Understanding Integrated Circuits From Silicon Wafer to Neural Network Computing in Modern AI Systems
Understanding Integrated Circuits From Silicon Wafer to Neural Network Computing in Modern AI Systems - Silicon Wafer Manufacturing From Raw Sand to Crystalline Perfection
The creation of silicon wafers, the foundation for countless electronic devices, is a fascinating journey starting with humble silica sand and culminating in flawless crystalline silicon. The process involves a series of carefully controlled steps, beginning with refining the sand to remove impurities. This purified material is then subjected to techniques like the Czochralski method, a process that essentially "grows" a single crystal of silicon from a molten state.
Subsequent steps involve cutting the crystal into thin wafers, then polishing them to exacting specifications. Every stage, from initial purification to the final polish, is crucial for achieving the necessary purity and structural perfection needed for advanced integrated circuits and other semiconductor applications. The performance and reliability of these devices are critically impacted by the quality of the silicon wafers. Minimizing impurities and defects within the silicon remains a significant challenge, with researchers constantly seeking better methods to improve the uniformity and quality of the final product.
This meticulous manufacturing process is central to technological progress, impacting a wide array of applications—from the supercomputers powering scientific discoveries to the neural networks driving the advancements in modern artificial intelligence systems. The silicon wafer, a seemingly simple piece of material, truly lies at the heart of this technological revolution.
Silicon wafer manufacturing begins with the most basic of elements—sand, specifically silica sand. However, this raw material undergoes a rigorous purification process to eliminate impurities such as iron and aluminum. These impurities can significantly hinder the electrical performance of the final silicon. The goal is to achieve an incredibly high purity level, often exceeding 99.9999999%, a testament to the exacting requirements of modern semiconductor fabrication.
The resulting purified silicon needs to be arranged in a specific way to be useful for electronics. The Czochralski process is a key method for achieving this. This process involves growing a large single crystal of silicon by slowly pulling a seed crystal from a pool of molten silicon. The carefully controlled process creates a silicon ingot with a very organized atomic structure. This single crystal structure is absolutely essential for the performance of modern integrated circuits.
However, even in this carefully controlled process, imperfections can arise. Crystal defects such as dislocations can negatively impact the electrical properties of the final silicon wafer and, consequently, the chips made from it. These defects become a critical concern for manufacturers, demanding careful control and monitoring throughout the process.
Over time, manufacturers have discovered ways to optimize the entire process and one significant change is the size of the silicon wafers. Starting with much smaller diameters, modern semiconductor factories now commonly use wafers measuring 300 mm (12 inches) or even larger. Increasing the size of wafers has economic advantages because more chips can be fabricated on a single wafer, reducing the overall cost per chip.
Preparing the wafer surface for fabrication is a crucial step that involves meticulously smoothing and removing any oxide layers. This surface treatment is essential for subsequent processing steps like photolithography. Even the slightest imperfection on the wafer surface can disrupt the precise patterns that are etched onto it, potentially impacting the functionality of the integrated circuit.
A critical method used in this stage is Chemical Vapor Deposition (CVD). CVD is used to carefully deposit extremely thin films of different materials onto the silicon wafer. The uniformity and precision of the film deposition are crucial to the eventual performance of the semiconductor devices. This is just one example of the incredible precision required in silicon wafer fabrication.
Doping is another critical step that introduces controlled amounts of specific impurities into the silicon. This process is done to precisely alter the electrical characteristics of the silicon, enabling engineers to create areas that are either p-type or n-type semiconductors. These p-n junctions are foundational to the transistors and other electronic devices built upon the silicon wafer.
Before the silicon wafer is cut into individual chips, it undergoes rigorous testing to detect any defects. These tests are crucial to ensure quality because, in some cases, as much as 50% of the wafers produced can be discarded due to defects. The quality control measures are extremely stringent and necessary.
The transformation of silicon from raw sand to wafer is a process that involves intense thermal manipulation. Numerous high-temperature processing steps, often exceeding 1,400 degrees Celsius, are required. This emphasizes the importance of advanced thermal management and control throughout the entire manufacturing process.
While silicon remains the workhorse of the semiconductor industry, it's crucial to note that researchers are constantly looking for alternatives. Materials like graphene and silicon carbide are being explored due to their potential advantages, such as improved heat dissipation and energy efficiency. This ongoing search for new materials underscores the continuous drive for innovation within semiconductor technology.
Understanding Integrated Circuits From Silicon Wafer to Neural Network Computing in Modern AI Systems - Transistor Architecture and CMOS Technology in Modern IC Design
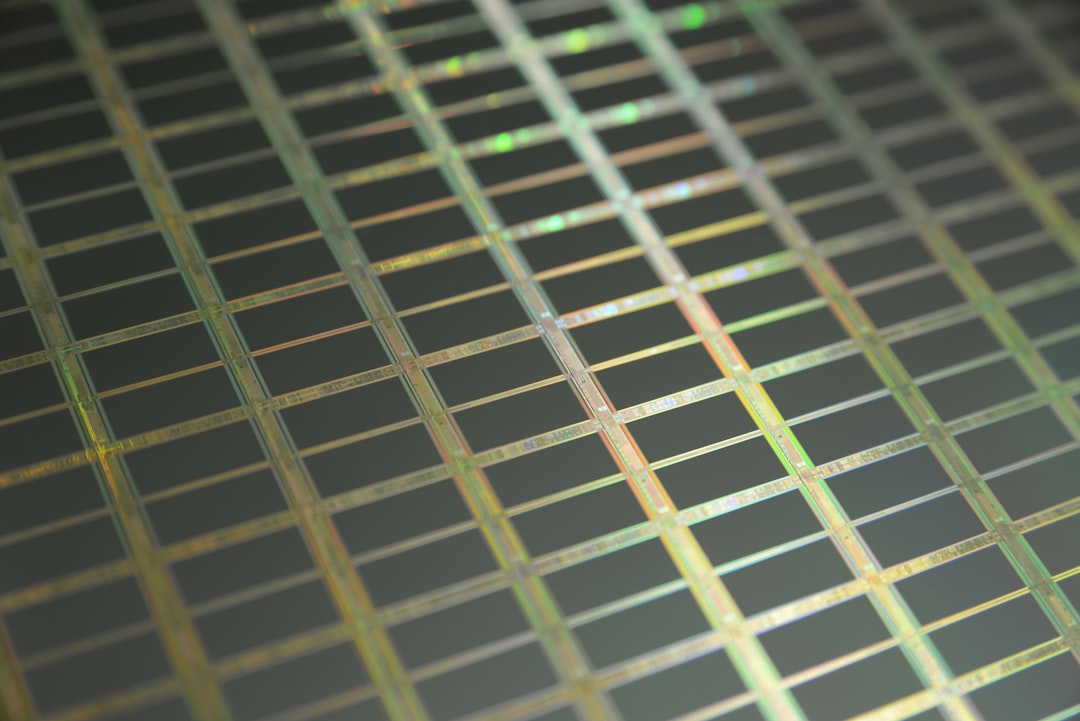
The design of modern integrated circuits (ICs) is deeply intertwined with the architecture of transistors and the dominant CMOS technology. CMOS, which utilizes complementary pairs of transistors, has become the standard for most electronic devices due to its energy efficiency compared to earlier technologies like TTL and nMOS. This dominance has led to a focus on developing design techniques that minimize power consumption while maximizing performance. Furthermore, there's a growing interest in exploring techniques like cooling ICs to extremely low temperatures to further enhance energy efficiency. The landscape of IC design is evolving towards increasingly complex 3D architectures, which bring about unique design challenges relating to how components interact and are physically interconnected. At the core of this evolution is a detailed understanding of CMOS components, especially MOSFETs (Metal-Oxide-Semiconductor Field-Effect Transistors), which is crucial for optimizing both present and future IC designs, especially within the context of growing AI applications where energy efficiency and performance are highly valued. While CMOS currently reigns supreme, there's a constant push to innovate, and it remains to be seen what technologies may emerge to challenge CMOS's dominance in the future.
Complementary metal-oxide-semiconductor (CMOS) technology has become the cornerstone of modern integrated circuit (IC) design, driving the development of everything from smartphones to supercomputers. Its dominance stems from its ability to pack billions of transistors onto a single chip, drastically boosting processing power. This feat is achieved through relentless miniaturization, with transistors now reaching dimensions as small as 3 nanometers. However, this relentless drive to shrink has introduced its own set of challenges.
One key development has been the emergence of FinFET architecture. Traditional planar transistors struggle to maintain performance at these incredibly small scales, and FinFET, with its 3D structure, offers better control over the transistor channel, reducing leakage current and enhancing overall speed. It's become an essential innovation for advanced CMOS designs.
Another pivotal change in CMOS technology has been the adoption of high-k dielectrics. These materials, which have a higher electrical permittivity than the previously used silicon dioxide, play a crucial role in mitigating leakage and improving electrostatic control, critical at nanoscale dimensions. It represents a significant step in material science within the semiconductor field.
While CMOS has become ubiquitous for digital ICs, bipolar junction transistors (BJTs) still hold their ground in analog circuits. They offer particular advantages in specific applications, demonstrating that the choice of technology depends on the operational needs of a specific IC.
Interestingly, as transistors become smaller, the impact of electrical noise increases. Quantum effects and thermal fluctuations introduce uncertainties into signals, highlighting the need for innovations like differential signaling to ensure accuracy and performance. This noise remains a significant challenge in maintaining signal integrity within shrinking ICs.
The reality of IC manufacturing is that slight variations in the production process are unavoidable and can lead to inconsistencies in transistor performance, even within the same batch of chips. Techniques like Statistical Process Control (SPC) play a vital role in pinpointing and mitigating these inconsistencies, helping to ensure consistency and quality of the final product.
A key trend in modern IC design is the integration of advanced packaging technologies like System-in-Package (SiP) and chiplet architectures. These approaches are intended to improve performance and minimize delays. Moreover, they pave the way for heterogeneous computing systems, where different types of chips can collaborate, leading to more sophisticated computational systems.
As we continue to shrink transistors, susceptibility to errors caused by external factors, such as radiation or cosmic rays, increases. This problem is more pronounced in specialized applications like space exploration and high-performance computing, necessitating the development of more robust, fault-tolerant designs. This ongoing challenge highlights the need for continuous innovation in circuit architectures to address potential weaknesses.
The drive for higher performance often clashes with the demand for energy efficiency. Engineers constantly grapple with the trade-off between computational speed and power consumption, as well as with thermal management concerns. This necessitates the use of sophisticated circuit optimization strategies to meet specific design requirements.
Silicon remains the primary material for ICs, but its limitations are becoming increasingly apparent. Researchers are looking to materials like transition metal dichalcogenides (TMDs) and carbon nanotubes to overcome these limitations. These materials have the potential to improve transistor characteristics and possibly lead to a new generation of flexible and wearable electronics, showing that the pursuit of innovation in semiconductor materials is still a very active field of research.
Understanding Integrated Circuits From Silicon Wafer to Neural Network Computing in Modern AI Systems - Parallel Processing Units Supporting Machine Learning Computations
Machine learning, a core component of modern AI systems, relies heavily on parallel processing to handle the immense computational demands of training and utilizing complex neural networks. Specialized processing units have emerged to address this need, with a primary focus on maximizing performance while minimizing energy use.
Tensor Processing Units (TPUs), developed for Google's AI initiatives, exemplify this trend. They are hardware specifically optimized for the mathematical operations commonly found in neural networks. The key benefit is that they are exceptionally efficient at handling the large quantities of low-precision computations typical of deep learning. This results in substantial gains in speed and energy efficiency, crucial for both training massive datasets and deploying trained models for inference tasks.
Graphic Processing Units (GPUs), originally designed for computer graphics, have also proven highly effective for machine learning due to their ability to perform numerous calculations concurrently. The inherently parallel nature of GPUs translates into significant speedups for a wide range of machine learning algorithms.
However, ongoing research explores even more advanced architectures. Neuromorphic photonics, for example, explores using light instead of electrons to execute computations, aiming for significant improvements in speed and efficiency. Memristors, another promising technology, offer an alternative approach to transistor-based designs, providing potential for high parallelism and low power consumption.
The continuous drive to develop these specialized and increasingly parallel computing units is driven by the need for AI systems that can handle the exponentially growing complexity of modern AI applications. The future of machine learning and AI is tightly coupled with innovations in how we design and implement computing architectures, pushing towards novel solutions to tackle the increasingly complex and computationally intensive tasks needed to create more intelligent and adaptable systems.
Parallel processing units (PPUs) are a crucial element in accelerating machine learning calculations. Unlike traditional central processing units (CPUs) which perform operations sequentially, PPUs can handle multiple operations concurrently, leading to a substantial speed-up for machine learning algorithms. This parallel processing is especially important for handling the massive datasets commonly used in today's AI systems, enabling real-time processing that would be impossible with sequential approaches.
Specialized architectures, like Graphics Processing Units (GPUs) and Tensor Processing Units (TPUs), are optimized for the types of mathematical computations prevalent in neural network training. These PPUs are specifically designed for operations like matrix multiplication, making them far more efficient for AI workloads than general-purpose CPUs. However, the efficacy of these specialized units heavily relies on memory bandwidth. To maximize performance, modern PPUs incorporate technologies like High Bandwidth Memory (HBM) which allows for faster data transfer rates. These high-speed data transfer mechanisms are essential for coping with the large volumes of data inherent in machine learning.
Interestingly, the level of floating-point precision also plays a role in the efficiency of PPUs. Many machine learning calculations can be performed at lower precision without sacrificing too much accuracy. Recently, we've seen the development of PPUs with mixed-precision capabilities, offering a balance between improved performance and energy efficiency.
Moreover, the parallel architecture of PPUs makes them highly scalable for machine learning. When workloads increase, more processing units can be integrated to distribute the computational burden. This feature allows for greater complexity in models without requiring extensive hardware redesign.
It's notable that some companies are developing custom PPUs, often referred to as Application-Specific Integrated Circuits (ASICs). These tailored solutions are often optimized for specific machine learning algorithms and offer performance advantages over general-purpose PPUs. However, with this increased specialization, we see a trade-off emerge between latency and throughput. The design of a PPU will often favor either fast response times or high data processing rates depending on the intended AI application. This choice highlights the importance of carefully considering the architectural design in the development of AI systems.
The intensive calculations performed by PPUs generate considerable heat, which can negatively impact performance. Advanced thermal management methods, such as liquid cooling, are becoming increasingly important in data centers where these units are widely used. The challenge of efficiently moving data between memory and processing units remains a significant hurdle. Efforts are focused on techniques like data locality and improved cache architectures to minimize potential bottlenecks.
The evolution of PPUs has resulted in a need for greater standardization across platforms. Initiatives like OpenCL and CUDA allow different hardware configurations to run machine learning algorithms seamlessly. This fosters a more adaptable ecosystem for AI development and allows for broader deployment of AI across different hardware.
While the development of PPUs has significantly accelerated the progress of machine learning, the field still faces challenges. Ongoing research and development will likely lead to further improvements in PPU design, thermal management, and data movement optimization, making machine learning and AI systems even more powerful and efficient in the future.
Understanding Integrated Circuits From Silicon Wafer to Neural Network Computing in Modern AI Systems - Memory Hierarchies and Data Flow Management in Neural Network ICs
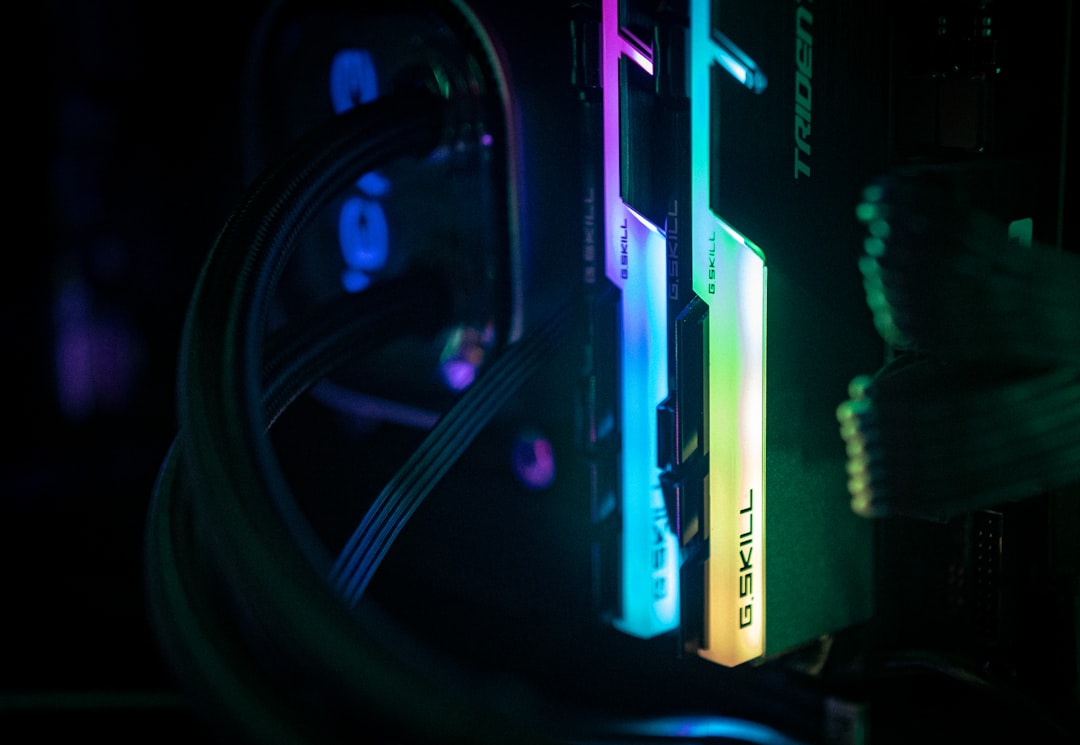
Neural network integrated circuits (NNICs) rely heavily on efficient memory hierarchies and data flow management to achieve optimal performance. As the scale and complexity of datasets used in modern AI grow, the ability to quickly and efficiently move data between different memory levels becomes paramount. Techniques like in-memory computing (IMC) attempt to optimize data flow by performing computations within the memory arrays themselves, reducing the need for extensive data movement between memory and processing units. This approach can lead to significant energy savings, a critical factor in many AI applications.
Furthermore, processing-in-memory (PIM) architectures are gaining traction as a way to tackle the limitations of traditional monolithic chip designs. The adoption of chiplet-based designs allows for improved scalability and flexibility, enabling the creation of more complex and adaptable neural network systems. However, the increasing reliance on parallel processing in NNICs presents unique challenges in coordinating and synchronizing data flows across numerous processing elements.
Beyond these existing approaches, the field is seeing a growing emphasis on bio-inspired design principles. Neuromorphic computing, which draws inspiration from the structure and function of biological brains, is being explored as a potential path to increased energy efficiency. Similarly, the development of new hardware solutions, such as specialized accelerators tailored for deep learning, is actively advancing the field. While the benefits of these approaches are promising, many of them are still in early stages of development and there are open questions about their scalability and practicality in real-world scenarios.
The continued evolution of NNIC design will hinge on the ability to not only improve computational performance but also address the increasing energy consumption and processing demands of the field. A thoughtful assessment of current memory hierarchy and data flow management approaches alongside a commitment to exploration and innovation in these areas are crucial to driving further advancements in the efficiency and scalability of neural networks within integrated circuits.
Memory hierarchies and how data moves around within neural network integrated circuits (ICs) are really important for making them fast and energy-efficient, especially when dealing with massive datasets. The way memory is structured, with different levels like registers, caches, main memory, and external storage, is crucial for keeping delays low and maximizing how much data can be processed.
Managing the flow of data between processing units and memory is key to making these chips perform well. Techniques like optimizing where data is stored and scheduling tasks efficiently can have a huge impact on how quickly neural networks can learn and make predictions. However, there's a persistent challenge with the speed of data transfer. As neural networks become more complex, the amount of data that needs to be moved around can easily overwhelm the current memory technologies. This becomes a major bottleneck, preventing us from getting the full potential of these chips.
SRAM memory is a popular choice for on-chip storage in neural network processors because it's fast and can handle lots of data quickly. But, it's more expensive and takes up more space compared to DRAM, meaning designers have to carefully balance these trade-offs when building chips.
One way to make neural networks more efficient is to reduce the precision of their computations. This quantization technique can help save memory and speed up processing, but it also can introduce errors into the network's results. Designers need to be aware of these accuracy trade-offs when they are working with lower precision.
We're seeing new memory technologies being combined with traditional memory to improve both speed and capacity. High Bandwidth Memory (HBM) and 3D-stacked memory are good examples of this. These hybrid systems aim to address the issues with single memory types.
Caching is another clever strategy used to improve performance. It allows the processor to anticipate the data it'll need, so it can avoid having to wait for data from slower memory. Caches are designed to hold the data that's most frequently accessed, leading to huge gains in how quickly computations can be performed.
For tasks that require multiple chips or computers, the way data is moved between them and how they synchronize their operations becomes very important. Efficient algorithms are needed to manage this flow without creating bottlenecks or conflicts in data.
The unique way deep learning networks access data, such as in convolutional layers, has a major impact on the design of the memory system. Understanding these patterns can help engineers tailor the memory system for maximum effectiveness.
And then there's the issue of heat. High-performance memory generates a lot of heat, which can affect the performance of the chip if it's not managed effectively. That's why efficient cooling solutions are becoming more and more important to keep these chips running reliably. All of these aspects are intricately connected, illustrating the continuous exploration and innovation in this field as researchers strive for the perfect blend of efficiency and performance in AI hardware.
Understanding Integrated Circuits From Silicon Wafer to Neural Network Computing in Modern AI Systems - Power Management and Heat Distribution in AI Processing Chips
AI processing chips, especially those designed for tasks like neural network training and inference, face increasing pressure to deliver high performance while minimizing energy consumption. The nature of AI workloads, which often involve massive parallel computations, generates substantial heat within the chips. This heat, if not effectively managed, can lead to decreased performance, reliability issues, and even hardware damage. Efficient power management and heat distribution are thus paramount, requiring careful consideration of chip architecture and material choices.
Approaches like processing-in-memory (PIM) aim to reduce power consumption and thermal issues by performing computations directly within the memory units. This reduces the need to shuttle data between memory and processors, thereby saving both energy and minimizing the heat generated by data transfers. The exploration of novel technologies, such as memristors, holds potential for further improvements in energy efficiency and heat dissipation compared to traditional silicon-based transistor designs. However, as AI applications become more prevalent across various devices, striking a delicate balance between computational demand, power efficiency, and heat management will remain a key challenge in the development of future AI hardware. It's a crucial area of research for ensuring the continuous progress and scalability of AI in the years to come.
The increasing computational demands of AI applications, particularly those deployed on edge devices, are pushing the boundaries of traditional power management and heat distribution within processing chips. The sheer amount of processing power needed by AI, especially in deep learning tasks, results in substantial heat generation, often exceeding 100 degrees Celsius. This poses a significant challenge because sustained high temperatures can lead to chip degradation and performance loss. Efficient cooling strategies are therefore critical for reliable AI operation, prompting exploration of techniques like advanced liquid cooling or integrated heat spreaders.
Furthermore, AI chips consume a substantial amount of power, often exceeding 20-30 watts per processing unit. As these chips continue to scale, managing the power distribution and the resulting heat becomes more intricate. Poor power management can lead to thermal throttling, a process where the chip reduces its clock speed to prevent overheating. This, in turn, impacts the overall performance of the AI system. Engineers are working to develop innovative power distribution architectures that can mitigate this issue and maintain a balance between energy efficiency and processing power.
This need for both performance and efficiency is particularly acute in edge AI applications. These applications often operate in resource-constrained environments such as mobile devices or remote sensors. Here, power consumption and heat generation become limiting factors because of battery life restrictions or limitations in cooling options. Innovative design approaches, with a focus on low-power circuits and efficient thermal management solutions, are essential for these scenarios.
One promising approach to better thermal management involves the use of 3D chip stacking. This approach vertically integrates multiple layers of chips, reducing the distances data needs to travel, thereby leading to reduced latency and lower heat dissipation. This inherently offers more efficient power management strategies because of the closer proximity of processing units and memory.
The quest for innovative thermal solutions has spurred research into custom cooling mechanisms such as phase-change materials or highly effective thermal interface materials. These custom solutions, when integrated into the chip design, can keep the chip at optimal operating temperatures, ensuring maximal performance without compromising the output of the AI tasks.
Modern AI chips also leverage dynamic power management techniques like Dynamic Voltage and Frequency Scaling (DVFS). DVFS intelligently adjusts the voltage and frequency of the chip based on the workload, minimizing power consumption and heat generation during less intensive tasks. This adaptive strategy ensures that the chip is not constantly operating at peak power, saving energy and reducing the thermal strain.
The materials used for interconnects within AI chips are also under scrutiny. The thermal properties of these interconnects can have a significant effect on the overall thermal performance. Materials like carbon nanotubes, which exhibit promising thermal and electrical properties, are actively being researched as potential replacements for conventional interconnect materials. This suggests that there is still room for innovation and progress in improving how AI chips manage heat, a crucial factor for overall system performance.
The memory hierarchy of an AI chip has a close connection to both performance and thermal output. Effective cache management can optimize data flow, which minimizes the need for frequent memory accesses by the processing units, leading to a reduction in energy consumption and heat generation. This exemplifies how the interconnectedness of system design affects the various performance aspects.
Remarkably, AI techniques are being used to optimize power management within the AI chips themselves. By training machine learning models to predict computational loads, we can adjust the power distribution and manage the heat dynamically. These 'self-aware' systems enable more efficient and adaptive control over power consumption and thermal characteristics, highlighting the potential of AI to solve some of the challenges it creates.
The pursuit of improved materials continues with efforts focused on thermoelectric materials, which offer a compelling alternative to traditional cooling methods. Instead of just passively removing heat, these materials potentially convert waste heat into usable electrical power, representing a fascinating intersection of thermal management and energy generation. This ongoing research highlights the breadth of innovation aimed at pushing the boundaries of energy efficiency and power management in advanced AI systems.
Understanding Integrated Circuits From Silicon Wafer to Neural Network Computing in Modern AI Systems - Circuit Integration Between Analog Sensors and Digital Neural Networks
The convergence of analog sensors and digital neural networks is reshaping AI system design, pushing beyond traditional digital-only paradigms. Analog sensors, being inherently suited for capturing real-world events, offer a rich, continuous stream of data that can be directly utilized by digital neural networks, leading to improved overall performance. This interplay also facilitates in-sensor computing, where data processing begins within the sensor itself, minimizing delays and energy expenditure compared to transferring raw analog data to a separate processing unit.
The pathway for achieving this seamless integration requires innovative mixed-signal circuit design and, increasingly, the exploration of memristor-based circuits that could optimize the handling and processing of analog sensor data. These approaches hold the potential for highly efficient and streamlined transfer between the sensor and the neural network's processing units. However, synchronizing the different data streams and optimizing the analog-to-digital conversion process are critical challenges that are constantly addressed through ongoing research. It's a complex and constantly evolving field where the design of the integrated circuits needs to seamlessly manage the transition from the physical world, as sensed by the analog sensors, to the computational world of the neural network. This careful balance between analog and digital domains is a crucial area for future advancements, as it's essential for developing efficient and adaptable AI systems.
The merging of analog sensors with digital neural networks presents a fascinating and often overlooked challenge in integrated circuit design. This hybrid approach offers a unique path to capturing the rich detail of real-world signals in the analog domain, effectively reducing the need for conversion to digital formats. Such conversions can add delays and introduce errors into the system.
One intriguing aspect of this integration is the possibility of directly training neural networks on raw sensor data. This can potentially boost performance in areas like image recognition and environmental monitoring, as the network learns to understand the nuances of the analog signal itself, preventing the loss of critical information during digital quantization.
Interestingly, analog sensor interfaces can lead to significantly lower power consumption compared to digital processing. This reduction in power is primarily seen during data acquisition, which is advantageous for devices operating on batteries or in environments with limited energy resources, making them especially relevant for edge computing scenarios.
Moreover, analog circuits can often offer superior resistance to noise compared to their digital counterparts, a benefit particularly valuable in environments with significant electromagnetic interference. This resilience can allow analog sensors to reliably transmit data to neural networks for processing, even in challenging circumstances.
Hardware acceleration is another interesting development in this area. Current designs are exploring the concept of implementing computations close to the sensor using analog circuits to accelerate the inference process in neural networks. This combined design approach for analog front ends and digital processing could significantly enhance processing speeds and eliminate bottlenecks frequently encountered in traditional architectures where sensing and processing are separate stages.
Scientists are investigating resonant neural network architectures within the analog domain where individual neurons can oscillate at specific frequencies. This technique has shown promise for handling temporal data and enhancing the responsiveness of AI models in time-critical applications.
Integrating multiple types of analog sensors within a single neural network may lead to powerful synergistic effects. For instance, combining data from temperature, pressure, and humidity sensors can improve the accuracy of environmental models, opening opportunities for use in intelligent home systems and advanced robotics.
Recent progress in this area involves using analog signals to dynamically influence the weights of connections in neural networks. This dynamic manipulation of weights could pave the way for real-time adaptive learning, where the network can adapt in response to input signals, drawing parallels to how biological neural networks function.
Despite these advantages, scaling analog-digital integrated systems presents considerable challenges due to the inherent tradeoffs in precision and the inherent complexities of mixed-signal circuit design. This necessitates innovative approaches to circuit design to maintain functionality as systems become larger and more complicated.
Finally, there's a potential for leveraging quantum effects in nanoscale analog devices to achieve advancements in computing. These devices could potentially act as both sensors and computational units, leading to entirely new paradigms for integrated circuits that go beyond the principles of classical electronics. The exploration of quantum effects could be a game-changer for future AI systems.
More Posts from aistructuralreview.com: