Revolutionize structural engineering with AI-powered analysis and design. Transform blueprints into intelligent solutions in minutes. (Get started for free)
Engineering Week 2025 How Structural Engineers Will Shape the Future of AI-Integrated Infrastructure from February 16-22
Engineering Week 2025 How Structural Engineers Will Shape the Future of AI-Integrated Infrastructure from February 16-22 - Machine Learning Models Predict Bridge Maintenance Schedules at MIT Infrastructure Lab
Researchers at the MIT Infrastructure Lab are exploring the use of machine learning to refine bridge maintenance schedules. Their system employs machine learning models to assess the condition of a set of 95 bridges, specifically focusing on concrete bridge types. Bridges in good condition, with a General Condition Rating above 7, are excluded as they are not deemed to be in urgent need of repairs. The approach leverages past performance data, though the limited availability of such data for many infrastructure agencies might pose a challenge. Interestingly, their research seeks to improve not just structural maintenance decisions, but also looks at how to schedule maintenance in ways that might minimize carbon footprint. By linking machine learning with linear programming, the goal is to develop a more efficient and environmentally-conscious bridge maintenance approach. This initiative signifies a move towards applying AI within civil engineering to address the challenges of managing a vast and aging infrastructure network. While the field of AI-based infrastructure management is still developing, this work suggests exciting possibilities for the future. It remains to be seen whether this technology can truly translate into significantly improved bridge maintenance strategies and more efficient use of public resources.
Researchers at MIT's Infrastructure Lab are exploring the use of machine learning to revolutionize bridge maintenance scheduling. They've developed a system that combines machine learning with linear programming to predict the condition of bridge components and optimize maintenance interventions. This approach leverages data from a large number of bridges, primarily focusing on continuous and prestressed concrete structures. Interestingly, bridges in excellent condition (those with a General Condition Rating above 7) were excluded from their analysis, as they wouldn't be expected to need immediate attention.
The core of this work is using historical bridge performance data to train machine learning models. However, a significant challenge lies in the fact that many agencies may not have the kind of extensive historical data that these models thrive on. Nonetheless, the potential applications for machine learning in bridge engineering are vast, ranging from assessing structural strength and predicting potential failures to optimizing maintenance schedules and repair costs.
Their approach includes developing a more refined inspection process, incorporating machine learning insights to help engineers assess bridges and plan maintenance strategies. Furthermore, they're looking into how maintenance schedules can be optimized not only for safety and service reliability but also for environmental sustainability by minimizing CO2 emissions.
Essentially, they're creating a kind of comprehensive bridge asset management system that keeps track of bridges across their entire lifespan, from initial construction through operation and ongoing maintenance. This detailed approach is particularly important considering that any structural damage can impact a bridge's safety, integrity, and longevity. The researchers point out that despite typical design lifespans of over 100 years for bridges, the need to manage aging infrastructure is increasing.
The research suggests that machine learning has the capacity to significantly refine bridge maintenance. However, the integration of these technologies requires careful consideration. While promising, the reliance on these machine learning models brings about questions regarding the interpretability of their outputs. Striking a balance between the insights derived from the models and traditional engineering judgment will be a critical aspect of realizing the full potential of this technology in the years to come.
Engineering Week 2025 How Structural Engineers Will Shape the Future of AI-Integrated Infrastructure from February 16-22 - Structural Health Monitoring Drones Map NYC Building Facades Through Neural Networks
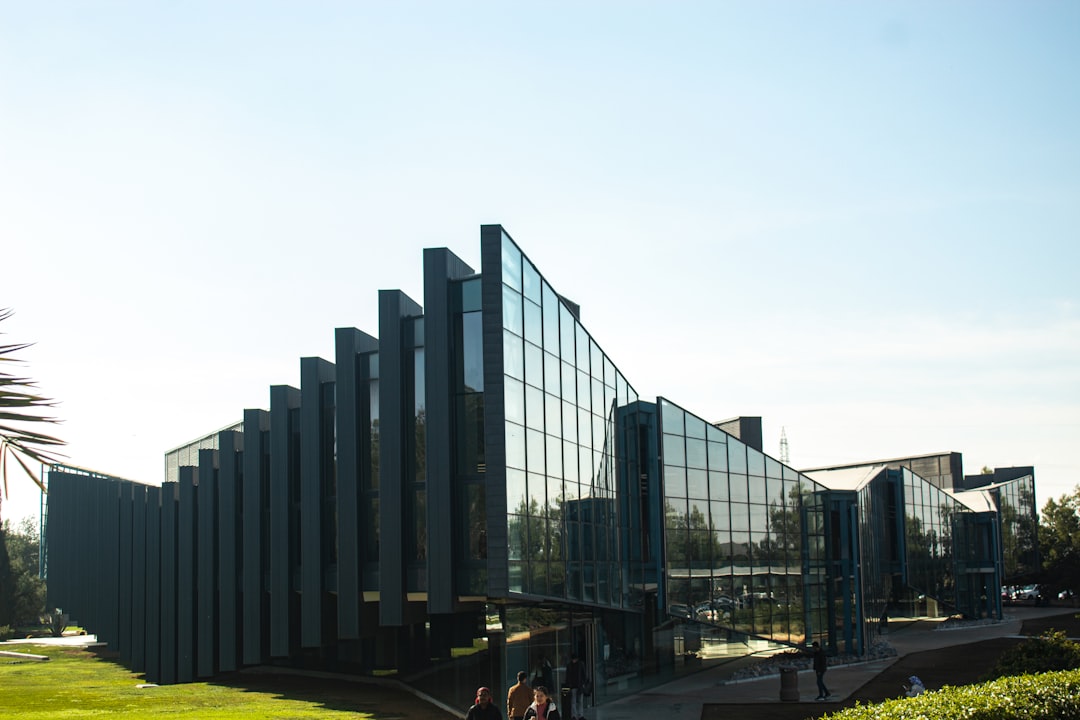
In the ever-growing urban landscape, particularly in cities like New York, the need for efficient and accurate methods of assessing structural health is becoming increasingly important. Drones, equipped with advanced technologies, are emerging as a powerful tool for structural health monitoring (SHM) of building facades. By using neural networks and deep learning algorithms, these unmanned aerial vehicles can provide detailed and non-destructive inspections of building exteriors. This automated inspection process allows for the rapid detection of potential issues like cracks, corrosion, and deterioration, facilitating prompt maintenance decisions.
The application of artificial intelligence within SHM represents a significant leap in infrastructure management. Machine learning models are able to analyze large datasets gathered from drone inspections, enabling engineers to make faster and more informed decisions about building safety. This intersection of technology and engineering suggests a future where AI-driven systems play a key role in maintaining our urban environments.
However, the increasing reliance on AI for structural assessment also requires a careful consideration of its limitations. As these complex algorithms become more integral, it's essential to balance their automated insights with the established knowledge and experience of structural engineers. The ability to interpret the data generated by these systems and avoid potential biases is paramount to ensuring safe and reliable infrastructure. These aspects will certainly be a focus of Engineering Week 2025, where the future of AI-integrated infrastructure management will be explored.
Structural health monitoring (SHM) is increasingly relying on drones equipped with a suite of sensors to gather high-resolution visual, thermal, and lidar data. This allows for the generation of detailed 3D models of building facades, providing a more precise way to assess their condition compared to traditional methods. The use of drones for these inspections offers a non-destructive approach, which is particularly valuable in urban environments. However, analyzing all that visual information can be overwhelming.
The integration of neural networks with drone-based data collection is proving to be a game-changer. These networks are able to automate the detection of anomalies like cracks or material degradation on building facades, streamlining the inspection process. This automation could lead to a reduction in manual inspections, freeing up human inspectors for other tasks.
One of the key advantages of drone-based SHM is the ability to quickly cover vast urban areas. This allows for simultaneous evaluation of numerous structures, which could greatly accelerate the process of identifying maintenance needs across a city like New York. By rapidly gathering and processing data from various buildings, engineers could potentially streamline maintenance scheduling and repair prioritization.
The power of this approach lies in the fusion of drone technology and machine learning. Data analysis, which might have taken human inspectors weeks, can be completed in a matter of hours by intelligent algorithms. This shift towards data-driven decision-making represents a fundamental change in how structural health monitoring is being conducted.
Drones provide a solution for inspecting hard-to-reach areas of building facades, reducing the need for scaffolding or lifts, which lowers safety risks for human inspectors. It's encouraging to see how technology can be used to make these jobs less hazardous. However, relying solely on automated systems raises concerns about the trade-off between efficiency and thoroughness, particularly when dealing with complex structural issues.
Research suggests that the accuracy of machine learning models used in drone-based SHM can improve over time as they analyze a greater number of inspection examples. This capacity for learning from past data could enhance the ability of these models to detect increasingly subtle structural flaws in buildings. However, the reliability of these models depends heavily on the quality and quantity of data they're trained on.
The application of drones in structural health monitoring is gaining momentum, not just because of the increased efficiency, but also due to the enormous amount of data they can collect. This information can be valuable for improving future design considerations and material selection. However, there's a need to ensure the long-term availability and reliability of these data sources for their value to be truly realized.
Neural networks can be trained using data from past inspections of similar buildings. This capability allows drones to leverage historical insights when examining newer structures, which can enhance the precision of their defect identification. It's important to ensure that training datasets are representative and encompass the range of building types and environmental conditions encountered in real-world applications.
Despite the numerous advantages of using drones and neural networks, there are some challenges that need to be addressed. Standardizing data collection and analysis procedures is crucial to ensure the comparability and reliability of inspection results across different buildings and engineering firms. This standardization will promote better communication and understanding of the condition of structures within a region or city.
The intersection of drone technology and artificial intelligence is poised to reshape the field of structural health monitoring. The future of SHM likely involves systems capable of not only assessing the current condition of structures but also forecasting future deterioration trends. These predictive capabilities could allow for proactive maintenance strategies that optimize resource allocation and enhance the longevity of our built environments. But, to reach that full potential, the field needs to invest in greater understanding of the AI systems and ensuring they remain aligned with the underlying engineering principles and traditional methods of assessment.
Engineering Week 2025 How Structural Engineers Will Shape the Future of AI-Integrated Infrastructure from February 16-22 - Berlin Engineers Deploy AI Earthquake Response System for Historic Buildings
Engineers in Berlin have developed a novel AI-based earthquake response system specifically for historic buildings. This system aims to improve the safety and structural integrity of these important structures during seismic events. It leverages AI to remotely assess the building's condition and helps determine appropriate responses, including potential retrofitting solutions. This initiative is a compelling example of how AI is being incorporated into structural engineering, especially for challenges related to preserving historical structures while ensuring they withstand natural disasters. It demonstrates the evolving role of AI in structural engineering and its potential to refine safety standards in the face of climate change related risks. While the system's effectiveness remains to be seen, it highlights the ongoing development of innovative approaches to protecting our built heritage from the hazards of earthquakes. The success of this system could signify a paradigm shift in how structural engineers protect historically important buildings in seismic areas. There is a potential for this to be adopted in other regions.
Engineers in Berlin are developing an intriguing AI-driven earthquake response system specifically tailored for the unique challenges posed by historic buildings. This system incorporates a network of sensors within these structures, allowing for continuous monitoring and real-time data analysis. The hope is that this system will offer more robust and adaptable responses compared to traditional earthquake mitigation strategies that might not fully account for the specific structural behaviors of these older buildings.
One of the key features of this system is its ability to rapidly process various data streams during an earthquake. This is particularly relevant for historic structures, where the complex interplay of diverse building materials and architectural styles can lead to unpredictable responses during seismic events. By analyzing data in real-time, the AI can potentially enable faster assessments of structural integrity and inform quicker decisions regarding evacuations.
The system's design isn't merely reactive. It leverages machine learning to learn from past earthquake data and adapt its response strategies accordingly. The goal is to develop a system that can not only respond to earthquakes but also learn and improve its predictive capabilities over time. Interestingly, the researchers have also incorporated simulation techniques that can model thousands of potential earthquake scenarios. This allows them to better understand how a building might react to various seismic conditions, which may uncover potential failure points that wouldn't be readily apparent through traditional engineering methods.
Furthermore, the AI system integrates with existing building management systems, creating a kind of "digital twin" for each historic structure. This digital representation continuously updates its risk profile based on a range of environmental factors, which can then inform ongoing maintenance and adaptation strategies. This approach could lead to more proactive building management and, hopefully, improved resilience to seismic events.
The system isn't designed to function in isolation. It's built to seamlessly communicate with emergency response services, potentially streamlining resource allocation and improving coordination during crises. This collaborative element is particularly crucial when it comes to protecting both human life and cultural heritage within vulnerable urban environments. This integration, however, presents its own challenges, requiring a careful consideration of the complexities of real-time information flow within emergency response networks.
While the system promises improved resilience and response capabilities, it's important to recognize that it's not a panacea. The AI's output still needs to be interpreted and verified by experienced structural engineers. The focus on balancing the strengths of the AI with the expertise of human engineers is a central theme in this development. It's crucial that AI-generated recommendations are vetted against established engineering principles and building codes to ensure safety and avoid unintended consequences.
This initiative raises broader considerations about the intersection of historic preservation and modern safety requirements. The insights gained through the AI earthquake response system could lead to important discussions around urban planning and building codes as they relate to historical districts and the need to balance preservation with the realities of seismic hazards. It's a compelling area where engineering and heritage management collide.
Engineering Week 2025 How Structural Engineers Will Shape the Future of AI-Integrated Infrastructure from February 16-22 - Digital Twin Technology Reduces Construction Time at Singapore High Rise Project
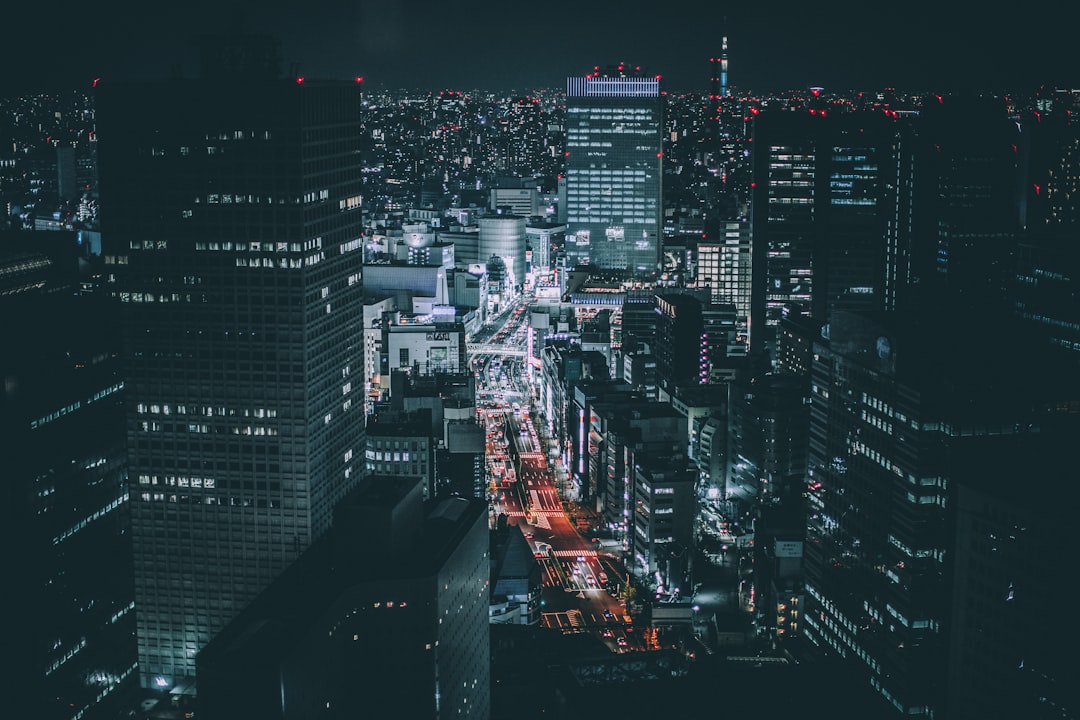
Digital twin technology has shown promise in speeding up construction of high-rise projects, notably in Singapore. This technology essentially creates a virtual replica of a building, allowing for detailed design work and continuous performance monitoring throughout the building's lifecycle. This approach puts Singapore in a leading position as it seeks to integrate digital tools into its city planning and infrastructure development. While initial successes have been seen, questions remain regarding the extent to which digital twin technology can improve things like energy efficiency and building performance. The upcoming Engineering Week 2025 will likely focus on how structural engineers can use artificial intelligence, including digital twins, to improve infrastructure projects. This event offers a platform for examining how this promising technology might mature further in the coming years.
Singapore's embrace of digital twin technology, particularly in a recent high-rise project, highlights the potential of this approach to accelerate construction. A review of relevant research suggests that digital twins are increasingly being explored for their role in virtual design and post-construction performance monitoring. Singapore's national-scale digital twin initiative, part of its Smart City and Industry 4.0 vision, is a strong indication of the city-state's commitment to innovative urban development.
This approach relies on a complex interplay of interconnected technologies. IoT devices, data integration techniques, machine learning algorithms, and real-time monitoring capabilities are essential components for a successful digital twin implementation. It seems that the initial application of digital twins has shown promise in construction, but there’s a sense that they haven't yet reached their full potential. It seems that using these technologies to improve things like energy efficiency in buildings still needs more development.
Singapore's early investment in a digital replica of the city, approximately SGD 73 million in 2014, underscores their foresight in recognizing the importance of digital technologies in construction and urban management. These developments are especially relevant for structural engineers, as digital twins can be invaluable tools for shaping the future of AI-integrated infrastructure projects.
The upcoming Engineering Week 2025 event, from February 16th to 22nd, is expected to delve into how structural engineers are at the forefront of exploring the use of digital twins and AI within infrastructure. It will be interesting to see what specific advances in the field are discussed. There's still a lot to learn about how to fully optimize these technologies and ensure that they truly enhance infrastructure development. It seems that there is a real need to carefully manage data integration and establish robust monitoring practices to realize the full promise of this technology. The integration of AI in construction is still in its early stages, and it will be critical to monitor its impact, particularly regarding decision-making processes and the human element in engineering.
Engineering Week 2025 How Structural Engineers Will Shape the Future of AI-Integrated Infrastructure from February 16-22 - Tokyo Team Creates Automated Material Testing Framework for Steel Structures
A team in Tokyo is developing an automated testing system specifically designed for steel structures in anticipation of Engineering Week 2025. This initiative, occurring from February 16th to the 22nd, highlights how artificial intelligence is poised to shape the future of infrastructure design. The framework, termed FrameRL, aims to improve the testing of steel structures through AI. Specifically, it uses a combination of Finite Element Method and Deep Reinforcement Learning to help optimize the testing process. While automation within design and testing is expected to reduce time and potentially improve accuracy, there are questions about how this change will impact the role of engineers and the overall quality of design decisions. It's still early days, and it's important to assess how the integration of AI into these critical areas will impact the final quality of infrastructure projects, as maintaining the balance between automation and human judgment will be crucial for safety and reliability.
A team in Tokyo is developing an automated framework for testing materials used in steel structures, a significant step towards improving efficiency within the field of structural engineering. This development, in line with the themes of Engineering Week 2025, uses robotics to automate tests that previously required significant human intervention, promising a dramatic reduction in testing time from weeks to just hours.
The framework incorporates a range of sensors that can accurately measure key mechanical properties of steel, including yield and tensile strength, along with elongation. These metrics are essential for confirming that the material meets stringent safety standards and guarantees the integrity of steel structures, be they buildings or bridges. By automating this process, the Tokyo team aims to improve the precision of tests and minimize human error, a critical step since misinterpretation of test results in structural engineering can have major safety implications.
The system's modular design allows for its implementation at various stages of a construction project. It's designed for ongoing monitoring and data collection, allowing for real-time analysis and validation of material properties. One of the more intriguing aspects is its AI component. This element can analyze historical data and potentially predict points of failure in steel structures, thereby shifting the emphasis from fixing problems after they appear to proactive risk management.
Furthermore, this automated system can help meet international standards by guaranteeing consistent and repeatable test procedures. This leads to better material usage within construction and potentially less material waste, as more accurate testing can allow engineers to make more precise decisions about what materials to use.
The developers incorporated machine learning into the framework, so the system can constantly learn from each test and improve its predictive capabilities. This continuous learning aspect suggests that the system will become increasingly reliable over time, potentially leading to major advancements in the accuracy of structural assessments.
While it's still early days, the Tokyo team's project has the potential to significantly reduce costs for construction firms. Faster, more accurate testing leads to reduced delays and better budget control. Ultimately, the work done in Tokyo illustrates a growing trend in engineering: adopting automation and AI to optimize established practices. This shift in approach might reshape how structural engineers test materials globally, providing a more efficient and scalable alternative to conventional methods. Whether this framework will become a broadly adopted standard remains to be seen, but it definitely represents a promising direction for the field.
Engineering Week 2025 How Structural Engineers Will Shape the Future of AI-Integrated Infrastructure from February 16-22 - California Bridge Project Uses AI Weather Data to Optimize Construction Schedule
A bridge project in California is employing AI-powered weather data to fine-tune its construction schedule, leading to better efficiency and faster project completion. By incorporating real-time and predicted weather information, likely through platforms like OpenWeatherMap, the construction team can adapt their plans to lessen the impact of disruptive weather. This is part of a broader movement toward integrating AI into infrastructure projects, showcasing how data-driven strategies are reshaping how things are built. It's not just about refining current methods; this also creates the groundwork for future advancements in civil engineering, making it a significant topic for the Engineering Week 2025 conversations on AI's role in infrastructure. As the field keeps developing, the value of AI in refining project management and tackling infrastructure hurdles will likely become more and more clear. There are always risks and uncertainties when implementing new technologies, but this project suggests some possible benefits of AI.
A bridge project in California is employing AI to analyze weather data and optimize its construction schedule. This approach seeks to improve efficiency and potentially shorten project timelines by proactively adjusting to changing weather conditions. The system is designed to analyze historical weather patterns alongside real-time data from various sources, allowing for more accurate predictions of potential delays. This suggests a promising shift in how infrastructure projects are managed, potentially reducing reliance on traditional, static scheduling approaches.
One intriguing aspect is the potential for this system to reduce the financial impacts of weather delays. By forecasting weather events, the project managers can better adjust labor allocation, material procurement, and overall project phases. This predictive ability could lead to a reduction in the need for costly contingencies designed to mitigate unforeseen weather issues. If this method proves effective, it could pave the way for a more nuanced approach to construction project management, especially in regions with unpredictable weather.
However, there are questions surrounding the breadth of data being integrated. While weather data is crucial, there are likely other project-specific factors that contribute to delays. Integrating a wider range of data, such as supplier performance or equipment availability, could provide a more holistic view and ultimately lead to more reliable predictions. Furthermore, the impact of this AI-driven approach on the existing workforce and project management teams requires careful consideration. Will this lead to more efficient workforce allocation or potentially displacement of workers in the long run?
The success of this approach is particularly important in the context of the nation's aging infrastructure and the need for efficient repairs and upgrades. This bridge project, if successful, could demonstrate the value of utilizing AI and weather data to create more resilient and adaptable construction practices. It could influence future infrastructure projects, potentially prompting broader adoption of similar AI-driven tools within the civil engineering field.
In the bigger picture, this California project highlights a trend we'll likely see more of: employing sophisticated technological tools to optimize construction processes. As we approach Engineering Week 2025, where the integration of AI and infrastructure will be explored in greater detail, the California Bridge Project offers an interesting case study to analyze. If proven successful, this project could establish a new standard for infrastructure development, showing how integrating AI into project management could enhance the safety, efficiency, and sustainability of our infrastructure.
Revolutionize structural engineering with AI-powered analysis and design. Transform blueprints into intelligent solutions in minutes. (Get started for free)
More Posts from aistructuralreview.com: