Revolutionize structural engineering with AI-powered analysis and design. Transform blueprints into intelligent solutions in minutes. (Get started for free)
Southwest Airlines' AI-Powered Predictive Maintenance A Deep Dive into Aircraft Structural Integrity
Southwest Airlines' AI-Powered Predictive Maintenance A Deep Dive into Aircraft Structural Integrity - Southwest's Implementation of AIXI Technology for Predictive Maintenance
Southwest Airlines has adopted AIXI's AI technology to revolutionize their predictive maintenance strategy. This system leverages artificial intelligence to sift through data collected by aircraft sensors, identifying potential mechanical failures before they become critical. The goal is to proactively address maintenance needs, contributing to increased safety and reliability. By combining this predictive capability with their existing IFS maintenance management system, Southwest aims to streamline all aspects of maintenance, repair, and overhaul across their large workforce. This combination seeks to provide real-time insights into aircraft health and optimize maintenance schedules. Given the growing emphasis on aircraft structural integrity, Southwest's integration of AIXI might represent a forward-thinking model for the broader airline industry as it navigates the evolving landscape of aircraft maintenance. However, it remains to be seen whether the promised benefits fully materialize, particularly in the context of complex aircraft operations and a vast network.
Southwest has adopted AIXI's AI-driven system to refine their maintenance procedures. It leverages AI algorithms to sift through a massive volume of data originating from aircraft sensors. This data-driven approach allows for a more nuanced understanding of potential component malfunctions before they occur, going beyond traditional methods.
This technology has pushed Southwest towards a more proactive maintenance approach rather than responding to failures after they happen. A crucial aspect is that AIXI doesn't necessarily need a mountain of pre-labeled data. This quality is quite valuable, especially in complex environments like aviation, where the data is consistently changing.
Furthermore, AIXI can spot unusual patterns in flight data, enabling early detection of structural integrity problems. Its design aims to strike a balance between minimizing maintenance expenditures and mitigating the consequences of unpredictable component failures.
As more data gets input into AIXI, the predictive capabilities constantly adapt and improve. Southwest has woven AIXI into a system of interconnected AI agents which facilitate interaction and data sharing between various aircraft and maintenance teams, driving improvements in overall efficiency.
While AIXI offers the promise of lower maintenance costs, the integration of this complex technology into Southwest's existing operational practices has not been without hurdles.
Looking ahead, there's the possibility that AIXI's algorithm prowess could extend beyond predictive maintenance to optimize things like flight routes and even fuel efficiency, potentially adding yet another layer of benefit for Southwest. This presents an interesting future research question for the field.
Southwest Airlines' AI-Powered Predictive Maintenance A Deep Dive into Aircraft Structural Integrity - How AI Analyzes Aircraft Sensor Data to Forecast Maintenance Needs
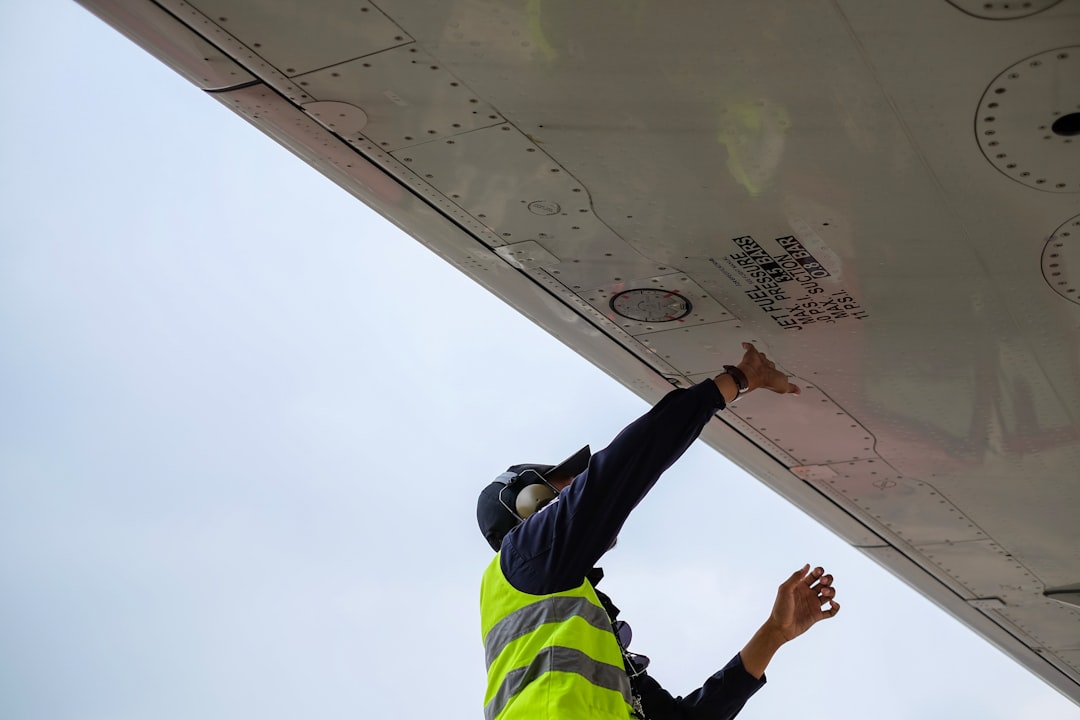
AI is increasingly being used to analyze the massive amounts of data collected by sensors on aircraft to anticipate maintenance needs. These algorithms can identify subtle signs of potential mechanical problems before they lead to failures, reducing the chance of unexpected maintenance events. By anticipating these issues, airlines can schedule maintenance proactively, leading to better aircraft reliability and potentially lowering costs by minimizing downtime.
Furthermore, this continuous monitoring of sensor data can reveal early signs of structural wear or fatigue, which is extremely important for ensuring aircraft integrity. This approach, as Southwest Airlines is demonstrating, is shifting the aviation industry towards a new era of smarter, data-driven maintenance strategies. However, we must remain cautious in evaluating the full impact of these technologies, especially as the operational environment is quite complex and always evolving. While the promise of increased safety and efficiency is enticing, realizing the full potential will require continuous development and integration efforts within existing airline operations.
Modern aircraft are equipped with over a thousand sensors, generating a massive amount of data throughout each flight. This data, which can reach several terabytes on longer flights, offers a treasure trove for predictive analytics. AI systems, like the one Southwest is using from AIXI, utilize advanced machine learning to analyze patterns of wear and tear, enabling airlines to develop replacement schedules for parts that are based on real-time conditions. This approach offers a departure from relying only on pre-determined intervals or historical data.
By digging into the data, we can also uncover specific flight situations that put more stress on particular aircraft components. This discovery can influence maintenance strategies and optimize performance across diverse operational conditions. Research indicates that predictive maintenance can lower unplanned maintenance events by a considerable amount (as much as 30% in some studies). This translates to greater aircraft availability and less downtime, a key operational advantage.
The heart of many data analysis methods lies in a technique called anomaly detection. It essentially flags minor deviations from standard operation, which can prove invaluable for forecasting problems before they lead to failures. Various sensor types capture a wide range of parameters – including temperature, pressure, and vibrations – essentially building a comprehensive health report for the aircraft. This multifaceted information helps enhance the accuracy of predictive models.
These algorithms have the capability to learn and adapt constantly as new data comes in. As an aircraft gets older, the predictive models' accuracy increases, as they are constantly adapting to evolving wear patterns. While the technology is impressive, it's important to acknowledge that implementing AI-driven predictive maintenance requires a substantial upfront investment. Airlines need to ensure that their systems and personnel are ready for this new technology.
Early identification of structural issues doesn't just lower safety risks, but also has a powerful impact on costs. Fixing issues before they worsen is usually far cheaper than reacting to a major system failure. Future AI advancements could leverage sensor fusion, where information from different sensors is combined and analyzed. This approach could potentially yield a more complete picture of an aircraft's health, leading to more streamlined maintenance routines. There's much more research needed to fully unlock the promise of AI in predictive maintenance, but the initial results from companies like Southwest are intriguing, at least for now.
Southwest Airlines' AI-Powered Predictive Maintenance A Deep Dive into Aircraft Structural Integrity - AI-Driven Inspection Systems Detecting Wear and Tear on Aircraft
AI is transforming aircraft maintenance by improving the detection of wear and tear. These advanced systems automate inspection processes, reducing the risk of human error in spotting critical issues. The Autonomous AI-enabled InspectoR (AAIR) illustrates one example of this advancement. These AI-powered systems analyze vast quantities of sensor data from aircraft, enabling predictive maintenance. This predictive approach allows for identification of potential mechanical problems before they cause failures, leading to safer and more reliable aircraft operations. Through continuous monitoring and analysis of this data, a much more comprehensive understanding of aircraft structural health is achievable. AI integration streamlines maintenance scheduling and procedures, offering a more proactive approach to aircraft upkeep. While promising significant improvements in both efficiency and safety, the deployment of these AI-driven inspection systems is a complex undertaking that requires ongoing assessment to ensure their effectiveness and to address any unforeseen challenges that might arise.
AI is rapidly transforming aircraft inspection processes, offering a substantial speed increase in spotting structural flaws compared to conventional methods. Human inspectors can take a considerable amount of time, whereas AI can often pinpoint issues in a fraction of that time. This swiftness is vital for keeping aircraft operational and ready for flights.
Sophisticated image recognition algorithms within these systems have the ability to scrutinize surface conditions with microscopic precision, identifying minuscule fatigue cracks that might be overlooked by the human eye. This enhanced accuracy boosts overall safety by permitting early interventions when issues are detected.
Traditionally, visual inspections relied on the expertise of human inspectors. But, research indicates AI-powered systems can achieve a level of consistency surpassing 95% in identifying particular defect types. This can significantly reduce the chances of maintenance oversights that might arise from human error.
AI-driven inspection systems incorporate data from multiple sensors in real-time. This capability allows the creation of a dynamic, 3D model of an aircraft's structural soundness. This, in turn, lets operators pinpoint areas experiencing localized stress under varying flight circumstances—an insight that static inspection methods can't provide.
These inspection systems use a continuous feedback loop, allowing them to refine their inspection parameters based on the data stream. This adaptation helps in identifying new wear patterns that emerge as aircraft age and their flight profiles change over time.
It's important to remember that although AI can pinpoint potential wear and tear, understanding the full implications of these findings still requires human expertise. Highly skilled engineers are needed to ensure appropriate maintenance steps are taken.
These inspection systems can carry out assessments during regular flight operations and overnight inspections, resulting in significantly less downtime. Airlines can optimize maintenance schedules without impacting flight schedules.
Studies show that AI inspection systems have the potential to identify up to 40% more anomalies compared to conventional methods. This suggests that these tools could enhance aircraft reliability and extend the life of different aircraft components.
AI-driven predictive analytics doesn't simply focus on individual components. Rather, it can analyze how different parts of an aircraft's structure are interconnected. This systemic understanding of wear and tear informs more comprehensive maintenance strategies.
Despite these advancements, implementing AI-driven inspection systems presents hurdles related to standardization and interoperability across different aircraft types. Further research and development efforts are needed to create industry-wide standards and practices for these kinds of technologies.
Southwest Airlines' AI-Powered Predictive Maintenance A Deep Dive into Aircraft Structural Integrity - Impact of AI-Powered Solutions on Reducing Airline Maintenance Costs
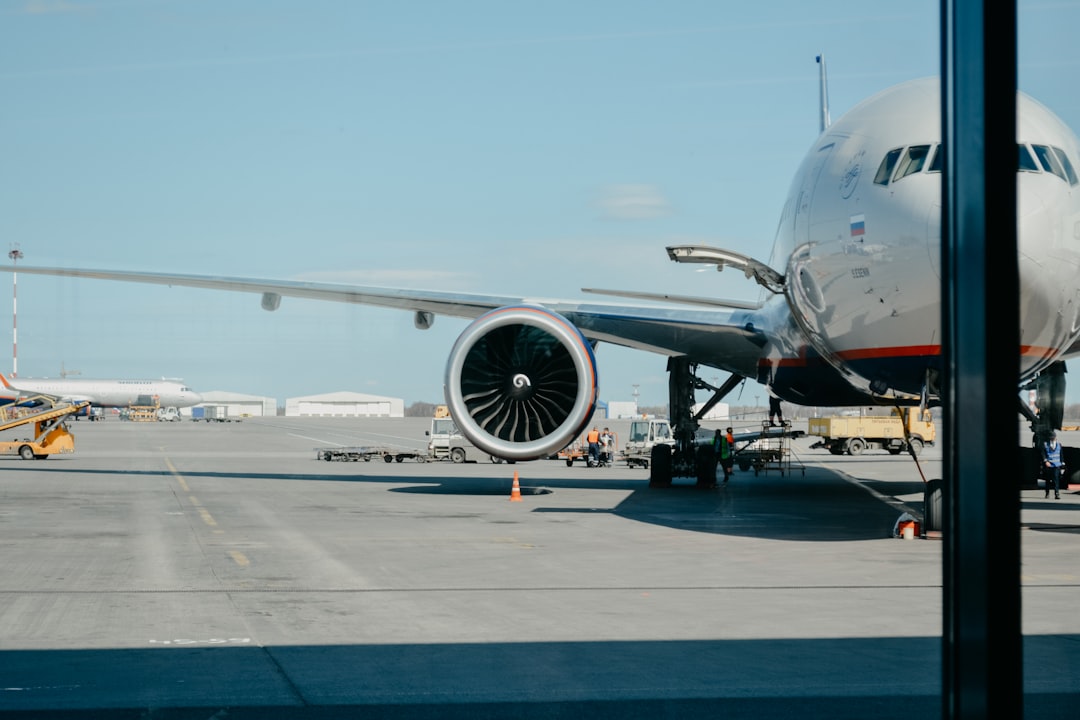
AI is transforming how airlines manage maintenance, leading to potential reductions in costs. By analyzing sensor data from aircraft, AI algorithms can predict when components might fail, enabling proactive maintenance instead of reactive repairs. This predictive approach, as demonstrated by Southwest Airlines, can optimize maintenance schedules, minimizing downtime and enhancing operational efficiency. Moreover, AI-driven inspection systems are improving the detection of wear and tear, potentially leading to earlier identification of structural issues, and further reducing the costs associated with repairs. While these AI-powered solutions show promise in streamlining maintenance and lowering expenses, the integration process is complex. The long-term effectiveness and full benefits of these technologies require continuous evaluation and adaptation within the dynamic context of airline operations. The complexities of implementing these systems require careful consideration and adaptation as the technology evolves.
AI-powered solutions are making waves in the airline industry by promising a reduction in maintenance costs. Studies show that these predictive systems can cut overall maintenance costs by as much as 25% by reducing labor and material expenses related to unforeseen repairs. This is a substantial change from the traditional, reactive approaches.
The sheer volume of data generated by modern aircraft—terabytes for a single long flight—can be meticulously analyzed by advanced AI algorithms to provide real-time insights into aircraft performance. This level of detail is a dramatic leap beyond the limitations of the older, static inspection methods.
Airlines are seeing some tangible benefits from this shift. With predictive maintenance strategies, aircraft availability rates can exceed 90%, which translates to fewer disruptions and increased operational efficiency. Having aircraft ready for flights more often, of course, results in higher revenue.
We also see an enhanced ability to detect anomalies in the structure of the aircraft. Using AI-driven inspection technology, airlines are finding up to 40% more issues than with traditional methods, leading to a better understanding of potential problems before they become serious.
What makes these AI-driven predictive models so powerful is that they can learn and improve. The algorithms' accuracy grows with each flight as they adapt to the changing patterns of wear and tear. This means maintenance schedules can become tailored to the specific aircraft rather than relying solely on historical averages or fixed schedules.
The AI's real-time capabilities are also notable. It can quickly alert maintenance crews to developing problems in a matter of minutes, optimizing the repair process. This reduces the need for more widespread inspections and can streamline repair workflows, leading to faster resolution of issues.
By automating inspections with AI, it's possible that airlines could save substantial time. Thousands of man-hours annually, researchers suggest, might be freed up allowing technicians to focus on complex tasks that need human attention.
Further, the algorithms can forecast the lifespan of parts with increasing accuracy. This capability allows airlines to improve inventory management and optimize procurement, likely leading to savings on parts. By better aligning part purchases with actual needs, rather than guessing, they could avoid overstocking.
While AI presents tremendous potential, it comes with upfront costs. Airlines may need to invest millions in technology and training, which raises questions about the return on investment, especially in the early phases of deployment.
If AI-powered maintenance solutions continue to gain wider adoption, they could reshape the aviation industry's benchmarks. They could cause a shift from older, traditional maintenance cycles and schedules, leading to new standards for how aircraft maintenance is done and optimized. It will be very interesting to see how these changes take hold in the years to come.
Southwest Airlines' AI-Powered Predictive Maintenance A Deep Dive into Aircraft Structural Integrity - Comparison with United Airlines' Predictive Maintenance Partnership
When assessing Southwest Airlines' approach to AI-powered predictive maintenance, it's valuable to compare it to United Airlines' efforts. United's partnership with Lufthansa Technik focuses on creating predictive maintenance systems specifically designed for their large fleet of Boeing 737s. This illustrates a more conventional path within the industry. Though both airlines are incorporating AI to improve maintenance, Southwest's collaboration with AIXI demonstrates a more pioneering, distributed strategy. Southwest emphasizes real-time analysis of aircraft data and taking proactive steps to address potential issues. This might offer a greater degree of flexibility in monitoring aircraft health over the long term. Despite these differences, the central difficulties of combining data sources and ensuring different systems work together remain for both Southwest and United. These obstacles are major for unlocking the full potential of these advanced maintenance systems. Given the persistent increase in maintenance expenses and the growing intricacy of airline operations, it will be crucial for both Southwest and United to continue refining and updating their AI strategies to achieve the expected benefits of these advanced technologies.
In contrast to Southwest's internal development approach with AIXI, United Airlines has opted for a collaborative model. Partnering with companies like Lufthansa Technik and integrating third-party software, United aims for a more comprehensive approach to aircraft health monitoring. This partnership model may speed up maintenance processes and improve efficiency. Notably, United's early adoption of a data analytics platform that blends historical records and sensor data gave their efforts a boost. This holistic perspective on aircraft performance over time provides a more thorough understanding of potential issues compared to Southwest’s more targeted AI solution.
Reports suggest United's approach has led to a significant reduction in unscheduled maintenance, with some estimates claiming a 30% decrease. This is a compelling argument for the effectiveness of their predictive maintenance compared to traditional methods. However, the choice between United's collaborative, third-party software model and Southwest's internal approach raises questions about the best path for the airline industry to follow. While Southwest emphasizes internal AI development, United seems to rely more heavily on integrating external software.
Further, United’s approach incorporates advanced machine learning methods to anticipate not just immediate maintenance but also long-term asset management decisions. This strategic perspective on aircraft lifecycle costs contrasts with Southwest's current focus solely on predictive maintenance. They've also prioritized training their maintenance teams in AI and data analysis, acknowledging it's vital for using predictive analytics effectively. This could be a significant hurdle for Southwest as they integrate the new AIXI technology into their established workflows.
United's centralized data operations center for managing predictive maintenance efforts stands in contrast to Southwest's potentially more decentralized setup. While a centralized approach could help United react more quickly to issues across their larger fleet, it can also introduce a higher level of complexity. United's larger fleet, encompassing a wider range of aircraft types, poses unique challenges for their predictive algorithms that Southwest should consider as they roll out AIXI across different aircraft models.
United's commitment to innovation also extends to research collaborations with NASA, positioning them at the cutting edge of predictive maintenance technologies in the aerospace sector. This proactive approach contrasts with Southwest's largely internal approach. Yet, one potential drawback of United's approach is the complexity arising from interfacing various data sources. While powerful, this integration can lead to inconsistencies if not managed effectively, potentially hindering decision-making. This aspect underscores the importance of careful management of data, a challenge that Southwest’s approach, with AIXI’s integrated system, may have a simpler path to overcome. These points of comparison offer insights into the different approaches to AI-driven aircraft maintenance within the airline industry, hinting at the varied challenges and opportunities inherent in pursuing this technology.
Revolutionize structural engineering with AI-powered analysis and design. Transform blueprints into intelligent solutions in minutes. (Get started for free)
More Posts from aistructuralreview.com: