Multiphysics Simulation Software Key Advances in Electromagnetic-Thermal Coupling Analysis for 2025
Multiphysics Simulation Software Key Advances in Electromagnetic-Thermal Coupling Analysis for 2025 - Neural Networks Enable Real Time Heat Distribution Modeling in Electromagnetic Fields
Neural networks, specifically those informed by physics principles (PINNs), are emerging as a powerful tool for modeling heat distribution within electromagnetic fields. This approach leverages the strengths of machine learning to create significantly faster solvers for multiphysics problems. By combining established methods like the Finite-Difference Time-Domain (FDTD) with neural networks, real-time temperature estimation becomes achievable with a high degree of accuracy. The physics-informed UNet exemplifies this, replacing traditional approaches for solving the heat equation and demonstrating considerably faster inference times compared to established finite element methods.
Further, the adaptability of PINNs is notable. They can function autonomously or alongside conventional simulation techniques, giving engineers a broader set of tools to tackle intricate electromagnetic and thermal coupling scenarios. This flexibility makes them a compelling option in a variety of applications. As neural networks continue to advance, their use in electromagnetic modeling is expected to improve both simulation accuracy and robustness, finding increasing use in areas like optimizing electric motor design. While the initial focus is on speed and accuracy, ongoing research and development in this domain will likely yield further benefits for multiphysics modeling across diverse industries.
Researchers are exploring the use of neural networks to create more efficient and insightful models of heat distribution within electromagnetic fields. This approach leverages the ability of neural networks to handle complex datasets, offering a potential pathway to significantly accelerate simulation times. Instead of the hours or even days that conventional finite element methods might require, neural network-driven simulations can potentially deliver results in a matter of minutes, making real-time analysis a tangible possibility.
The ability to witness heat distribution in real-time could be transformative. Engineers can immediately see the impacts of design alterations or material choices directly within the simulation environment. This dynamic feedback loop offers a powerful tool for iterative design processes and enables on-the-fly optimization. Interestingly, these neural networks also hold promise for identifying nonlinear material behaviors that might go undetected using standard methods. This opens doors to a deeper understanding of how materials behave under complex electromagnetic and thermal conditions.
Furthermore, unlike classical analytical models, neural networks can learn from previous simulation data. This "machine learning" aspect can lead to continually improving model accuracy with experience. This approach also potentially simplifies the setup process, reducing the need for extensive mesh generation—a step that can be both complex and error-prone in traditional simulations.
Predicting thermal hotspots is a crucial aspect of ensuring the safety and reliability of electromagnetic systems. Neural networks seem particularly adept at this task, enabling engineers to pinpoint these potential failure points and develop mitigation strategies. The flexibility of neural networks is also a major advantage in real-time simulations, making them easily adaptable to various operating conditions without extensive recoding.
This application of neural networks to electromagnetic-thermal simulations could pave the way for more efficient design practices. The potential to optimize thermodynamic performance with minimal computational overhead is particularly enticing. Moreover, neural networks might uncover hidden correlations within multidimensional data that traditional methods struggle to identify, leading to enhanced accuracy in predicting the thermal behavior of intricate systems.
The future of this field hinges on continued advancements in neural network architectures and training methodologies. If these networks can be further refined to effortlessly handle various electromagnetic data, including both transient and steady-state responses, they could revolutionize how engineers approach thermal management in complex systems. While many questions remain, the potential for neural networks to significantly impact the field of multiphysics modeling is undoubtedly intriguing.
Multiphysics Simulation Software Key Advances in Electromagnetic-Thermal Coupling Analysis for 2025 - Advanced Temperature Control Systems for EV Battery Safety Through Multiphysics Analysis
Ensuring the safety and longevity of electric vehicle (EV) batteries is paramount, and advanced temperature control systems are emerging as crucial components in achieving this. The field is witnessing a growing emphasis on multiphysics analysis, where machine learning techniques are being incorporated to create more comprehensive models of battery thermal behavior. This allows researchers and engineers to better understand the intricate interplay between thermal, electrochemical, and degradation processes within lithium-ion batteries.
One promising area of development involves the use of phase change materials (PCMs) in battery thermal management systems. These materials can effectively absorb excess heat generated during operation by transitioning between solid and liquid states, providing a more efficient means of preventing overheating.
Furthermore, there is an increasing focus on understanding and mitigating the risks of thermal runaway, including battery fires. This has led to more sophisticated electromagnetic-thermal coupling analyses, which seek to provide more accurate predictions of potential failure scenarios.
Ultimately, the trend towards more advanced cooling strategies and real-time thermal monitoring signifies a shift towards a future where battery safety is a core design priority. These innovations are expected to play a key role in ensuring EV batteries can maintain optimal performance and lifespan, even under the most demanding operating conditions. However, it is important to validate the accuracy of these advanced models through rigorous testing and validation before deploying them in full-scale applications.
Maintaining the safe operation of electric vehicle (EV) batteries is paramount, and advanced temperature control systems are playing a crucial role in this endeavor. Understanding and preventing thermal runaway, a catastrophic event where batteries overheat and potentially ignite, is a central focus. Multiphysics analysis has emerged as a valuable tool for gaining insights into the intricate interplay of thermal, electrochemical, and degradation processes within batteries. By combining machine learning and multiphysics models, researchers are developing frameworks that can accurately predict the onset of thermal runaway.
The ability to model battery behavior across multiple scales is particularly insightful. We can now examine how material properties at the micro-level impact the overall system behavior at the macro-level. This deeper understanding is critical for developing robust thermal management solutions. Phase change materials (PCMs) are gaining attention as a potential solution. These materials are designed to absorb excess heat during operation by undergoing a solid-to-liquid phase change, providing an effective buffer against temperature extremes.
Beyond just thermal management, these advanced simulations are starting to consider the effects of electromagnetic interference (EMI) on battery thermal behavior. Understanding how EMI impacts heat generation and distribution is vital for designing appropriate shielding in EV battery packs. Further, real-time thermal control is becoming a reality. By incorporating sensors and leveraging dynamic feedback control, thermal management systems can adapt to varying environmental conditions and driving demands. This adaptability enhances safety and performance across a wider range of operating scenarios.
The geometry of battery packs has a significant influence on how heat is distributed, and multiphysics simulations are revealing the nuanced interplay between design and thermal management. Even subtle alterations in geometry can have a substantial effect on heat flow, highlighting the importance of meticulous design optimization. In a similar vein, understanding thermal interface materials (TIMs) is essential for improving heat transfer. Simulations can guide the selection of optimal TIMs to minimize thermal resistance and maximize efficiency.
Accelerated aging tests, enabled by multiphysics simulations, are proving valuable for evaluating the long-term performance and reliability of battery materials under thermal stress. This ability to accelerate aging and predict failure modes allows for more informed design choices. It's notable that battery management systems (BMS) and temperature control systems are becoming increasingly integrated. This tight coupling allows for real-time adjustments based on electrochemical data, leading to optimized battery performance while enhancing safety.
The ultimate goal is to create batteries that are robust and resilient across a range of extreme conditions. Multiphysics simulations are providing the means to explore battery behavior in situations such as rapid discharge, high temperatures, and mechanical stress. This enhanced predictive capability offers a pathway to design safer and more durable batteries that can withstand rigorous operational demands. While there's still much to learn, these advancements in multiphysics simulations are proving incredibly valuable for improving EV battery safety and performance.
Multiphysics Simulation Software Key Advances in Electromagnetic-Thermal Coupling Analysis for 2025 - Surface Impedance Method Reduces Computation Time in 3D Electromagnetic Studies
The Surface Impedance Method offers a promising way to speed up complex 3D electromagnetic simulations. By reducing the number of unknowns in the problem from six to three per element, it significantly cuts down on the computational resources needed to solve these problems. This translates into faster simulation times and reduced memory demands. Importantly, this method produces results that are quite accurate when compared to established, exact solutions like the Mie series, especially within the context of 3D eddy current calculations.
However, challenges remain. The accuracy of the method near sharp edges or corners can be affected by rapidly changing surface impedances, requiring special treatment. Looking ahead, research and development related to this technique is likely to focus on improvements that address convergence issues plaguing existing iterative solver approaches and on enhancing the accuracy of electromagnetic scattering analyses. It's expected that refinements to the method will allow researchers to better tackle complex 3D electromagnetic scenarios with greater speed and reliability.
The Surface Impedance Method offers a compelling approach to accelerate 3D electromagnetic simulations, particularly in scenarios where computational resources are limited. By essentially simplifying the representation of complex 3D surfaces into equivalent impedance boundaries, it reduces the number of variables in the simulation from six to three per element. This, in turn, significantly decreases the time and memory needed for simulations, potentially cutting down the time it takes to find a solution from hours to minutes.
While this reduction in complexity is beneficial, it's not without its caveats. For example, accurately representing rapidly changing impedances near sharp edges or corners can be tricky, demanding special attention in the simulation setup. Nonetheless, this method's strength lies in its ability to provide numerically sound results in scenarios involving eddy currents, showing strong agreement with exact solutions like the Mie series.
Interestingly, this method isn't confined to a single type of solver. It can be integrated with various established techniques, such as Finite Element Method (FEM) or Boundary Element Method (BEM), broadening its applicability across different design scenarios. This integration has the added advantage of easing the complexity of mesh generation, a crucial step in most electromagnetic simulations. Simplified meshes directly translate to fewer potential sources of error and faster simulation setups.
The benefits extend to real-world applications. In fields like automotive engineering, where components like antennas and sensors play increasingly important roles, the Surface Impedance Method can help streamline the design process. Designers can more efficiently iterate through different design options due to the reduced simulation time, ultimately saving them valuable time and resources. Moreover, the method's adaptability allows engineers to account for materials with varying electromagnetic properties (anisotropic materials), improving the accuracy of the simulation.
Furthermore, the Surface Impedance Method can facilitate faster feedback on design changes if combined with real-time simulation platforms. In the future, this could lead to an interactive design environment where changes are immediately visualized, enhancing design iterations considerably. And while this is primarily a method for accelerating numerical simulations, it has implications for broader electromagnetic concerns, such as Electromagnetic Compatibility (EMC) in a design. With more efficient simulation, understanding how components will behave in real-world electromagnetic environments becomes less computationally intensive, and therefore more accessible for researchers.
It's important to recognize the method's utility in scaling up the complexity of the model without a direct increase in computational costs. This makes it particularly well-suited for studying large-scale systems. Finally, the fundamental concepts behind the Surface Impedance Method also open doors for further research. By carefully modeling the interactions of electromagnetic waves with different materials, the method has the potential to contribute to a deeper understanding of wave interactions, with implications across various fields from telecommunications to radar systems. While not a panacea, the Surface Impedance Method holds the potential to accelerate 3D electromagnetic simulations in ways that would have seemed impossible only a few years ago, fostering faster innovation across various industries.
Multiphysics Simulation Software Key Advances in Electromagnetic-Thermal Coupling Analysis for 2025 - Integration of Aeroacoustic Effects in Power Electronics Thermal Management
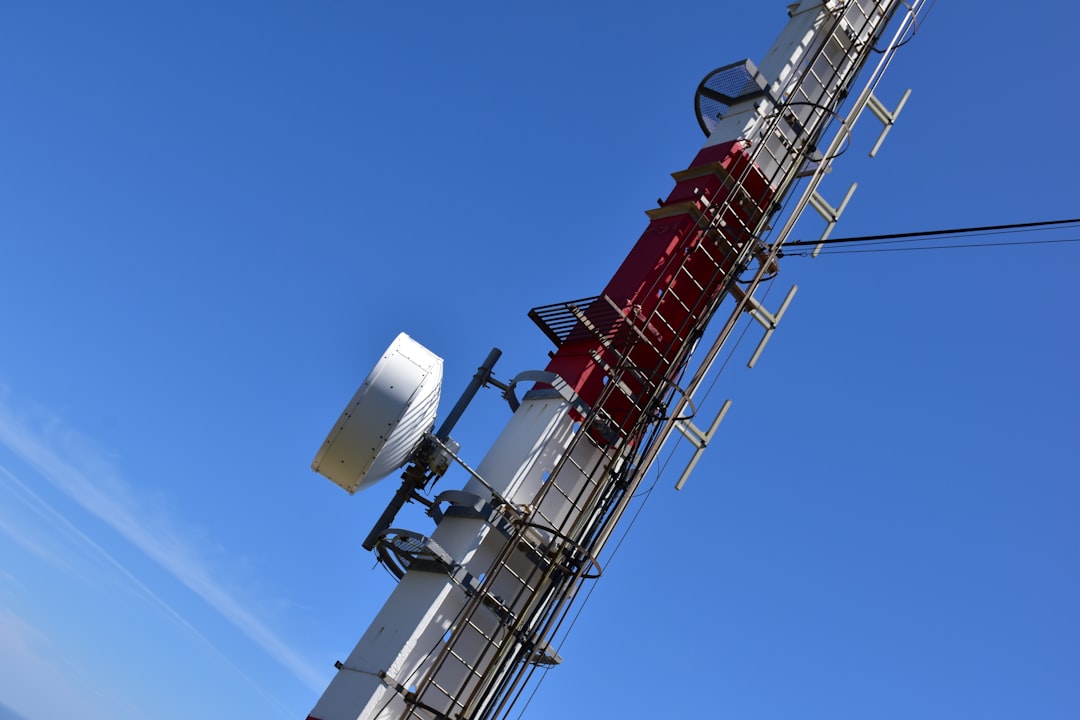
The growing importance of high-power electronics in various applications necessitates a deeper understanding of how aeroacoustic effects influence their thermal management. This is because the increased power densities and operating frequencies of modern power electronics generate significant heat, often leading to operational challenges and potential component degradation. Until recently, thermal management analyses largely focused on purely thermal and electromagnetic effects. However, recognizing the impact of noise and turbulence generated by cooling systems, it's now vital to consider how airflow dynamics contribute to overall thermal behavior.
Integrating aeroacoustic effects into multiphysics simulations offers a more holistic view of the thermal environment of these devices. Such models allow engineers to analyze the complex interactions between the turbulent airflow, noise propagation, and heat transfer mechanisms. By comprehending these coupled phenomena, better cooling solutions can be designed. The goal is not only to improve heat dissipation but also to mitigate any potential negative consequences of noise on device performance and reliability.
This integration of aeroacoustic phenomena is especially relevant in high-power scenarios where more compact packaging is desired. As system designers try to pack more power into smaller spaces, careful consideration of cooling systems and their associated noise become crucial. As simulation software evolves to provide greater precision in modeling these complex coupled phenomena, a more nuanced understanding of these systems will arise, ultimately paving the way for more optimized and resilient designs.
The influence of sound and air movement (aeroacoustics) on the thermal behavior of power electronics is becoming increasingly important. These effects can significantly alter heat transfer and thermal resistance within cooling systems, making it crucial for engineers to understand them. For example, the way cooling systems are designed and the materials they're made of needs to account for how airflow and sound might impact heat transfer.
The interaction between air movement, sound waves, and the electromagnetic fields generated by power electronics creates a complicated design challenge. Turbulence and airflow generated by the power electronics themselves can worsen heat buildup in components. This intricate interplay necessitates complex multiphysics models to accurately predict how they will behave in real-world situations. We can no longer ignore this interplay.
By incorporating aeroacoustic modeling into thermal management strategies, we can simultaneously reduce noise and vibration in power electronics. This is becoming more and more important, particularly for devices like electric vehicles and consumer electronics, where quiet operation and user experience are key considerations.
Precisely predicting how heat is affected by aeroacoustic forces requires using advanced numerical techniques like Large Eddy Simulation (LES). LES can capture the intricate details of turbulent airflow around the power electronics, providing a more accurate view of what is happening.
Being able to simulate aeroacoustic effects in real time can be incredibly helpful. Designers can immediately see the impact of changes to a design and make adjustments as they work. This capability is particularly useful in rapid prototyping environments where quick tweaks to thermal performance are needed to get the most efficient design possible.
By integrating fluid dynamics and acoustics into our thermal analysis, we can better identify thermal hotspots. This early identification of potential failure points is a significant advantage and reduces the need for a lot of physical prototypes.
Using sophisticated computational methods for aerodynamics within our thermal management systems is revealing unexpected design opportunities. For example, certain airflow patterns can actually improve heat transfer, which might not be obvious using traditional methods.
Teams of engineers are increasingly relying on integrated simulation platforms to streamline their design process. This means they're moving towards combining thermal, aerodynamic, and acoustic modeling into one software platform, eliminating the need for numerous, separate tools. This streamlines things and potentially cuts down on errors.
The close connection between aeroacoustics and thermal performance is making us rethink how we design cooling systems. This is leading to new approaches, like the exploration of vortex generators and microchannel heat exchangers, to optimize heat removal.
The demand for smaller, higher-performance power electronics is pushing us to integrate aeroacoustics into thermal management. This is shifting design approaches towards more comprehensive, multiphysics solutions, which are essential for meeting future needs.
Multiphysics Simulation Software Key Advances in Electromagnetic-Thermal Coupling Analysis for 2025 - Machine Learning Algorithms Speed Up Electromagnetic Field Calculations
Machine learning algorithms are increasingly being used to accelerate and improve the accuracy of electromagnetic field calculations, a critical component of multiphysics simulation software, especially for scenarios involving electromagnetic-thermal coupling. These algorithms, including those based on physics-informed neural networks, are being integrated with established techniques like the Finite-Difference Time-Domain (FDTD) method to create faster and more accurate solutions to complex problems that combine thermal and electromagnetic effects. The ability to construct generalized surrogate electromagnetic solvers using deep convolutional neural networks is a particularly promising development, potentially leading to both higher accuracy and considerably faster simulations. Furthermore, deep active design optimization methods are being developed with the goal of improving how we explore and refine designs in multiphysics systems, but the complexities of efficiently leveraging design spaces remain a challenge. These advances demonstrate that machine learning is poised to revolutionize how we approach electromagnetic modeling and simulation. While there are limitations to overcome, the potential for these methods to significantly improve performance prediction and design optimization is undeniable.
The integration of machine learning algorithms into electromagnetic field calculations is reshaping how we approach these problems. Deep learning, in particular, is proving exceptionally adept at handling large datasets far more swiftly than traditional techniques, significantly accelerating simulation times. Instead of waiting hours for results, we can now potentially achieve a solution in mere minutes, greatly boosting the pace of design iterations.
One of the key advantages of machine learning is its adaptive learning capability. Unlike rigid mathematical models based on fixed equations, these algorithms can dynamically adapt to changes in design parameters. Engineers can modify aspects of a design, and the machine learning model swiftly updates its predictions, allowing for quicker assessments without the need for extensive recalibration. This nimble feedback loop speeds up the design optimization process considerably.
Furthermore, the capacity of machine learning for identifying errors within simulation models is notable. By comparing simulated outputs with observed data, discrepancies can be pinpointed, highlighting potential weaknesses in the models. This error detection aspect is a boon for enhancing simulation reliability, as it encourages a deeper dive into areas that classical methods might overlook.
Moreover, machine learning algorithms are proving exceptionally useful for understanding complex material interactions, particularly in the realm of nonlinear behaviors. Uncovering the intricate ways materials respond to diverse electromagnetic fields has always been challenging for conventional models. The multidimensional nature of this data can be effectively handled by machine learning models, leading to a richer and more accurate representation of real-world phenomena.
Some machine learning approaches, such as Principal Component Analysis (PCA), provide mechanisms for reducing the dimensionality of the datasets involved in electromagnetic simulations. This feature is advantageous as it simplifies data interpretation while still preserving core information. In addition, machine learning's ability to tackle multiscale problems is critical for bridging the gap between micro-level material behaviors and their impact on larger-scale systems.
The application of machine learning also offers the potential for establishing real-time feedback loops within simulation workflows. The immediate visualization of design alterations and their impact allows for quicker evaluations and facilitates more informed decision-making. This is particularly valuable during the prototyping phase.
The inherent scalability of machine learning algorithms is another significant benefit. Incorporating additional data or complexities into models doesn't necessarily equate to a large increase in computational resources, unlike with many conventional methods. This scalability is particularly advantageous for the simulation of massive and intricate electromagnetic systems.
Another noteworthy benefit of machine learning lies in its ability to improve predictive maintenance. With machine learning models, it's possible to identify potential failure points, such as those linked to thermal fluctuations in electromagnetic systems, which is a vital element for preventing costly failures.
The utilization of machine learning within electromagnetic field calculations is also promoting increased collaboration across diverse engineering disciplines. Data science and computational physics are contributing insights that enrich conventional electromagnetic simulation techniques, fostering a new era of hybrid approaches. While challenges certainly remain, the integration of machine learning is already demonstrating its power for advancing our understanding of and ability to design with electromagnetic systems.
Multiphysics Simulation Software Key Advances in Electromagnetic-Thermal Coupling Analysis for 2025 - Next Generation Time Domain Methods for Complex Material Analysis
The development of Next Generation Time Domain Methods is pushing the boundaries of electromagnetic simulation, particularly when dealing with complex materials. The Finite-Difference Time-Domain (FDTD) method, a well-established approach, continues to be a core tool for exploring electromagnetic fields in intricate structures. However, it's the emergence of hybrid techniques that is most noteworthy. These approaches couple FDTD with machine learning, for instance, through physics-informed neural networks. This fusion results in solutions to multiphysics problems that can be achieved in real time. It represents a substantial leap forward in simulation power. The ability to accurately model how complex materials behave under electromagnetic and thermal conditions is a major strength of these next-gen approaches, often outperforming traditional methods. There are ongoing hurdles, yet the progress is undeniable. The future of electromagnetic-thermal coupling analysis likely relies on these kinds of advances. By 2025 and beyond, expect these methods to become more sophisticated, flexible, and widely used in creating superior simulation tools.
Time domain methods are proving increasingly valuable for analyzing complex materials, especially in the context of electromagnetic-thermal coupling. The ability to visualize temperature distributions in real-time as simulations run—something not readily available in traditional methods—is greatly accelerating the design process. It allows engineers to quickly see the effects of changes to a design and adjust accordingly.
We're also beginning to gain a better understanding of how materials behave under dynamic electromagnetic and thermal conditions. These next-generation time domain techniques are better able to capture nonlinear material responses, providing insights often missed by more traditional methods. This is particularly important when dealing with scenarios where materials experience rapid changes in temperature or electromagnetic fields.
Another significant advancement is the integration of homogenization techniques. This allows for more efficient modeling of composite materials. Instead of having to deal with the intricate details of every constituent within the material, these approaches can effectively average out the complex interactions into simpler, yet accurate, material properties. This makes simulating materials with complex internal structures more manageable.
Further, we're seeing more sophisticated coupling between the electromagnetic and thermal domains with other physical phenomena like mechanical stress and fluid flow. This helps us develop a more complete picture of how these systems truly behave, especially in dynamic situations. However, the intricacies of successfully coupling different physical domains can be challenging, with careful consideration needed to avoid errors and ensure solution validity.
Adaptive meshing has also made strides in improving the efficiency of time domain simulations. By focusing computational efforts only in regions where significant changes occur, these methods significantly cut down the computational time without sacrificing accuracy. These methods require sophisticated algorithms to determine where refinement is needed but can result in considerable time savings, especially in complex geometries.
The development of more advanced time-stepping schemes has also improved the stability and convergence of time domain simulations, leading to greater accuracy and longer simulation times without a noticeable degradation in performance. Care needs to be taken in implementing these advanced schemes, and the selection of appropriate schemes can heavily influence the overall simulation performance.
Dynamic boundary conditions are now more readily accommodated in time domain methods. This means we can more accurately reflect real-world scenarios with fluctuating environmental conditions, like fluctuating heat loads or rapidly rotating components. This ability to include dynamic conditions adds a higher level of realism, but also adds complexities that require a more comprehensive understanding of the problem.
Multi-scale simulations, previously a significant challenge, are becoming more feasible with time domain methods. We can now study materials from their microscopic interactions to their macroscopic effects, giving us a more comprehensive understanding of how a particular material or system will behave. While promising, these multi-scale methods require careful attention to detail and the correct application of simplifying assumptions.
We've seen significant advancements in algorithms that aim to minimize the computational load for time domain methods. This has opened up the possibility to tackle complex problems that were previously computationally too expensive. The continued advancement of these computational methods is crucial to further broaden the scope of problems that can be addressed.
Finally, the incorporation of data-driven methods within time domain simulations is an exciting development. By using past simulation data to refine the accuracy of future simulations, the simulation process effectively becomes self-improving. This is similar to machine learning in other fields but needs to be applied carefully to avoid introducing bias or errors into the process.
While still in their infancy, these next-generation time domain methods have the potential to significantly enhance our ability to model and simulate complex materials, with the potential for revolutionizing the design of novel systems that can function in extreme conditions.
More Posts from aistructuralreview.com: