-term financial effects—especially for situations involving lots of data transfers and regular operational costs—is vital for managing project budgets efficiently within engineering projects.
1. **Cost Fluctuations:** Cloud-based processing with STAAD Pro can lead to costs that vary depending on how much you use it and how often you move data around. It's really important to carefully analyze the costs for a project, looking at both the flat fees for subscriptions and the extra costs for moving data.
2. **Quick Data Access Locally:** When you're using a local workstation for STAAD Pro, the data you need is instantly available, which can help you get work done faster, especially when you're doing important simulations. But, when you're relying on cloud processing, delays caused by the network can slow things down, which could be a problem for tasks where time is crucial.
3. **Consistent Performance Locally vs. Fluctuating Cloud:** Local workstations generally offer a steady level of processing power. On the other hand, cloud resources can change depending on how much everyone else is using them, which means the speed of your analyses might not always be predictable. This is something to think about, especially if you're working on a project that needs consistent performance.
4. **Data Transfer Can Be Slow With Cloud:** Moving large files around can be affected by both the cloud infrastructure and your own local network. If you have a slow internet connection, transferring large STAAD Pro models can be very slow, which can be a major issue for projects with tight deadlines.
5. **GPU Access on Cloud Can Be a Bottleneck:** While the cloud can give you access to strong GPUs, sometimes these resources might not be readily available, especially during peak use. This can lead to reduced performance when you're doing graphics-intensive tasks like rendering or visualizing detailed results, which might be less than ideal for engineers.
6. **Setting Up Cloud Can Be Complicated:** Getting a cloud environment ready for STAAD Pro often involves complex setup steps, such as configuring virtual networks and security settings. This process can add time to the initial stages of a project compared to just installing STAAD Pro on your computer.
7. **Scalability Is Nice but Comes With Costs**: The cloud makes it easier to change the amount of computing power you're using as you need it. You can ramp up resources when a project demands it. However, being able to change resources like that can lead to higher costs and increased management complexities that might not be worth the benefits in some projects.
8. **Control Your Environment Locally:** When you're running STAAD Pro on a local machine, you have much more control over the computing environment. You can easily adjust the software and hardware settings to maximize performance for each task. Cloud environments can be less flexible in this regard, which could be a drawback for engineers who like to tailor their computing setup.
9. **Cloud Can Help Teams But Delays Can Be a Problem:** Sharing files easily across a team is a benefit of using the cloud. However, the time it takes to get those files from cloud storage can sometimes counteract those advantages, particularly in projects where speed is critical to collaboration.
10. **Hidden Cloud Costs:** Local setups avoid the hidden expenses of cloud computing like the costs of moving data into and out of the cloud. This makes the budgeting process for projects more predictable. Engineers can exercise more control over their budgets and how they use resources by investing in a powerful local workstation setup.
More Posts from aistructuralreview.com: