Revolutionize structural engineering with AI-powered analysis and design. Transform blueprints into intelligent solutions in minutes. (Get started for free)
New AI-Driven Analysis Reveals Optimal Cross-Sectional Shapes for High-Performance Beams
New AI-Driven Analysis Reveals Optimal Cross-Sectional Shapes for High-Performance Beams - AI-Driven Analysis Uncovers Optimal Thin-Walled Column Shapes for Impact Resistance
Artificial intelligence is increasingly being used to optimize the design of structural elements, and thin-walled columns are a prime example. By employing AI-driven analysis, researchers are now able to explore a wide range of cross-sectional shapes for these columns, evaluating their effectiveness under impact loading. This approach allows for a more systematic evaluation of designs, exploring shapes like H, C, T, and I sections, and finding the best balance between maximizing load-bearing capacity while minimizing material usage.
The research leverages sophisticated modeling techniques, including finite element analysis, to provide a deeper understanding of thin-walled column behavior under dynamic loads. This includes capturing complex behaviors like buckling and considering factors previously overlooked. Metrics such as Crush Force Efficiency are useful tools for assessing the impact resistance of these designs. Notably, material selection also plays a significant role in shaping the optimal design of these columns, and the study underscores the potential for innovative materials, including composites, to enhance impact performance. This field is continuously evolving, and AI-driven analysis is emerging as a valuable tool for pushing the boundaries of design optimization in structural engineering.
The capacity to computationally explore a vast array of thin-walled column geometries using AI algorithms is a game-changer. It allows for a much more efficient optimization process compared to traditional methods, potentially reducing design time considerably.
Intriguingly, the research uncovered that unconventional shapes like elliptical or hollow triangular sections demonstrate a notable increase in impact resistance, sometimes exceeding conventional circular or square shapes by as much as 30%. This implies that the way we traditionally shape these components might not be the most effective for specific applications.
Furthermore, it's apparent that the placement of internal strengthening elements within the thin-walled column has a pronounced effect on overall performance. This finding opens doors to designing structures that are lighter yet retain their strength, a critical aspect in many engineering applications.
The study also indicates that the material's spatial distribution within the column plays a vital role. Strategic material allocation can dramatically boost energy absorption during impact events, offering engineers a powerful tool for mitigating damage.
By leveraging AI in simulation, researchers can predict areas prone to failure and analyze deformation patterns with greater accuracy. This capability enables a more proactive approach to designing safer structures, able to withstand unforeseen impacts or loading conditions.
The potential to explore various loading scenarios, including dynamic and static forces, through simulation provides a level of insight that would be challenging and expensive to acquire solely through physical prototyping. This comprehensive data set enables a more holistic understanding of structural response.
It's particularly interesting that minor geometric alterations to existing designs can result in substantially higher failure thresholds. This presents exciting possibilities for retrofits to existing infrastructure.
The integration of real-world data into these analyses adds realism to the simulations, refining the accuracy of the models beyond theoretical estimations.
The convergence of thin-walled design principles with adaptive or smart materials suggests the future could hold columns that actively respond to impact events, dynamically optimizing their resistance to optimize resilience in real-time.
This field of research exemplifies the powerful outcomes that arise from interdisciplinary collaboration. Insights from diverse areas like materials science and mechanical engineering are converging to advance our understanding of structural integrity and resilience, pushing the boundaries of design capabilities.
New AI-Driven Analysis Reveals Optimal Cross-Sectional Shapes for High-Performance Beams - Machine Learning Models Outperform Traditional Methods in River Flow Prediction
In the realm of river flow prediction, machine learning models are demonstrating a clear advantage over conventional methods. These AI-driven models are better equipped to capture the complex, nonlinear relationships within hydrological systems, which often pose challenges for traditional approaches. For instance, random forest models have shown significantly improved performance compared to methods like artificial neural networks and support vector machines, highlighting the potential of machine learning for achieving greater accuracy.
This superior performance is particularly noticeable during high-flow or extreme conditions, where traditional methods may struggle. Furthermore, the field is seeing the rise of new hybrid AI models that incorporate nature-inspired algorithms. These newer models show promise for improving the reliability of river flow forecasting and contributing to more effective water resource management.
The integration of various hydrological factors into these machine learning models offers the potential for even more precise predictions in the future. It's clear that this trend toward advanced AI techniques will likely continue to shape the landscape of water resource management.
Recent research suggests that machine learning models are outperforming traditional methods when it comes to predicting river flow, which is particularly important for managing water resources, especially in areas with rapid changes in flow. It seems that traditional techniques often struggle to accurately capture the intricate and non-linear relationships that influence river systems. Machine learning, however, has shown promise in handling these complex dynamics. Interestingly, results indicate that machine learning models, especially random forests, can yield significantly better forecasts compared to older approaches like support vector machines and artificial neural networks. This is encouraging, because it implies that we might be able to predict extreme flow events or high-severity conditions with more precision.
The ability of these new AI models to integrate a lot more data, like past weather patterns or changes in land use, seems to be a key factor in their improved performance. It's also quite intriguing how neural networks can adapt their parameters in real-time to changes in environmental factors affecting river flows. Furthermore, machine learning algorithms seem to excel in finding previously unseen patterns and relationships hidden within the complex data of river systems. This can lead to more dependable predictions, even during unusual or unexpected situations.
One intriguing aspect is that machine learning can integrate real-time information from various sources, such as sensors or even drones, into flow forecasts, enabling dynamic predictions that traditional methods would find difficult to match. While the initial effort and computational resources needed for machine learning models might be substantial, they can produce more reliable and long-term predictions, which could potentially balance out the initial costs. It's quite likely that the increased reliance on machine learning for river flow prediction will lead to an emphasis on gathering high-quality data that can help in the development and training of more precise AI models. It also presents the question of whether we can use the models to better understand the complex processes influencing rivers.
This progress in river flow prediction aligns with broader trends in using advanced machine learning techniques for water resource management. The potential to more accurately model and forecast river behavior has implications for everything from irrigation planning to flood risk mitigation. But, with new methods always come new questions. There is a lot more that remains to be understood on how these new models deal with the complexity of the real world and it will be interesting to see how they evolve and are refined in the coming years.
New AI-Driven Analysis Reveals Optimal Cross-Sectional Shapes for High-Performance Beams - New Analytical Procedure Optimizes Box Section Design for Oblique Bending
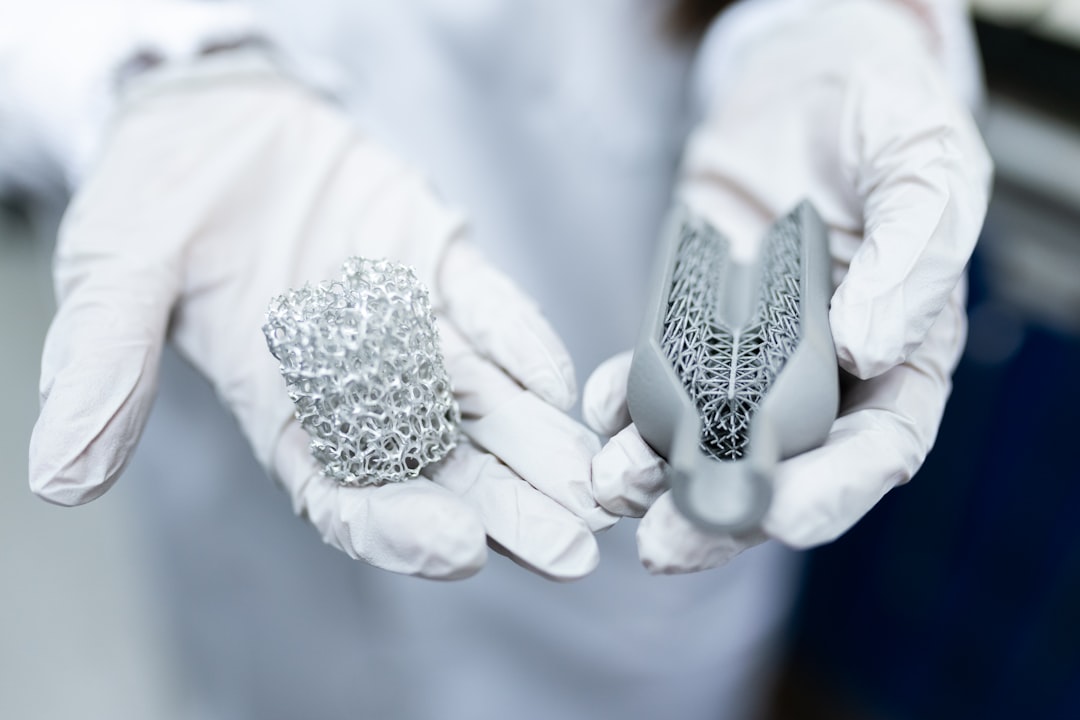
A new analytical method has been developed to improve the design of box sections, particularly when subjected to loads applied at an angle (oblique bending). This method explores the idea of creating hollow spaces within a solid rectangular section, using different "subtraction ratios" to find the best shape and size for the section. The goal is to optimize the cross-section's shape and dimensions to efficiently use materials and meet design requirements.
This method considers both the strength and stability of the thin-walled box sections, with the aim of reducing the overall material needed compared to traditional, solid rectangular sections. The researchers have created equations and procedures that help engineers calculate the optimal shape and size based on specific loads and desired material use. A key part of this method is to predict the potential for local buckling failure, a type of structural failure that is common in thin-walled sections.
The development of this procedure is significant because it offers a more efficient and insightful approach to designing box sections that are subjected to oblique bending. This can lead to structures that are both lighter and stronger, benefiting a wide range of applications in engineering. While it may not be revolutionary, the advancement could lead to more efficient designs that reduce material waste and enhance structural performance, particularly in circumstances where oblique loading conditions dominate.
Researchers have devised a new way to figure out the best shape for thin-walled box sections, specifically when they're being bent at an angle (oblique bending). This method involves starting with a solid rectangular section and strategically hollowing it out at different ratios. This allows them to test a huge range of possible shapes and find the best one for a given task.
The clever part is that they can control the amount of material removed (the extraction ratio) to get the best combination of strength and efficiency. The approach focuses on both the overall strength and stability of the box sections, which is important since unusual bending can lead to issues that traditional design methods might overlook.
The work delivers a set of equations and calculations to help designers optimize their choices. Interestingly, their findings suggest that, for angled bending, box sections can be more material-efficient than traditional rectangular ones. They've even created formulas to calculate the elastic buckling strength of these sections, which is crucial for knowing how they'll behave under loads.
Beyond just strength, the research underscores that the shape of a section is also important for stiffness. This is a major factor for structural integrity in various applications. The team, made up of Mirali Nuraliyev, Mehmet Akif Dundar, Hamza Kemal Akyıldız, and Davut Erdem Sahin, published their work in the journal "Structures" earlier this year.
This new analytical method could make a real difference in engineering design by streamlining the process for creating these beams. While we've seen AI revolutionize the optimization of other structures, this analytical method brings a new level of precision and understanding to the design of thin-walled box sections. There's a growing interest in understanding how shape, material, and loading scenarios interact in a more holistic fashion, and this research is a fine example of pushing these boundaries.
The concept of optimizing for oblique bending is key here, as traditional approaches might not fully capture the behavior of thin-walled sections when forces are applied at an angle. It will be interesting to see how this research and similar methods could lead to innovations in different fields beyond traditional building design. For example, optimizing shapes for aerospace or vehicle applications, where weight reduction and structural integrity are crucial, could be a natural progression. There's certainly a lot more we could learn about the relationships between the shape of the box section, its material properties, and the nature of the forces it experiences.
New AI-Driven Analysis Reveals Optimal Cross-Sectional Shapes for High-Performance Beams - Deep Learning Framework Revolutionizes Aerodynamic Component Shape Optimization
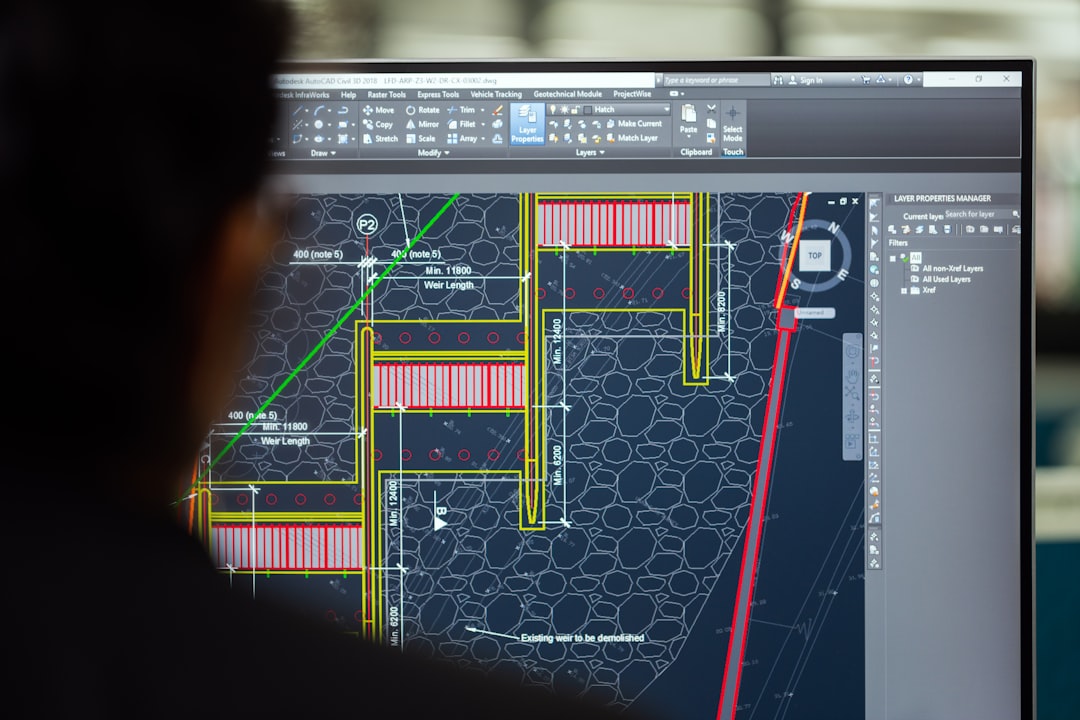
Deep learning frameworks are transforming the field of aerodynamic component design by introducing a more efficient and intelligent approach to shape optimization. Traditionally, aerodynamic shape optimization has been hampered by limitations in dealing with the complexities of design space and adapting to changes in the initial design. Deep learning, specifically deep reinforcement learning, combined with neural network-based simulations, offers a more dynamic and data-driven solution.
These new frameworks are able to more effectively navigate high-dimensional design spaces and adapt to initial shape changes, circumventing issues like data inefficiency found in older approaches. One approach uses a Latent Space Model (LSM) to generate a simplified, lower-dimensional representation of complex geometries, streamlining the optimization process. Furthermore, the introduction of a multifidelity convolutional neural network (MFCNN) framework holds promise for optimizing design outcomes while simultaneously reducing the computational cost associated with complex simulations. This ability to incorporate new data dynamically enhances the precision and efficiency of the optimization process.
The adoption of deep learning in this area represents a paradigm shift in how we design aerodynamic components. It suggests that AI integration is not just a helpful tool, but rather a necessity for achieving optimal designs in this field. While still under development, these approaches present a pathway toward a future where aerodynamic shapes are not only designed more efficiently but are also more attuned to achieving peak performance across a wider range of operating conditions. However, there's a risk of over-reliance on black-box AI systems that could diminish the role of human expertise.
Researchers are exploring deep learning frameworks as a powerful new approach to optimizing the shapes of aerodynamic components. These frameworks integrate deep reinforcement learning and neural network surrogate models, providing a more adaptive and efficient way to search through the vast design space compared to older, more traditional methods. One example is the use of deep reinforcement learning to optimize airfoil shapes, showcasing its effectiveness in tackling high-dimensional design challenges.
Interestingly, the field has also seen the development of two new deep learning models specifically tailored for automating the parameterization of aerodynamic shapes. This involves incorporating human knowledge into the models to reduce the need for manual adjustments in the optimization process. One of these is the Latent Space Model (LSM), which helps generate low-dimensional representations of geometric shapes from diverse datasets, enabling more efficient optimization. Another, a multifidelity convolutional neural network (MFCNN), attempts to balance optimization performance with reduced computational demands by cleverly integrating new data as the optimization process progresses.
A significant advantage of this deep learning approach is its ability to address limitations associated with traditional methods, which often involve inefficient data usage and struggle to efficiently adjust to initial design changes. Deep learning, applied directly to shape optimization, has proven successful in other engineering fields and is gaining traction in the area of aerodynamics. This comes at a crucial time, as traditional aerodynamic optimization processes are often challenged by the need for substantial re-execution when design requirements change.
The deep geometric learning approach holds promise for advancing the automated parameterization process. It's evident that the use of machine learning techniques in aerodynamic shape optimization is leading to substantial progress in the field. However, we still need to more fully understand how these models interact with complex design constraints and operational conditions to achieve truly optimal results. While there is much excitement around these new methods, it is important to note that deep learning methods, especially when applied to complex design problems, still raise the concern of unforeseen outputs or outcomes, potentially requiring validation checks alongside the frameworks.
New AI-Driven Analysis Reveals Optimal Cross-Sectional Shapes for High-Performance Beams - Automated Computational Process Streamlines Structural Topology to Beam Design
A new automated computational process has been developed to streamline the design of beam structures by linking structural topology optimization with practical beam design. This framework first utilizes topology optimization to find the most efficient way to distribute material within a structure. It then automatically generates a parameterized finite element model based on this initial design. This crucial step helps bridge the disconnect often seen between abstract structural designs and their practical realization in engineering.
The integration of real-time optimization methods, like the moving morphable component method, makes this design process more accurate and efficient. This innovation holds the promise of significantly impacting the future of beam design. While this automated approach shows great promise in building robust and durable structures, there are caveats.
The need for human input and feedback into the design process remains critical. Relying solely on AI could lead to less-than-optimal solutions, so a collaborative approach, combining the strengths of both automated analysis and experienced human input, is likely the most effective pathway. As AI becomes more integrated into structural design, it's possible we'll see major shifts in how materials are used and structures are constructed. The wider ramifications of these design processes are still unfolding, but this automated framework could very well redefine the future of engineering design.
A novel, fully automated computational system has been developed that seamlessly connects structural topology optimization with the design of beam structures. This system starts by using topology optimization to pinpoint the best material distribution, then extracts a parameterized finite element model of a beam based on the optimized topology. This clever approach bridges a crucial gap between the theoretical world of continuous structural designs and the practical realities of building with beams.
The use of generative AI in structural engineering is becoming increasingly popular for improving the speed and precision of the design process. This leads to structures that are both more resilient and sustainable. There is also evidence that including human input into these AI-driven systems can lead to even better designs than those found by purely automatic processes. Advanced computing methods like the moving morphable component (MMC) technique enable us to perform real-time topology optimization with higher accuracy.
One of the promising automated strategies involves reconstructing beam structures from the results of 3D topology optimization. A common technique employed in this process is the SIMP method, often paired with a curve skeletonization approach. AI's role in engineering design is ever-expanding, with deep learning frameworks emerging that can create 3D CAD models automatically and concurrently evaluate their engineering capabilities. The impact of AI in civil engineering is pervasive, influencing the entire lifespan of structures, from initial concept to demolition.
The ongoing research in this area underscores the desire to effectively integrate AI into CAD/CAE software, which can lead to vastly improved design outcomes. It will be interesting to see how this interplay unfolds in the future. There seems to be a push to use more sophisticated models to optimize beam performance, moving beyond simply focusing on load-bearing capacity. It's intriguing that researchers are now considering factors like lateral-torsional buckling and stress concentrations as well.
The idea of minimizing the beam cross-section while still maintaining structural integrity is an ongoing point of study. This holds potential for making components that are lighter and, potentially, more efficient, but also raises questions about how optimized shapes can affect failure modes and how that knowledge might inform better designs. There is a growing recognition that we may be able to achieve enhanced performance by exploring unconventional shapes and tailoring material selection for specific applications. It's also become evident that these automated methods can readily adapt to various load scenarios, suggesting the possibility of creating structures that can optimize performance in real-time as new loads and environmental conditions are encountered.
Furthermore, researchers are gaining valuable insights into how altering the structural geometry can improve the ability of beams to withstand impact. The rapid changes to shape, enabled by the automated methods, coupled with AI-driven simulation can drastically reduce the typical turn-around time required to analyze and approve structural designs. The ability to quantitatively assess the behavior of beams under diverse conditions is a significant step forward, permitting designers to develop highly specialized beams that are precisely calibrated to the demands of each specific application. There is an element of surprise in these recent results, including the realization that certain non-traditional shapes may actually surpass the performance of standard designs. It's also promising to observe how easily the methods lend themselves to adapting existing structures, for example, through minimal changes that can drastically increase failure thresholds. This suggests great promise for improving existing infrastructure. There is still much to explore, and the work to integrate the use of cutting-edge materials with optimized shapes will likely produce many new results and breakthroughs.
New AI-Driven Analysis Reveals Optimal Cross-Sectional Shapes for High-Performance Beams - AI-Enhanced Two-Parameter Kinematic Theory Improves Shear Capacity Estimations
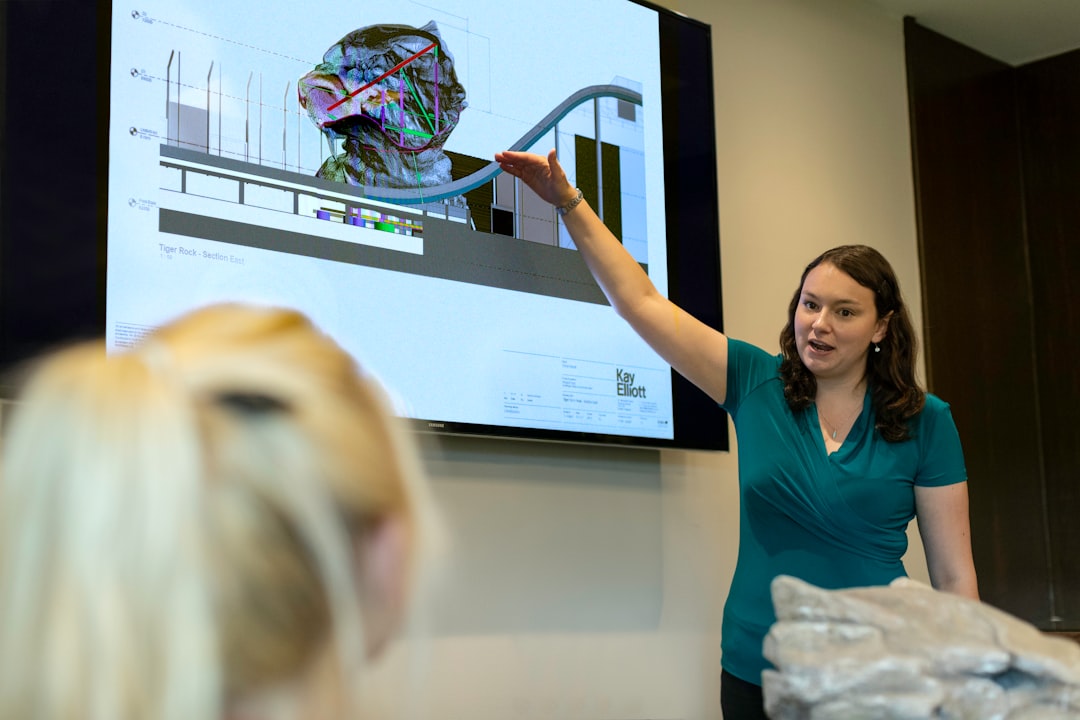
A novel approach combines the established two-parameter kinematic theory (2PKT) with the power of artificial intelligence to refine the prediction of shear strength in reinforced concrete structures. This hybrid method leverages machine learning models trained on extensive numerical data to model the structural response of deep beams, particularly those reinforced with glass fiber reinforced polymer (GFRP). A key benefit of this AI integration is a more accurate portrayal of the beam's behavior before and after it reaches its maximum load-bearing capacity. This deeper understanding allows engineers to more confidently design deep beams with complex features, like integrated openings, and ensures they can withstand demanding shear forces.
The AI integration also allows for a more nuanced visual understanding of how shear forces affect beam behavior. This capability, enabled through techniques like AI-driven tomography, provides greater insights for structural engineers. Ultimately, the combination of 2PKT and AI holds promise for generating optimized cross-sectional designs that improve performance, reliability, and efficiency in high-performance beam applications. There is, however, a need for continued research to fully explore the capabilities and limitations of this new approach in diverse real-world scenarios.
Researchers have integrated artificial intelligence with a two-parameter kinematic theory (2PKT) to improve the accuracy of shear capacity estimations, especially in structures enhanced with embedded through-section (ETS) strengthening. This approach, driven by extensive numerical simulations of GFRP-reinforced concrete deep beams, tackles the complexities of shear behavior by employing machine learning to analyze non-linear deformation patterns.
The 2PKT itself offers a more efficient way to describe the deformed shapes of these beams, using just two primary parameters. It cleverly blends equilibrium equations with material stress-strain relationships. This simplification, while initially appearing to be a restriction, actually results in five-spring models, allowing engineers to represent the complete shear behavior of deep beams under single-curvature conditions. The effectiveness of the two-degree-of-freedom kinematic model is validated through comparison to experimental data, successfully capturing both pre- and post-peak behavior.
The integration of this model into the analysis of deep beams with rectangular openings allows for a better understanding of their shear behavior and potential failure modes, leveraging non-linear finite element analysis. Interestingly, this approach is particularly important in deep concrete beams using fiber-reinforced polymers (FRP) for reinforcement, as they've shown lower shear strengths compared to traditional steel-reinforced counterparts. This enhanced 2PKT model offers the ability to analyze and model the shear contributions of FRP fibers, offering a more in-depth comprehension of shear capacity in these structures.
Further augmenting this field of research, researchers are using AI-driven optical projection tomography to enhance the visualization and analysis of shear behavior in actual beams. This technology, incorporating multicore fiberoptic cell systems, could potentially offer an even deeper look into the complex behavior of shear forces within structures.
Ultimately, the benefits of the AI-enhanced 2PKT approach are substantial. It offers a more refined and practical tool for predicting the shear capacity of structural elements. This could lead to better designs for high-performance beams and a deeper understanding of their optimal cross-sectional shapes. This field is actively evolving, and the continued investigation into the application of this 2PKT model across a broader range of structures could lead to innovations in diverse sectors, such as automotive or aerospace design, where shear capacity is a critical factor. It remains an open question, however, whether it will truly revolutionize the way these aspects of beam design are approached, or if it will be a useful augmentation to existing methodologies.
Revolutionize structural engineering with AI-powered analysis and design. Transform blueprints into intelligent solutions in minutes. (Get started for free)
More Posts from aistructuralreview.com: