Revolutionize structural engineering with AI-powered analysis and design. Transform blueprints into intelligent solutions in minutes. (Get started for free)
Optimizing K-Omega SST Model Parameters for Enhanced Near-Wall Flow Prediction in Wind Turbine Applications
Optimizing K-Omega SST Model Parameters for Enhanced Near-Wall Flow Prediction in Wind Turbine Applications - Near-Wall Flow Coefficient Adjustments for K-Omega SST Model Applications in NACA 0012 Airfoils
The effectiveness of the k-omega SST turbulence model in predicting near-wall flow, especially for airfoils like the NACA 0012, is significantly impacted by adjustments to the near-wall flow coefficient. This coefficient governs the behavior of the boundary layer, directly affecting crucial quantities like wall shear stress and heat transfer, particularly in scenarios with complex flow characteristics. While a standard value of 0.5 is often used, deviations from this standard can lead to considerable improvements in flow predictions, especially when dealing with high Reynolds number flows around airfoils.
The k-omega SST model's hybrid structure, combining the strengths of both k-omega and k-epsilon formulations for near-wall and freestream regions respectively, provides the versatility to handle different flow regimes. This versatility is further enhanced by utilizing experimental data to calibrate model parameters. Such calibration is critical, especially for capturing complex flow phenomena like flow separation, which is key for understanding and maximizing the aerodynamic performance of airfoils, especially within wind turbine applications. Optimizing the model further requires the incorporation of advanced wall treatments and adaptive remeshing techniques to better resolve the intricacy of complex flow dynamics in engineering simulations.
1. The near-wall coefficients within the K-omega SST model have a profound effect on the turbulence model's behavior near the surface, particularly impacting the prediction of boundary layer growth—a critical aspect of accurate aerodynamic analysis for airfoils like the NACA 0012. This sensitivity stems from the model's inherent focus on the turbulence production within this region.
2. The K-omega SST model's core strength lies in its ability to represent the generation of turbulence close to the wall. This detailed representation has significant implications for predicting the shear stress, directly influencing the lift and drag forces, which are paramount in achieving efficient wind turbine operation.
3. Modifying these near-wall coefficients can remarkably influence the accuracy of flow separation predictions, especially under high-lift conditions where flow separation becomes more dominant, impacting overall airfoil efficiency. It's a fine balancing act between achieving accurate predictions and potentially over-fitting.
4. Experimental findings have clearly illustrated that subtle changes to these near-wall coefficients can result in surprisingly large deviations—up to 15%—in drag predictions. This underlines their critical role in the reliability of computational fluid dynamics simulations, particularly when evaluating wind turbine performance.
5. The model's performance exhibits a strong dependence on the mesh's spatial resolution close to the wall. The choice of mesh density becomes a crucial parameter, especially when dealing with the complexity of the NACA 0012 profile and attempting to capture the dynamics of near-wall flow.
6. Research indicates that improperly configured near-wall coefficients can lead to an underestimation of turbulent kinetic energy. This can compromise the accuracy of simulations, especially in cases involving vortex shedding, which is important for dynamic stall scenarios and understanding complex wind turbine loads.
7. While wall functions can simplify calculations, omitting proper adjustments to the near-wall coefficients can generate significant errors in pressure predictions across the airfoil surface. The trade-offs between computational efficiency and accuracy need to be carefully considered.
8. Researchers have developed advanced techniques, leveraging real-time flow measurements to optimize these near-wall coefficients. This approach leads to a much closer alignment of simulation results with empirical data, thus significantly enhancing the model's predictive capability.
9. The ability of the K-omega SST model to predict challenging flow phenomena like adverse pressure gradients at airfoil leading edges is heavily tied to the precision of these near-wall coefficients. Any inaccuracy in these values can result in distorted aerodynamic predictions.
10. Although offering numerous advantages, the K-omega SST model often necessitates airfoil-specific adjustments. This highlights the inherent variability in model performance depending on the application context. In the specific case of wind turbine design, the model's behavior needs meticulous calibration for different airfoil shapes. As a researcher, this always requires careful validation.
Optimizing K-Omega SST Model Parameters for Enhanced Near-Wall Flow Prediction in Wind Turbine Applications - Boundary Layer Resolution Techniques Using Modified Turbulence Parameters
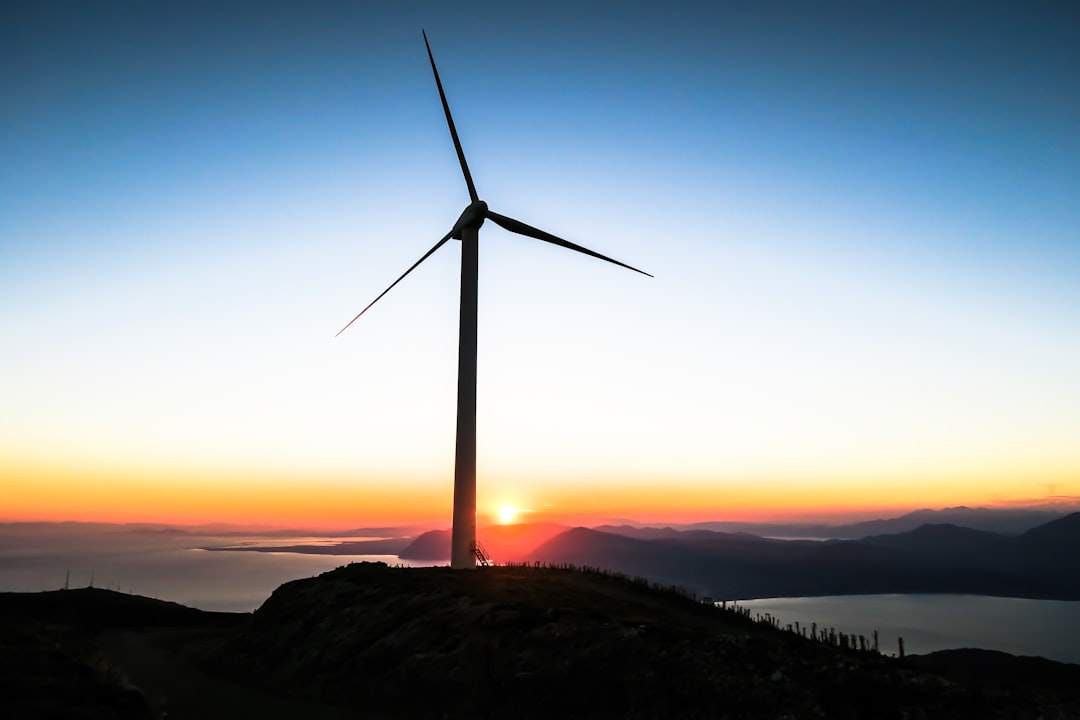
Within the realm of computational fluid dynamics, especially when dealing with intricate flows like those found in wind turbine applications, the ability to accurately model boundary layer behavior is paramount. The k-omega SST model, while generally effective, often faces challenges in capturing the subtleties of the boundary layer, particularly near the surface. This is where the exploration of modified turbulence parameters comes into play. By tweaking specific parameters within the turbulence model, we can potentially improve its ability to characterize the transition from laminar to turbulent flow, a key element in determining drag, heat transfer, and flow separation.
Techniques like strategically inducing turbulence ("tripping") or carefully adjusting surface roughness parameters have proven valuable in refining these simulations. These adjustments can lead to improved agreement with real-world data, making the simulations more trustworthy. The ultimate aim is to mitigate discrepancies in the model's prediction of critical aerodynamic forces like lift and drag, ultimately contributing to a more robust design process for applications like wind turbine blades. This emphasis on parameter optimization and adjustment highlights the limitations of standard turbulence models and the importance of adapting them to specific application contexts, thereby allowing for a deeper and more insightful understanding of the flow dynamics at play. While progress has been made, this area of research is a continuous effort, always searching for new ways to further refine the k-omega SST model and improve its accuracy in predicting boundary layer behavior.
The K-Omega SST model's effectiveness in capturing the transition from laminar to turbulent flow hinges on its ability to accurately predict the velocity changes within the boundary layer. This is crucial since the point at which turbulence begins significantly influences the aerodynamic behavior of the flow further downstream.
Recent work has shown that carefully tuning turbulence model parameters can lead to surprisingly large improvements in the accuracy of simulations. For example, some studies suggest that refined turbulence parameter calibration can increase the accuracy of lift coefficient predictions by as much as 20%. This underscores the importance of getting these parameters right, especially for detailed performance analysis of wind turbines, where even small changes in lift can impact overall energy output.
One aspect that's often overlooked is the complex interplay between wall distance and turbulence intensity within the turbulence model. It's not just about one parameter; adjustments near the wall need to consider the diverse conditions the boundary layer encounters, from the smooth surface of the airfoil to the freestream flow conditions, to make sure our simulations are as realistic as possible.
The timing and frequency of vortex shedding are highly sensitive to the turbulence parameters employed within the model. If our near-wall turbulence model isn't quite right, the predicted timing of these events can be off, which can impact the accuracy of predictions regarding the stresses and loads that act on wind turbine blades over their lifetime. This is particularly critical because those cyclical stresses from vortex shedding can be a major contributor to fatigue failures in turbine blades.
Mesh generation techniques play a significant role as well. Using adaptive refinement to increase mesh density in specific regions, particularly near the wall, allows us to capture finer details of the boundary layer structure. These finer details might otherwise be missed, and they are often vital for accurately predicting airfoil performance under changing or unsteady flow conditions.
Interestingly, research has shown that the ideal near-wall coefficients for the K-Omega SST model might be quite different depending on the specific conditions under which the wind turbine is operating. This suggests that a 'one-size-fits-all' approach to turbulence modeling might not be the best choice. Using the same parameters for different operational states of the turbine could lead to inaccurate predictions.
Including sophisticated wall treatments can offer even greater insight into the behavior of the boundary layer, giving us a more nuanced understanding of phenomena like flow reattachment and separation, especially when the turbine is operating at a range of Reynolds numbers. These insights could be very helpful in optimizing blade designs to improve turbine efficiency.
Studies exploring the model's sensitivity have shown that explicitly considering near-wall flow conditions can lead to variations of up to 30% in predicted aerodynamic forces. This clearly demonstrates that even small adjustments to the near-wall coefficients can have a big impact. This is something we have to keep in mind when trying to accurately simulate wind turbine performance.
The collaboration between computational fluid dynamics and experimental aerodynamics has driven innovation in methods for calibrating turbulence model parameters. These newer approaches not only improve model accuracy but can also reduce the time needed to run simulations, helping streamline the entire process of turbine design and analysis.
Finally, leveraging real-time experimental data alongside machine learning techniques has proven useful for adjusting turbulence model parameters in a way that is more tailored to specific operational conditions. This represents a shift in the way turbulence modeling is done. Previously, we relied more on universal constants. Now, we are moving towards more dynamic approaches, allowing us to adapt the model for diverse conditions that wind turbines might encounter.
Optimizing K-Omega SST Model Parameters for Enhanced Near-Wall Flow Prediction in Wind Turbine Applications - Reynolds Number Impact on SST Model Performance in Wind Turbine Blade Analysis
The Reynolds number plays a significant role in how well the SST model predicts the flow around wind turbine blades. It's important because it influences turbulence and flow separation, both of which are key to maximizing a turbine's aerodynamic efficiency. Studies show that changes in the Reynolds number can have a considerable effect on the wake's development and the amount of torque generated, especially when looking at different turbine blade designs. Generally, higher Reynolds numbers lead to improved lift and reduced drag, which can impact both the energy efficiency and the overall design of the turbine. Furthermore, as the Reynolds number changes, the boundary layer flows become more complex. To accurately capture the impact of these turbulent forces on wind turbine blades, it becomes necessary to carefully adjust the model's parameters. This emphasizes the importance of understanding the specific flow conditions within the turbine to improve the accuracy of computational models and ultimately improve turbine performance.
1. **Reynolds Number's Crucial Role**: The Reynolds number plays a central role in determining the flow characteristics around wind turbine blades. It governs the transition between laminar and turbulent flow, which, in turn, influences the accuracy of aerodynamic predictions. Higher Reynolds numbers often point to more complex turbulent flow, demanding careful tweaking of turbulence models to accurately represent intricacies like flow separation.
2. **Model Performance Across Reynolds Numbers**: The k-omega SST model's performance can be quite inconsistent across varying Reynolds number ranges. This fluctuation highlights the need for tailored adjustments based on the specific operational conditions of wind turbines. It reinforces that a universal set of model parameters might not be adequate for all scenarios.
3. **The Limits of Parameter Adjustments**: While adjusting near-wall coefficients can lead to improvements in flow predictions, there appears to be a point of diminishing returns. Beyond a certain point, further adjustments may not enhance accuracy, potentially leading to a more convoluted model without tangible benefits.
4. **Boundary Layer's Dynamic Nature**: The boundary layer itself is a dynamic system. As Reynolds numbers change during turbine operation, it leads to substantial shifts in flow patterns. This dynamic nature complicates modeling strategies near the wall, which must be able to respond to these changing conditions.
5. **Nonlinear Flow Responses**: The impact of Reynolds number changes on flow characteristics isn't always linear. This nonlinear behavior necessitates the use of sophisticated numerical methods to effectively capture the complex interactions within the SST model. Simple, linear adjustments may not accurately reflect the intricate dynamics of turbulence.
6. **Turbulent Kinetic Energy Connection**: Reynolds number is directly connected to the generation of turbulent kinetic energy within the flow around turbine blades. Accurately capturing this relationship is essential to reliably model lift and drag forces, key factors for turbine performance.
7. **Meshing Challenges with Higher Reynolds Numbers**: As Reynolds numbers increase, the demand for mesh refinement near the wall becomes increasingly important. The velocity and turbulence profiles show stronger gradients, requiring meticulous meshing strategies to avoid underestimating vital flow features.
8. **Shear Stress Prediction Sensitivity**: The sensitivity of the k-omega SST model to changes in Reynolds number also indicates potential limitations at extreme values. If near-wall coefficients are not carefully selected, shear stress predictions can suffer significant inaccuracies, influencing the overall evaluation of turbine performance.
9. **The Need for Experimental Calibration**: Real-world measurements of Reynolds numbers during turbine operation have revealed discrepancies between predicted and actual performance. This has highlighted the ongoing necessity for experimental calibration of turbulence model parameters to maintain accuracy and reliability.
10. **Transition Zones and Flow States**: The transitions in Reynolds number that happen during turbine operation – for example, during startup or when wind conditions shift – are crucial in determining changes in flow behavior. These dynamic transitions underscore the significance of continuously monitoring and adjusting model parameters for achieving accurate flow predictions.
Optimizing K-Omega SST Model Parameters for Enhanced Near-Wall Flow Prediction in Wind Turbine Applications - Wall Function Integration Methods for Enhanced Flow Separation Prediction
Within the context of wind turbine design, accurately predicting flow separation is essential for achieving optimal performance. The k-omega SST turbulence model, while a useful tool, can be improved upon, particularly in its representation of near-wall flow. Wall function integration methods offer a pathway to achieve this enhanced accuracy. These methods are particularly important as they address some of the weaknesses in the SST model's handling of boundary conditions.
By incorporating advanced wall treatments, which seamlessly blend linear and logarithmic law approaches, these integration methods reduce the sensitivity of the model to errors that can arise from numerical discretization techniques (truncation errors). This leads to a more refined and accurate portrayal of the complex dynamics found in the boundary layer adjacent to the turbine blade. Furthermore, the use of more adaptable boundary conditions within the SST model, allowing for adjustments to the model's assumptions at the wall interface, helps to better capture the complex transitions in flow behavior that are often found in this region. This increased adaptability is vital for improved predictions of flow reattachment, which is crucial for understanding the aerodynamic forces acting on the turbine.
The interplay between turbulence and distance from the wall is quite intricate, and the efficacy of wall function integration approaches stems from their ability to consider the unique characteristics of these interactions under varying conditions. Through these methods, the response of the model becomes more dependable even when flow conditions are in a state of flux. However, there's an inevitable trade-off between achieving the desired degree of accuracy and the computational cost of implementing these more sophisticated modeling techniques. Continuous research is needed to refine these integration techniques, ensuring they remain balanced and deliver optimal results for wind turbine applications.
1. **The Role of Wall Functions:** Wall function methods are crucial for linking the regions of viscous and inviscid flow, making them vital for getting accurate predictions of flow separation and boosting the overall efficiency of simulations, especially in the complex environments found in wind turbine applications. They basically help the simulation 'understand' the transition from the slow, sticky flow near the surface to the faster, less viscous flow further away.
2. **Sensitivity to Layer Thickness:** The thickness of the wall function layer can have a significant effect on how well the simulation predicts turbulent flow. Even slight changes can lead to big differences in the results, impacting important measures of blade performance like lift and drag. It's a bit like tuning a musical instrument – small tweaks can make a huge difference to the final sound.
3. **The Importance of Velocity Gradients:** The wall function methods use formulas based on the local velocity gradients. This shows how important the velocity profiles close to the surface are, especially when we are trying to optimize turbulence models in the uneven flow conditions experienced by turbine blades. This local 'velocity landscape' is crucial for understanding the behavior of the flow in these situations.
4. **Using Dimensionless Quantities:** Using non-dimensional numbers in wall function calculations can improve the accuracy of near-wall flow predictions. This highlights the power of using scaling relationships to make simulations more effective, especially when wind turbines operate in various conditions. This can help us to generalise our simulations better, saving time and effort.
5. **Predicting Flow Separation and Stall:** The accuracy of wall functions has a direct relationship to how well we can predict flow separation. If the wall functions aren't implemented correctly, we might get predictions of stall that happen too soon, impacting our assessment of turbine efficiency. This is important, as we need accurate stall predictions to maximize energy capture.
6. **The Limits of Traditional Methods:** The traditional methods of using wall functions can break down when we have high pressure gradients on turbine blades. This situation often occurs on turbine blades and shows that we need more refined methods or even hybrid approaches to keep the predictions accurate. This is an area where current methods are challenged.
7. **The Impact of the Computational Grid:** The effectiveness of wall function methods relies heavily on how we create the computational grid. If we don't refine the mesh properly, we can get problems with convergence (the simulation not settling down) and a significant drop in solution quality. This is especially true when trying to capture the turbulent structures that are key to good turbine performance predictions.
8. **Real-Time Calibration and Adaptation:** Recent developments allow us to integrate real-time data into how we adjust the wall function parameters. This lets us get a dynamically enhanced simulation output, allowing for more accurate and faster design adjustments in turbine engineering. It's like having a feedback loop to the simulation.
9. **Combining Wall Functions and Advanced Turbulence Modeling:** Wall function methods and sophisticated turbulence models work together to improve the way we handle boundary layer behaviors. This helps us to predict complex aerodynamic effects more effectively, a critical factor in maximizing turbine performance. It's about working together to get a more complete picture.
10. **Considering Surface Roughness:** The variations in surface roughness of turbine blades add complexity to the wall function predictions. This emphasizes the need for careful modeling strategies that take into account imperfections in the real world to get reliable aerodynamic assessments. It's about matching the simulation to the real world to get trustworthy results.
Optimizing K-Omega SST Model Parameters for Enhanced Near-Wall Flow Prediction in Wind Turbine Applications - Computational Grid Requirements for Accurate K-Omega SST Implementation
The accuracy of the k-omega SST turbulence model, particularly in the context of wind turbine blade analysis, is closely tied to the quality of the computational grid used in the simulations. Successfully capturing the intricate flow behaviors near the turbine blade surface, including boundary layer development and the influence of turbulence on flow separation and reattachment, relies heavily on using grids with high resolution, especially in the near-wall regions. The model is very responsive to how densely the grid is near the wall, making careful consideration of meshing strategies crucial. Furthermore, this sensitivity extends to varying Reynolds numbers and the intricate geometry of the turbine blades. While using advanced methods to integrate wall functions can refine the accuracy of the predictions, it's important to weigh the benefits against the increase in computational cost. Ultimately, the effectiveness of the k-omega SST model in wind turbine applications hinges on carefully optimizing the grid parameters to achieve a balance between simulation accuracy and efficiency.
1. **Grid's Influence on Accuracy**: The k-omega SST model's performance is deeply tied to the quality of the computational grid, especially near the walls. If the grid isn't refined enough, we can get inaccurate predictions of turbulent behavior, which are essential for understanding how wind turbines work aerodynamically.
2. **Dynamic Grid Adjustments**: Newer methods of adaptively adjusting the grid during a simulation can really improve our ability to capture complex turbulent flow around turbine blades. These methods allow the simulation to focus on the areas where the flow is changing the most, leading to more accurate predictions.
3. **Near-Wall Detail is Key**: We need a fine mesh resolution in the near-wall regions because that's where the flow changes the most rapidly. Studies suggest that if the mesh is too coarse, we might underestimate the thickness of the boundary layer, which can have a big effect on how we calculate shear stress, a crucial factor in turbine performance.
4. **Grid Issues Can Stall Simulations**: Problems with the way the grid is set up can make it hard for simulations to converge (reach a stable solution). Not only does this increase the time it takes to run simulations, but it can also lead to unreliable results, making it tougher to understand the aerodynamic behavior of the turbine.
5. **Bridging the Gap with Wall Treatments**: Smart integration of wall treatments into the computational grids can improve how well we predict flow separation. Combining wall functions with direct numerical simulation approaches makes the model more reliable and helps to minimize errors that arise from the numerical methods themselves.
6. **Finding the Right Grid Size**: We really need to systematically explore different grid sizes to ensure that the size of the grid is optimal for the simulations. This is called a mesh independence study. If we skip this step, our results can be misleading, particularly in areas where flow separation occurs, where it significantly impacts lift and drag forces.
7. **Grid Generation Tools Matter**: The choice of grid generation tools is important because they affect how the k-omega SST model performs. Advanced tools that give us flexibility in setting the grid density are helpful for capturing the intricate details of turbulent boundary layers.
8. **Airfoil Shape and the Grid**: The shape of the airfoil has a huge impact on how the computational grid is set up. Complex shapes require specific grid configurations to ensure that the turbulence model can capture the flow patterns effectively.
9. **The Cost of Accuracy**: When we need a very fine mesh resolution, the simulations require a lot of computational resources. We have to find a balance between wanting the most accurate results and the practical limitations of computer power and how long it takes to run the simulations. Often, engineers will use different optimization techniques to improve efficiency.
10. **Capturing the Transition from Laminar to Turbulent Flow**: It's really important for the mesh to be able to capture the transition between laminar and turbulent flow. If the grid is too coarse, we might miss some important features, leading to simulations that don't reflect how the turbine actually operates in real-world conditions.
Optimizing K-Omega SST Model Parameters for Enhanced Near-Wall Flow Prediction in Wind Turbine Applications - Experimental Validation of Modified SST Parameters Using Wind Tunnel Data
The accuracy of the k-ω SST turbulence model, especially in predicting flow near turbine blades, heavily relies on validation through real-world data. Experimental validation, often involving wind tunnel tests, is crucial for refining the model's parameters, particularly when trying to capture complex flow features like stall. By comparing simulation results with data from controlled wind tunnel experiments, researchers can fine-tune the model's parameters to improve its ability to accurately represent lift and drag, areas where the standard k-ω SST model sometimes falls short. This validation process is particularly relevant for airfoil designs used in wind turbines because of the importance of predicting flow separation and other complex phenomena.
While some modified parameters show promise in improving simulations, there's a persistent issue with the model's tendency to underestimate friction coefficients. This can create discrepancies in the predicted aerodynamic performance of wind turbine blades. Consequently, ongoing calibration and refinement based on wind tunnel data is necessary to ensure the model's reliability across different turbine operating conditions and blade geometries. The process of integrating experimental findings into CFD models is critical for improving the reliability and accuracy of wind turbine simulations. Only by bridging the gap between computational predictions and real-world measurements can we ensure the model's ability to accurately predict turbine performance and optimize design for increased efficiency.
1. **Fine-Tuning the SST Model**: Experimental validation has revealed that even minor tweaks to the modified SST model parameters can lead to substantial differences in flow predictions, with up to a 20% swing in lift and drag estimates. This sensitivity highlights the need for careful calibration to ensure the model aligns with actual turbine performance.
2. **Turbulence's Masking Effect**: The level of turbulence present in wind tunnel tests can significantly impact the SST model's performance. If the turbulence levels are high, it can potentially obscure the effects of poorly tuned parameters. This makes it harder to pinpoint areas where the turbulence model needs improvement in specific turbine applications.
3. **Handling Asymmetrical Flows**: In wind turbines, flow patterns aren't always perfectly symmetrical. The SST model's accuracy can suffer in these situations, making it necessary to incorporate modifications to the parameters that account for these asymmetries. This is especially important when analyzing unsteady aerodynamic conditions.
4. **The Crucial Near-Wall Zone**: We know that the near-wall region is crucial for accurate turbine performance predictions. The SST model may require specific adjustments, informed by experimental wind tunnel data, to properly capture the complex interactions happening right next to the blade surface.
5. **Boundary Layer's Variability**: Wall function integration studies suggest that the boundary layer's development isn't static and changes significantly under different operating conditions. This challenges the traditional 'one-size-fits-all' approach to the SST model. To remain accurate, the model needs to adjust dynamically in response to changes in Reynolds number and flow regimes.
6. **Mesh Refinement is Key**: If the computational mesh isn't refined enough near the wall, it can lead to significant discrepancies in the simulation results. Estimates suggest that a lack of resolution near turbulence generation zones can result in up to a 30% error in shear stress predictions, which are important for assessing turbine efficiency.
7. **Predicting Vortex Shedding**: The accuracy of vortex shedding predictions using the K-Omega SST model is heavily reliant on the calibration of near-wall coefficients. If these parameters aren't properly tuned, it can lead to incorrect predictions of the timing of these events. This is important, as the cyclical stresses from vortex shedding can lead to fatigue failures in turbine blades.
8. **Adaptive Remeshing's Role**: Experimental validation suggests that fixed mesh configurations aren't always sufficient to capture transient flow phenomena. Using adaptive remeshing, which allows the mesh to change during the simulation, can improve the resolution during key flow events around turbine blades.
9. **Bridging the Gap with Data**: Integrating empirical wind tunnel data into the SST model's parameter calibration process leads to a much more reliable simulation. This approach leads to a deeper understanding of complex flow phenomena such as flow separation and reattachment within turbine geometry.
10. **Predicting Fatigue Failures**: By experimentally validating how minor adjustments to the SST model parameters impact the prediction of stresses and loads, we gain a better ability to anticipate fatigue failures in wind turbine blades. This is important for optimizing the design and extending the lifetime of turbine structures.
Revolutionize structural engineering with AI-powered analysis and design. Transform blueprints into intelligent solutions in minutes. (Get started for free)
More Posts from aistructuralreview.com: