Soil Health Monitoring How AI and Structural Analysis Are Revolutionizing Precision Agriculture
Soil Health Monitoring How AI and Structural Analysis Are Revolutionizing Precision Agriculture - AI-Powered Soil Sensors Detect Micronutrient Deficiencies in Real-Time
Artificial intelligence is increasingly integrated into soil sensing technologies, offering real-time insights into soil nutrient levels and plant health within agricultural settings. These AI-driven sensors continuously gather data, which is then analyzed by sophisticated algorithms to pinpoint instances of micronutrient deficiencies and broader nutrient imbalances that can negatively impact crop production. By connecting with other technologies like drones and satellite imaging, these systems allow for a comprehensive view of environmental factors and crop growth trends. This translates to better decision-making in agriculture. While these sensors have shown potential in adapting to diverse soil and plant types, highlighting their contribution to sustainable farming practices, further long-term studies are essential to evaluate their widespread use across varying agricultural contexts. It's clear that as soil monitoring techniques advance, AI's role in ensuring precision and efficiency in farm management will continue to grow.
AI-powered soil sensors are pushing the boundaries of nutrient monitoring by offering unprecedented levels of detail. They can pinpoint micronutrient concentrations down to a few parts per million, allowing us to detect subtle deficiencies that traditional methods might miss. This precision is made possible by combining electrical conductivity measurements with spectral analysis, effectively providing a comprehensive picture of the soil's micronutrient profile without relying solely on lab tests, which can be costly and time-consuming.
The importance of monitoring these micronutrients is clear. Studies have shown that even small shortages of key elements like zinc and iron can significantly impact crop yields – potentially leading to reductions of 30% or more. Real-time monitoring with these sensors therefore becomes crucial for maximizing productivity and ensuring optimal plant health.
One of the key features of these sensors is their ability to operate autonomously and deliver a constant stream of data. This continuous flow of information empowers farmers to react swiftly to changing soil conditions, whether it's implementing fertilization strategies or making decisions about crop rotations. Furthermore, the AI algorithms behind these systems analyze the data to identify potential nutrient deficiencies before they become major problems, using historical trends to predict future needs. This predictive capacity promotes a proactive approach to management instead of constantly playing catch-up to address existing issues.
The potential for resource optimization through the use of these AI-driven systems is quite exciting. Several studies have shown that using AI-powered sensors can decrease fertilizer use by up to 25%. This reduction in fertilizer application not only decreases the environmental impact of agriculture but also helps farmers to optimize nutrient input for maximum crop growth without over-applying.
The high sampling frequency provided by these sensors allows for deeper insights into how nutrient availability in the soil changes over time. By capturing these fluctuations, we can develop a more nuanced understanding of soil dynamics than with traditional methods that often only provide static snapshots of soil conditions.
Additionally, combining sensor data with satellite imagery enables us to pinpoint nutrient deficiencies across a whole field, allowing farmers to apply treatments where they are needed, rather than employing a one-size-fits-all approach. This targeted approach enhances the effectiveness of interventions while minimizing resource waste.
Integrating sensor data with established agricultural models can improve the accuracy of yield predictions. Farmers can gain a clearer picture of expected yields and make better-informed decisions about planning and resource allocation based on these predictions.
The encouraging trend of decreasing sensor costs is broadening access to these technologies, potentially enabling smaller farms and growers to implement precision agriculture practices. This development has the potential to level the playing field and enhance agricultural practices across the board.
Soil Health Monitoring How AI and Structural Analysis Are Revolutionizing Precision Agriculture - Machine Learning Models Predict Crop Yields with 95% Accuracy
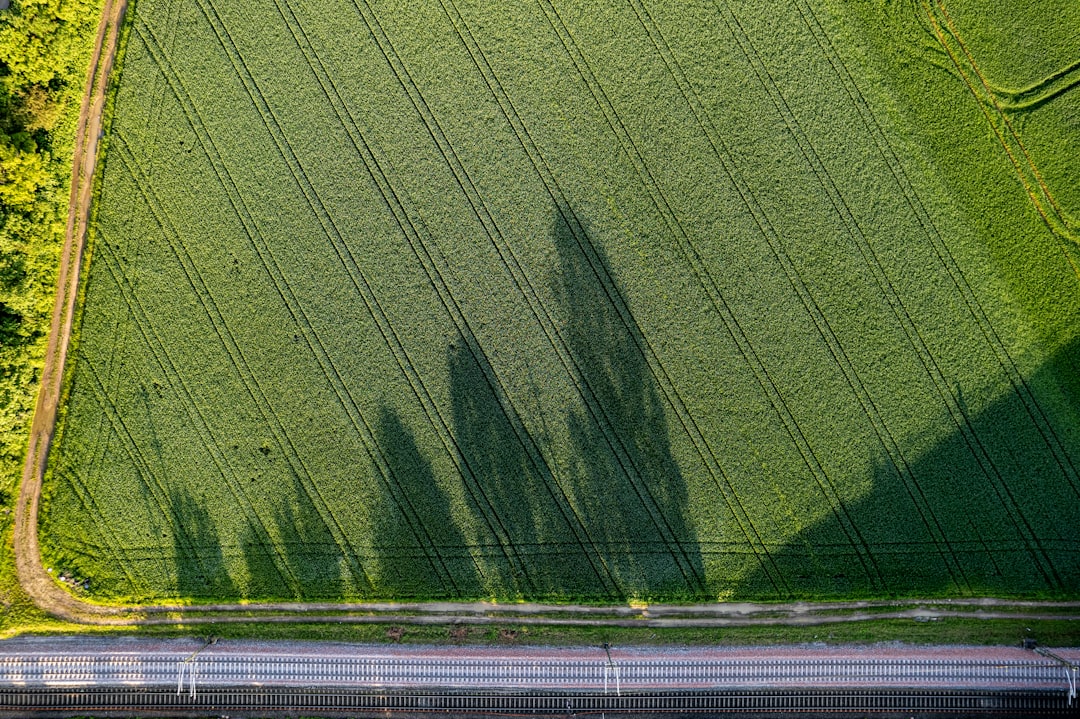
Machine learning models are demonstrating a remarkable ability to predict crop yields, achieving accuracy rates as high as 95%. These models primarily utilize decision tree algorithms, like Random Forests and XGBoost, to analyze a multitude of factors, including soil health, moisture, and fertility levels. The integration of real-time sensor data further strengthens these predictions, providing farmers with a more precise understanding of crop suitability and optimizing resource allocation. This increased predictive power is a key development in the evolution of precision agriculture, holding significant potential for enhancing food production and ensuring food security in a rapidly changing world. However, it's important to recognize that the success of these machine learning models hinges critically on the quality and relevance of the data they're trained on. The reliability and consistency of data collected from sensors and other sources will be crucial to maximizing the accuracy and applicability of these predictions across diverse farming contexts.
Machine learning models are demonstrating impressive capabilities in predicting crop yields, achieving accuracy rates as high as 95% in some cases. These models leverage substantial historical datasets encompassing weather patterns, soil properties, and past crop performance, enabling algorithms to uncover intricate relationships among variables that might escape human observation. However, it's important to recognize that their predictive power isn't universally consistent. Performance varies across different crops and geographic locations, necessitating continuous adjustments and refinements to ensure predictions remain relevant to specific conditions.
Recent progress in deep learning has paved the way for neural networks that can analyze multi-faceted data from diverse sources, such as sensor readings and satellite imagery, offering a more comprehensive grasp of the factors influencing crop growth. The incorporation of real-time data into these models has further enhanced their predictive capabilities. As new information streams in, the models dynamically adapt and refine yield estimates to reflect immediate shifts in environmental conditions.
Interestingly, the application of machine learning has unveiled unexpected correlations that shed light on the subtle influences on yields. For example, certain soil microbial communities and their effects on nutrient uptake, previously overlooked by conventional agricultural models, are now becoming more apparent. This deeper understanding can potentially lead to more targeted management practices.
The adoption of predictive models has the potential to boost farmers' profitability by facilitating more precise planting, irrigation, and fertilization strategies, ultimately contributing to reduced waste and optimal resource allocation. The implications extend beyond the farm, influencing supply chain management. By anticipating potential yield fluctuations, stakeholders across the agricultural sector, from producers to distributors, can better prepare for changes in demand and refine their strategies accordingly.
Some models employ ensemble techniques, combining forecasts from multiple algorithms to bolster overall accuracy, highlighting the importance of model diversity in attaining dependable results. Despite their demonstrated promise, these models are not without their limitations. They can be prone to overfitting, a situation where a model excels at predicting outcomes based on its training data but falters when confronted with new, unseen data. This potential for overfitting necessitates cautious application of these models in practical field scenarios.
Furthermore, continuous validation of the models against actual yield data is crucial. Agricultural conditions are in a constant state of change, and models must be routinely updated to maintain their reliability as new challenges and environmental conditions emerge. Ongoing refinement and adaptation are essential to ensuring that the insights gleaned from these models remain relevant and beneficial in the dynamic environment of modern agriculture.
Soil Health Monitoring How AI and Structural Analysis Are Revolutionizing Precision Agriculture - Drone-Based Imaging Systems Map Soil Organic Matter Content Across Fields
Drones equipped with specialized cameras are increasingly used to map the distribution of soil organic matter (SOM) across fields. These aerial platforms utilize advanced imaging techniques to capture high-resolution data, providing a detailed view of SOM content that's not easily achieved through conventional, ground-based methods. The ability to obtain this detailed view over wide areas in real-time offers a more comprehensive understanding of SOM variability across a field. This has implications for improving soil management and decision-making for sustainable farming.
While traditional approaches to soil analysis can be infrequent and limited in scope, drone technology allows for more frequent and spatially detailed assessments. However, the utility of drone-based imaging hinges heavily on the accurate interpretation of the captured data. Fortunately, artificial intelligence is playing a crucial role in refining the analysis of this data, enabling the creation of more precise SOM maps. By integrating AI into the process, we can generate better insights for guiding soil health practices.
The potential benefits of these technologies extend across various aspects of farming. The improved understanding of SOM variation across a field enables farmers to optimize nutrient management, tailor irrigation techniques, and even adjust tillage practices for improved soil health. While promising, further research is needed to fully understand the intricacies of soil-vegetation interactions, as well as the long-term impacts of applying this knowledge in diverse farming contexts. Nevertheless, the development and refinement of drone-based imaging systems with AI-driven analysis represents a significant step towards a more informed and precise approach to managing soil health, which ultimately contributes to the advancements in the field of precision agriculture.
Soil organic matter (SOM) is a fundamental indicator of soil health and nutrient availability. Accurately mapping its distribution across fields is crucial for optimizing soil management and agricultural practices. Drone-based imaging systems, using advanced camera technologies, are emerging as a valuable tool for achieving this goal. These unmanned aerial vehicles (UAVs) capture high-resolution images that allow for detailed mapping of SOM content, providing information that's difficult to obtain using traditional ground-based methods.
The ability of drones to capture a wide spectrum of light reflected from the soil surface is particularly useful. By analyzing the light signatures, we can effectively estimate the concentration of SOM without the need for extensive, and potentially disruptive, soil sampling. This spectral analysis, a core function of hyperspectral cameras frequently incorporated into drone systems, has opened up new possibilities in areas like precision agriculture, digital soil mapping, and environmental monitoring.
While drones offer rapid data collection over large areas, allowing us to get a quick overview of SOM variation, the resulting data requires careful interpretation. It's not always a straightforward process. Factors like crop type, shadows cast by plants, or the inherent variability of soil itself can influence the accuracy of the resulting maps. Furthermore, although drone technology is becoming more accessible, the cost of specialized hyperspectral cameras and data processing capabilities can still pose a barrier for some farmers.
Despite these considerations, the integration of drone imagery with other data sources offers great potential for advancing precision agriculture. When combined with data from AI-powered soil sensors or weather stations, we can obtain a more comprehensive picture of soil health. Machine learning can play a vital role in further analyzing the drone data, revealing previously hidden correlations and patterns. This could lead to more tailored soil management plans, optimizing fertilization or irrigation practices based on specific locations within a field.
One of the primary advantages of drone-based imaging is its non-destructive nature. Unlike traditional methods that require soil core samples, drone imagery allows us to assess SOM without physically disturbing the soil. This preserves the soil's structure and natural processes, which is particularly important for long-term soil health. Moreover, by collecting data at regular intervals, farmers can track SOM trends over time, enabling a more nuanced understanding of the impact of various agricultural practices on the soil ecosystem. However, ongoing validation against traditional ground-based sampling is still important for maintaining the reliability of these new techniques.
Although still evolving, drone-based imaging systems show great promise for improving our ability to monitor and manage soil health. It's a valuable addition to the suite of tools available for enhancing agricultural sustainability and optimizing resource use. As the technology matures and becomes more accessible, the potential for transformative changes in agricultural practices seems certain.
Soil Health Monitoring How AI and Structural Analysis Are Revolutionizing Precision Agriculture - Structural Analysis Algorithms Identify Early Signs of Soil Erosion
Algorithms designed for structural analysis are finding new applications in the early detection of soil erosion, a significant threat to long-term soil health. Given the detrimental impact of soil erosion on agricultural productivity and food security, the ability to identify erosion at its onset is crucial. Researchers are employing machine learning methods to create models that forecast the rate at which water causes erosion, giving us a better understanding of the underlying factors contributing to soil loss. These algorithms empower proactive management approaches by allowing farmers to intervene early and address emerging erosion problems, preventing further damage and optimizing farm practices. The incorporation of these sophisticated algorithms is a pivotal development in the effort to build more resilient and sustainable agricultural systems, especially as climate change and other factors are putting strain on soil resources. While promising, it's important to continually evaluate the performance of these algorithms in real-world environments and in diverse agricultural contexts.
Structural analysis algorithms are increasingly being used to not just identify the early signs of soil erosion, but also to monitor its evolution over time. This continuous monitoring gives us a more dynamic understanding of how soil stability is impacted by rainfall, land use, and other factors. It's fascinating to see how these algorithms can bring together information from various sources like moisture sensors and thermal imaging to create a comprehensive picture of soil health, moving beyond basic erosion metrics.
One promising application of this technology is predictive maintenance for agricultural practices. By analyzing current soil conditions and historical erosion patterns, these algorithms can forecast future risks, allowing farmers to intervene early and implement preventative strategies. This approach is significantly more proactive than the traditional methods of responding to visible damage. Furthermore, the scale at which these algorithms operate is quite remarkable—from small experimental plots to vast agricultural landscapes. This flexibility makes it possible to customize erosion control strategies for a particular farm or even a specific region.
It's important to recognize that soil erosion exhibits significant variation, even across small areas due to variations in topography. These structural analysis tools address this spatial variability, allowing for very precise interventions to control erosion, something that traditional methods often fail to achieve. We can then measure how effective these interventions are—whether it's cover cropping or contour farming—making it possible to choose the best approach for a particular situation. The algorithms can also analyze historical erosion trends, offering valuable insights into long-term soil health and the potential impacts of future land management practices.
The real-time feedback provided by these algorithms enables rapid adjustments to farm management strategies. Instead of waiting for periodic soil assessments, farmers can react to changing conditions immediately, leading to much more effective soil conservation. Additionally, the algorithms can perform cost-benefit analyses for various erosion control options, optimizing resource allocation and maximizing returns on investment.
Recent advances in machine learning are continuously enhancing these algorithms, allowing them to learn from new data and adapt to evolving environmental conditions or shifting agricultural practices. This ability to learn and improve is crucial in maintaining their accuracy and relevance in a constantly changing environment. While still an active area of research, it appears these structural analysis techniques hold enormous potential for revolutionizing how we manage and conserve our soils, ensuring the sustainability of agriculture for generations to come.
Soil Health Monitoring How AI and Structural Analysis Are Revolutionizing Precision Agriculture - IoT Devices Monitor Soil Moisture Levels and Automate Irrigation Systems
IoT devices are increasingly important in modern farming by providing real-time insights into soil moisture and automating irrigation. These devices use soil moisture sensors to measure water levels and relay this information to control systems for irrigation. The integration of IoT with AI enables farmers to use current moisture, weather, and plant needs to make more precise irrigation decisions. This allows for the optimized use of water while boosting crop productivity. While the potential benefits of automated irrigation are substantial, there are still hurdles to overcome like ensuring the accuracy of the sensor data and the seamless integration of different systems. Therefore, continued research and development of these technologies are crucial to ensure their effectiveness across a variety of farming environments.
IoT devices are increasingly used in agriculture to monitor soil moisture levels, offering a more precise and automated approach to irrigation compared to traditional methods. These devices typically utilize sensors buried within the root zones of plants, employing techniques like capacitive and resistive sensing to measure moisture with millimeter precision. This level of detail surpasses what's achievable with traditional hand-held meters, which provide only intermittent and less accurate readings.
The integration of IoT sensors into irrigation systems allows for automatic adjustments to watering schedules based on real-time moisture levels. This automation optimizes water usage and minimizes waste, with the potential to reduce water consumption by up to 50% compared to conventional irrigation practices. However, it's important to recognize that the effectiveness of these systems can vary based on factors like soil texture and crop type.
Furthermore, IoT devices provide farmers with immediate access to soil moisture data via dashboards or mobile apps. This capability allows for swift adaptation to changing conditions, such as unexpected rainfall or rapid changes in temperature. The ability to see and respond to these events in real-time minimizes over-irrigation and potentially reduces stress on irrigation infrastructure. However, the usefulness of these systems is contingent on reliable data connectivity and the accuracy of the sensor data.
It's also worth considering that different soil types exhibit different water retention capabilities. Sandy soils, for instance, tend to drain quickly, while clay soils retain moisture more effectively. IoT sensors can help farmers understand how various soil textures influence moisture levels, allowing them to develop more tailored irrigation strategies for different crops. While this appears advantageous, it also increases the complexity of the system and requires the user to have a good understanding of their soil.
The combination of soil moisture readings with weather forecasts is another useful application of IoT technology. By predicting optimal irrigation times based on anticipated weather conditions, these systems can mitigate the risks of unpredictable events like drought or sudden rainstorms. However, it's crucial to remember that weather forecasting has limitations and might not always be reliable enough to make decisions solely on this type of information.
The continuous monitoring capabilities of IoT devices lead to the creation of substantial historical datasets. This wealth of information allows for detailed analysis of how soil moisture patterns correlate with crop yields. Such analyses can then inform future planting decisions and irrigation schedules. However, the proper interpretation of these complex datasets and ensuring the representativeness of the data will be critical to obtaining valid insights.
Moreover, some advanced IoT soil moisture sensors are capable of measuring soil compaction. This information provides insights into how soil structure influences water retention and helps inform practices aimed at mitigating compaction, which can be a significant factor affecting soil health and plant growth. It's important to understand the limitations of these sensors, including their depth and range, to ensure proper use.
The geographical variability of soil moisture within a field can also be identified using IoT systems. The ability to create detailed maps of soil moisture enables more targeted irrigation, reducing the need for across-the-board watering and improving crop health on a localized scale. This aspect presents advantages but increases the complexity of managing the system.
Many of the IoT sensors designed for soil moisture monitoring are built for extended use, with battery life extending up to several years. This extended battery life makes continuous monitoring feasible without needing frequent maintenance or replacement. Nevertheless, the long-term reliability and accuracy of these sensors will be an important consideration over time.
One potential future direction for IoT technology in soil moisture monitoring is its greater integration with drones. The combination of ground-based soil moisture sensors and aerial mapping through drones could significantly enhance the precision of irrigation and crop health management. However, ensuring interoperability and harmonizing data from multiple sources presents technical challenges that need to be addressed for wider implementation.
In conclusion, while IoT-driven soil moisture monitoring shows great promise for revolutionizing agricultural practices, ongoing research and development are essential to address challenges related to sensor reliability, data interpretation, system complexity, and ensuring long-term applicability in various agricultural contexts.
Soil Health Monitoring How AI and Structural Analysis Are Revolutionizing Precision Agriculture - Satellite Data Integration Enhances Large-Scale Soil Health Assessment
Satellite data is increasingly being used to assess soil health across large areas, revolutionizing how we manage agriculture. By using satellites to gather information, we can create detailed, digital maps of soil conditions, taking into account variations in soil types, how land is used, and weather patterns. This gives farmers more access to important soil data, allowing them to make decisions about their crops in a more timely way, which is crucial for good management. Moreover, combining satellite data with artificial intelligence enhances our understanding of how soil behaves, supporting farming methods that are more environmentally sustainable. Nevertheless, further study is vital to confirm the accuracy and usefulness of these methods across different types of farming operations. It's only with more testing that we can unlock the true potential of this technology for agriculture.
Integrating data from satellites into soil health assessments offers a compelling approach to improving our understanding of soil conditions across large areas. Satellite imagery can capture spectral signatures that reveal a lot about soil properties, such as nutrient levels, offering a more comprehensive view of nutrient availability compared to traditional, ground-based methods which often focus on a limited number of sampling points. One major benefit of this approach is the ability to cover expansive agricultural areas quickly, enabling real-time monitoring of soil health across numerous fields or land parcels, which would be highly time-consuming with traditional techniques.
Furthermore, the high-resolution images captured by some satellite platforms are sensitive enough to detect subtle changes in soil moisture content, a critical factor for optimizing irrigation strategies and forecasting potential water deficits before they negatively impact crop growth. The frequency of data collection from these platforms is also quite impressive, with some providing daily observations, offering an unparalleled ability to track changes in the soil environment. It is through the consistent collection of this kind of data that we can respond to shifting conditions more effectively, leading to proactive management of soil resources.
Beyond the temporal aspect, satellite imagery helps us to visualize the spatial variability of soil types within a single field, enabling farmers to tailor their practices more specifically to the requirements of various regions within that field, rather than implementing a one-size-fits-all approach. This approach to precision agriculture, which has emerged with improved satellite technology, improves our understanding of where specific management practices are most needed. Moreover, the satellite data can be coupled with existing soil health records, improving the performance of predictive models that forecast crop yield based on the current soil environment, leading to more informed decisions for optimizing agricultural production.
The reliance on extensive ground-based soil sampling is reduced with satellite data, a significant advantage as sampling can be quite expensive, time-consuming, and prone to human error, thus streamlining the process of assessing soil health and reducing the overall cost. The advancement of satellite imaging technology, with improved resolution and multi-spectral capabilities, has enabled us to differentiate between varying levels of soil organic matter, adding another dimension to soil management strategies.
It's also encouraging to note that satellite-based monitoring systems can act as early warning indicators for soil degradation, identifying erosion patterns and alterations in landscape structure before these issues become significant problems. This ability to spot potential degradation early is critical for ensuring soil health over the long term. When combined with localized sensor readings, the data from satellites can create a comprehensive view of soil health, leading to a more holistic understanding of soil dynamics and promoting a multi-scale approach to precision agriculture. This combination of macro-level trends (as captured by the satellite) and micro-level variability (from local sensors) is something that wasn't easily attainable just a few years ago, making the adoption of this type of technology quite intriguing.
More Posts from aistructuralreview.com: