Quantifying Splice Capacity New Methodologies for Structural Integrity Assessment
Quantifying Splice Capacity New Methodologies for Structural Integrity Assessment - Advancements in St.
Venant Torsional Shear Analysis for Box Section Splices
Recent work in St. Venant torsional shear analysis for box section splices has focused on improving how we understand the relationship between uniform and non-uniform torsion. New methods, such as finite-volume techniques, provide a refined way to analyze shear stresses, especially in situations where warping is restricted. This is particularly valuable for slender box sections because it helps to clarify the intricate interaction between St. Venant torsion and warping torsion.
Improvements in computational modeling are enabling researchers to better capture and define shear strains and stress distributions. This is crucial for designing more robust structures. Furthermore, the accuracy of these analyses is sensitive to the details of the box section's shape and the fineness of the computational mesh used in simulations. This highlights the need for carefully considering these aspects to ensure reliable results when assessing structural integrity.
St. Venant torsion theory, which traditionally assumes a uniformly distributed shear stress in thin-walled sections under free warping, has seen significant scrutiny recently, especially concerning its application to box section splices. The restriction of warping, a common occurrence in splices, introduces non-uniform torsion and, consequently, a more complex shear stress distribution that past methods often didn't capture adequately.
Researchers are now using sophisticated methods, such as finite-volume techniques, to explore how warping functions and boundary conditions impact the solution of St. Venant's torsion problem. This approach, coupled with the stress function methodology, enables a more precise calculation of shear stresses, accounting for both St. Venant and warping torsion. It's becoming clear that the interplay between these two types of torsion, particularly in slender box sections, plays a critical role in overall structural behavior under torsional loading.
We're also seeing progress in computational tools that allow for detailed examinations of torsional behavior in various scenarios. These include exploring the membrane analogy, a useful visualization tool for understanding torsion, and its application to both open and closed cross-sections. Furthermore, there's increasing interest in how composite materials react differently under torsion compared to homogeneous materials. The hope is that these detailed models can lead to a more accurate prediction of shear strains and stress distributions, ultimately improving the design and assessment of box section splices.
However, the accuracy of these analytical methods varies depending on the specific geometry of the section. Designing box and T-sections subject to torsional forces requires particularly careful consideration. Furthermore, the accuracy of finite element analyses heavily depends on the chosen mesh size when trying to determine torsion constants, especially for materials like precast concrete. Getting a handle on this relationship is crucial to achieving the necessary accuracy in predicting the torsional behavior of such structures. It’s becoming evident that as analysis techniques advance, the interaction of various factors like geometry and material properties needs to be closely scrutinized.
Quantifying Splice Capacity New Methodologies for Structural Integrity Assessment - Machine Learning Algorithms for Predicting Splice Performance Under Dynamic Loads
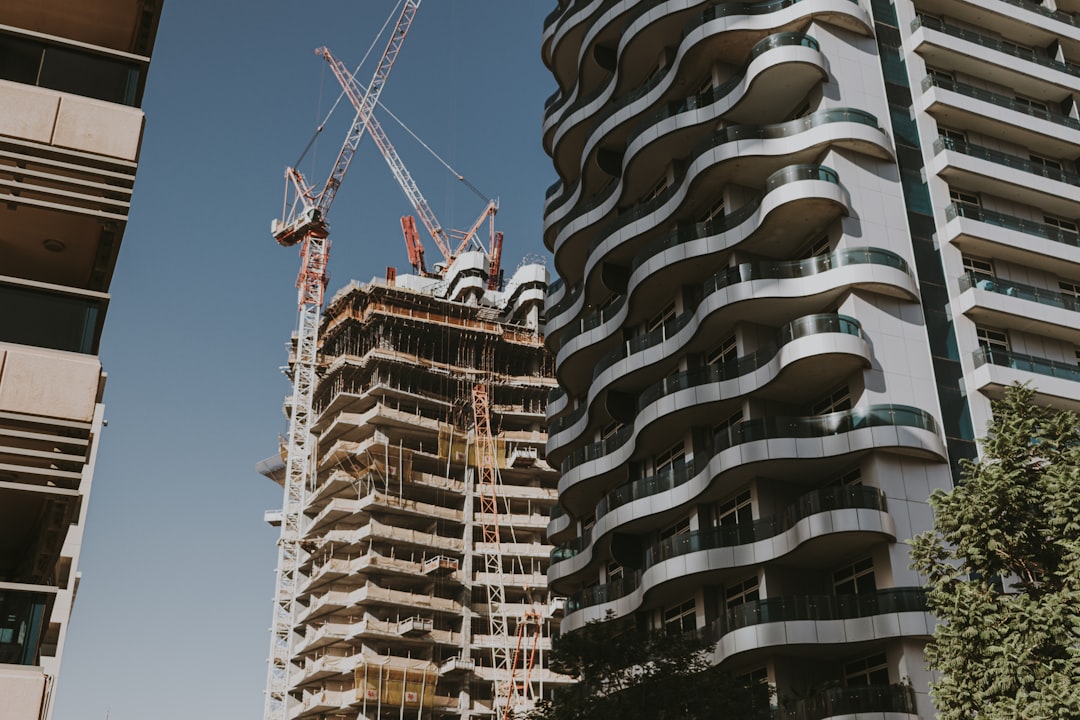
Predicting the behavior of structural splices under dynamic loads is crucial for ensuring structural integrity. While traditional methods, such as St. Venant torsion analysis, have provided valuable insights, they often face challenges in capturing the complexities of dynamic loading and the resulting splice performance. This has led to the development of machine learning algorithms, which show significant promise in improving our understanding and prediction of splice behavior under these demanding conditions.
The application of machine learning techniques, particularly when combined with established physics-based approaches, can refine our ability to model and analyze structural behavior during dynamic events. These algorithms can more effectively represent the complex interplay of material properties, geometry, and loading conditions, providing a more nuanced picture of how a splice reacts under dynamic stress. Furthermore, innovative approaches like graph neural networks (GNNs) are introducing new ways to model and predict the complete, time-varying response of a structure in a way that could fundamentally change how we approach this problem.
Machine learning algorithms, such as AdaBoost regression, have demonstrated remarkable capabilities in accurately predicting parameters like load-carrying capacity. This illustrates their practical value in structural design. Moreover, the inclusion of probabilistic methods within machine learning frameworks allows for a more refined assessment of uncertainty and variability inherent in the behavior of splices. This aspect is critical for safety assessments and robust design decisions. The integration of machine learning methodologies within the structural integrity assessment field is opening up new avenues for understanding and quantifying the performance of structural splices, ultimately leading to safer and more efficient structures. While still in its early stages, the field of machine learning holds immense potential for advancing structural design and integrity assessment.
Machine learning methods offer a promising avenue for exploring the intricate relationship between splice performance and dynamic loads. By sifting through extensive datasets of past splice behavior, these algorithms can identify subtle trends and correlations that traditional approaches might miss, potentially leading to more accurate predictions. Interestingly, combining multiple machine learning models through ensemble methods has proven particularly effective in improving the robustness of predictions, especially when dealing with the unpredictable nature of dynamic loads.
The careful selection of features – variables like material properties and load conditions – that go into these models is crucial. It highlights the importance of understanding what factors truly influence splice behavior. Moreover, some of these algorithms can handle the complexity of non-linear relationships within data, a feature often absent in more traditional linear analysis. This nuanced understanding of behavior can lead to more refined design guidelines.
One attractive aspect of machine learning is its adaptability. As operational conditions or material choices change, these models can be easily updated with new data, keeping predictions relevant over time. This ongoing refinement is important given the evolving nature of engineering materials and structural design. Furthermore, machine learning incorporates techniques like cross-validation, which can help mitigate the issue of overfitting – a scenario where models become overly tuned to specific training data, resulting in less generalizable insights.
The capacity of some machine learning methods to quantify uncertainty in input parameters is also noteworthy. This means engineers can better grasp the potential risks associated with splice performance under dynamic loads. Another intriguing possibility is the ability to supplement training datasets with simulations of various loading scenarios. This strategy could significantly reduce the reliance on physical testing, which can be costly and time-consuming.
However, it's essential to acknowledge the limitations. The quality of data fed into machine learning models directly impacts the accuracy of predictions. Robust and comprehensive datasets are essential for achieving reliable results. There's a growing interest in combining machine learning with traditional engineering principles to develop what are called hybrid models. This approach leverages the data-driven power of machine learning with the theoretical understanding of structural behavior, ultimately aiming to create even more accurate and robust predictive tools for the field of structural integrity.
Quantifying Splice Capacity New Methodologies for Structural Integrity Assessment - Novel Non-Destructive Testing Methods for Evaluating Splice Integrity in Aging Structures
The assessment of splice integrity in aging structures is increasingly important, especially as infrastructure ages and experiences the effects of environmental factors and material fatigue. Traditional methods often fall short in fully characterizing the complex degradation processes that occur in these structures. As a result, the development of new non-destructive testing (NDT) techniques is crucial for maintaining structural integrity and safety.
Novel approaches like advanced ultrasonic signal processing are enabling deeper insights into the condition of splices. This includes using techniques such as EMD correlation coefficient analysis, fuzzy entropy algorithms, and Hilbert transforms, which refine our ability to analyze composite materials. Coupled with this is a shift towards integrating automated and intelligent systems into NDT workflows. This leads to more efficient data collection and processing, potentially increasing the accuracy and speed of assessments.
The spectrum of NDT is also expanding beyond ultrasonic techniques. Acoustic emission and optical methods are emerging as valuable tools to probe the structural health of splices. These methods can provide complementary information that strengthens overall assessments and identifies potential failure mechanisms. In addition, these advanced inspection techniques often support the implementation of improved repair procedures and strategies for mitigating the risk of further deterioration.
It is becoming clear that machine learning techniques can play a significant role in enhancing the evaluation of splices. By analyzing massive datasets of structural behavior and splice performance under different conditions, these algorithms can build predictive models that enhance our understanding of how splices react to various loading scenarios. These tools may potentially reduce the need for costly and time-consuming experimental testing, allowing for more targeted and informed decision-making during structural inspections and maintenance.
While challenges remain, particularly in ensuring the quality and reliability of the input data, these NDT innovations represent a significant step towards creating more robust and reliable methods for ensuring structural integrity in aging structures. These developments pave the way for safer and more efficient approaches to evaluate and manage aging infrastructure.
New non-destructive testing (NDT) methods are being developed to assess the integrity of splices in aging structures, aiming to improve structural health monitoring. This is increasingly important as structures age, experience harsh conditions, and endure variable loads, which is a challenge globally. Advancements in ultrasonic signal processing, for example, using techniques like empirical mode decomposition (EMD) and the Hilbert transform, are increasing the depth at which we can assess composite structures with phased array methods.
While traditional techniques like ultrasound, acoustic emission, and optical methods have been used to evaluate structural health and repair effectiveness, the field is becoming more sophisticated. Automated inspection systems are increasingly integrated with NDT, improving the speed and accuracy of data processing. The push is to create inspection methods that are both safe and cost-effective while maintaining high standards of accuracy.
Understanding the microstructure of composite materials through optimized manufacturing processes is critical to evaluating their structural integrity. Non-destructive evaluation (NDE) research is pushing the boundaries, exploring both traditional and emerging technologies for structural assessment. Real-world studies have shown that these new methods are successful in damage assessment and evaluating the efficacy of repair efforts in aging structures.
Continual developments in NDT are crucial for the accurate assessment of splice capacity, providing a more comprehensive evaluation to ensure overall structural integrity. This involves exploring new technologies to detect subtle defects and monitoring structural health over time. There is also a strong desire to push beyond simple defect identification and move towards truly predictive maintenance, where machine learning might potentially forecast future issues based on current NDT data.
This is a rapidly evolving area with potential to redefine how we inspect and maintain infrastructure. There's a growing recognition that understanding the detailed behavior of structures under stress, even with highly specialized analytical methods, may not always be sufficient. The ability to detect subtle changes and variations, and then accurately link those with possible future performance issues, might be the next frontier in NDT. It's fascinating how the ability to non-invasively probe the interior of structures could lead to a fundamentally different understanding of how they age and fail, and thereby how we can maintain them more effectively in the future.
Quantifying Splice Capacity New Methodologies for Structural Integrity Assessment - Implementing Augmented Reality for Real-Time Splice Capacity Assessment in the Field
Augmented reality (AR) offers a new way to assess the capacity of structural splices in real-time during field inspections, improving how we evaluate structural integrity. AR's ability to overlay digital information onto the physical world allows inspectors to intuitively measure structural deformation and assess damage more readily. This is especially valuable for evaluating structures after seismic events, where rapid damage assessments are crucial. By combining AR with tools like 5G networks, we can facilitate real-time data sharing and remote collaboration between field personnel and engineers, addressing challenges in traditional inspection methods that often involve delayed data processing and difficulty in visualizing complex structural details. In a world with increasing concerns about the integrity of aging infrastructure, AR holds significant potential to enhance structural health monitoring processes, leading to more timely and reliable assessments. While still emerging, the potential for AR to revolutionize structural inspections and improve structural safety appears strong. There are questions about how accurate these visualizations are, the role of human interpretation in the AR generated data, and the cost-effectiveness of AR applications, but they represent a promising area of innovation for the field.
Augmented reality (AR) presents a promising avenue for real-time splice capacity assessment in the field. By overlaying digital information onto the real-world environment, AR can provide engineers with immediate access to sensor data, allowing for on-the-spot analysis of splice conditions. This direct link to information reduces the delays often associated with traditional data collection and processing.
We can envision AR being particularly helpful for visualizing complex stress distributions within a splice. Instead of relying on static 2D representations, AR could create interactive 3D visualizations, giving engineers a more intuitive grasp of potential failure points. This visual enhancement could significantly improve the understanding of the stresses within the splice, aspects that might be difficult to capture with traditional methods.
The training potential of AR is another intriguing aspect. AR interfaces could serve as interactive training tools, guiding less experienced engineers during on-site inspections. This could be a powerful way to enhance competency in structural assessment, particularly in cases where understanding complex failure modes or splice behavior is critical.
Beyond training, AR can enhance decision-making in the field. By providing context-rich information through overlays, engineers can swiftly evaluate a splice's condition and make informed decisions about necessary actions. This is important when engineers face critical decisions relating to structural integrity.
AR also holds the potential to connect theoretical models with the physical reality of structures. We could see AR being used to overlay digital models of stresses or failure modes onto real-world splices, thus bridging the gap between abstract concepts and actual conditions. This type of visualization could significantly improve our ability to teach and understand how a splice functions or fails.
Moreover, AR could be effectively integrated with existing machine learning tools that are designed to predict splice performance. This means the AR-equipped inspector could view both the actual splice conditions as well as the predictions of machine learning algorithms, providing a point of comparison that could refine inspection and maintenance decisions.
Further, AR, when coupled with suitable hardware and software, could offer enhanced precision in measurements of splice geometries and conditions. This increased accuracy could translate into more reliable assessments of splice capacity and health.
The remote collaboration potential of AR is noteworthy. Engineers in remote locations could interact with field personnel, guiding them through complex inspections using AR overlays. This could allow for a more efficient use of expert knowledge and a greater degree of shared understanding in the assessment of critical structures.
Beyond this, the ability to integrate AR with automated inspection systems offers the potential to further streamline the assessment process. The idea is that automated inspection could collect the data, and the AR overlay would present this in an intuitive and informative way.
Despite these exciting possibilities, there are challenges to overcome before AR becomes widely adopted for this purpose. The development of robust mobile computing platforms in the field and maintaining data accuracy and reliability in challenging environments are critical issues. Moreover, the long-term cost-benefit analysis of implementing AR, including both capital and maintenance costs, is still being studied.
It's still early days for AR in this application, but the potential benefits are compelling. This technology has the ability to change how we collect and interpret data about splice capacity in the field, leading to more informed decision-making and improved structural integrity assessments.
Quantifying Splice Capacity New Methodologies for Structural Integrity Assessment - Quantum Computing Applications in Modeling Complex Splice Behavior
Quantum computing presents a novel approach to modeling the complex behavior of structural splices, particularly in scenarios involving intricate interactions between various components. Utilizing the principles of quantum mechanics, specifically quantum bits (qubits), allows for simulations that can more effectively capture the behavior of these many-body systems, often found in the mechanics of structural connections under load. While still in its early stages, noisy intermediate-scale quantum (NISQ) technology shows promise for enhancing our ability to model complex splice behavior under dynamic loads, where traditional computational methods may struggle. This has the potential to significantly enhance structural integrity assessments, particularly when predicting splice performance under difficult circumstances. The increased computational power of quantum computing offers the potential to redefine how we approach quantifying splice capacity, although significant hurdles still need to be addressed in terms of data quality and algorithm efficiency. The ongoing research and development of quantum computing applications, however, suggests that it holds a potentially significant role in future structural integrity analysis methodologies.
Quantum computing, with its unique capabilities, presents exciting possibilities for improving our understanding and analysis of complex splice behavior. The potential for a "quantum speedup" in computationally intensive tasks related to splice modeling is particularly intriguing. For instance, we could see significant reductions in the time it takes to run large finite element analyses, which are often a bottleneck in traditional structural assessments.
Unlike classical computers that rely on bits, quantum computers leverage qubits, which can exist in multiple states simultaneously. This allows for the simulation of materials at the atomic level, a capability that could revolutionize how we model the behavior of materials under stress, including the shear stresses prevalent in torsional loading of splices. Moreover, the concept of quantum entanglement – a phenomenon where two or more qubits become linked – offers the potential to model intricate relationships between structural variables, potentially revealing new insights into splice interactions that were previously difficult or impossible to study.
Quantum computing also holds promise for efficiently handling the complex geometric shapes and transformations relevant to splice configurations. This includes the ability to dynamically model how splice geometries change under load, an area that classical simulations often struggle to capture accurately. Integrating quantum machine learning with existing structural models might further improve the accuracy of predictions about splice performance, especially under dynamic loads, by revealing previously hidden patterns in the data.
One of the key advantages of quantum computing is its potential to more effectively quantify uncertainties in splice behavior models. This could lead to better risk management strategies in design and maintenance practices, by providing more precise confidence intervals for splice capacity. Further, the potential for real-time data processing using advanced quantum networking technologies could significantly change how we assess structural integrity, particularly after dynamic events such as earthquakes.
Quantum algorithms could also be used to augment NDT methods, leading to faster processing and analysis of the massive datasets collected during inspections. This could facilitate the identification of subtle spatial variations in splice integrity, which is particularly crucial for monitoring the health of aging structures. Additionally, quantum annealers could find applications in optimization problems relevant to the design of splice components, potentially enabling us to more effectively optimize aspects like material selection and geometry to improve resilience.
Finally, the introduction of quantum algorithms could pave the way for entirely novel structural models that go beyond the approximations often used in classical approaches. This may involve more accurately representing non-linear material behavior and complicated load distributions in splices.
While quantum computing faces its own set of challenges and is still in its early stages of development, the potential for applying it to structural integrity assessment, and specifically to modeling splice behavior, is undeniably intriguing. The possibility of obtaining a deeper understanding of splice performance under a wider variety of load scenarios promises to advance the field in innovative and exciting ways.
Quantifying Splice Capacity New Methodologies for Structural Integrity Assessment - Integration of Nanosensors for Continuous Monitoring of Splice Structural Health
Integrating nanosensors into structural health monitoring (SHM) systems offers a new way to continuously track the condition of splices. This continuous, ongoing monitoring provides a wealth of data that can be used to understand how a splice is performing over time and allows for early detection of problems. The different types of nanosensors available, with their unique ways of working and how they are made, are helping to improve how we evaluate structural integrity.
This approach helps create "smarter" materials and structures by allowing for both detailed, local assessments and broader, system-wide evaluations. As we move forward, there are important factors to consider. Understanding how these sensors influence the materials they are embedded in is critical, as is making sure the entire SHM system is working as intended. However, the potential benefits are compelling: less reliance on traditional inspections and potentially lower overall maintenance costs. It's clear that embracing nanosensors represents a significant step forward in proactively managing the health and safety of infrastructure and structures.
Nanosensors are showing great promise in detecting and assessing the structural health of bridges, providing a more proactive approach to structural health monitoring (SHM) compared to traditional methods. Their integration allows for a continuous stream of data about structural integrity, creating a new generation of smarter structures and materials. This is particularly exciting because the various types of nanosensors, which employ diverse operating principles and fabrication techniques, are pushing the boundaries of structural integrity assessment.
The field of SHM, with its focus on minimizing the need for routine inspections and reducing maintenance expenses, has become very important. This is made possible by dividing SHM techniques into local and global approaches, based on their areas of application, enhancing the precision of damage detection. However, implementing these nanosensors successfully requires careful consideration of the integration methodology and its potential impacts on the mechanical behavior of the host materials.
Embedded sensors (ESs) are central to SHM systems, continuously monitoring key material properties that are vital for effective condition management. Recent advancements in nanotechnology have significantly improved the capabilities and functionalities of these sensors, leading to refined assessments of structural health.
The continuous monitoring facilitated by nanosensors allows for real-time evaluation of structural health. This enables engineers to make informed decisions to enhance the safety and extend the lifespan of infrastructure. However, concerns regarding sensor accuracy and long-term reliability remain areas of active research, as does the need to understand how sensor data can be efficiently interpreted to improve decision-making and maintenance strategies. The question of how the size and type of sensor affect the structure itself is also a continuing area of study. Nonetheless, the potential for nanosensors to revolutionize how we monitor and maintain bridges is undeniable, particularly for complex connections like splices where stress concentrations are a concern.
More Posts from aistructuralreview.com: