7 Engineering Master's Programs Leading AI Integration in Structural Design Through 2025
7 Engineering Master's Programs Leading AI Integration in Structural Design Through 2025 - Stanford Engineering Department New ML-Enhanced Structural Lab Simulates High Rise Design at 85% Less Cost
A new facility within Stanford University's Engineering Department is reportedly utilizing machine learning to simulate high-rise structural designs. The claimed benefit is a substantial reduction in design costs, potentially reaching 85% less than traditional methods. This development underscores the quickening pace of integrating artificial intelligence into established civil and structural engineering processes. These AI-enhanced tools are being explored to improve project efficiency, enhance the optimization of structural components, and contribute to more sustainable building practices. The work conducted at this lab, employing techniques often rooted in neural networks and similar computational approaches, illustrates the expanding influence of AI on design practice and the evolving skillset necessary in the engineering field.
Stanford's Engineering department, specifically within areas like its Structures and Composites Laboratory, appears to be making significant strides in leveraging machine learning for structural analysis. The headline figure of potentially reducing high-rise design simulation costs by 85% is certainly attention-grabbing, and if achievable across varied project types, it suggests a considerable shift in accessibility for advanced simulation techniques. Central to this approach seems to be the application of machine learning algorithms capable of sifting through considerable design data, theoretically enabling engineers to converge on optimized configurations more rapidly than traditional trial-and-error or purely empirical methods.
Crucially, the lab's simulations reportedly extend to modeling structural behavior under significant environmental loads like seismic events and wind, which is fundamental for safety verification and code compliance. The notion of real-time feedback within simulations to quickly adjust parameters sounds promising for compressing traditionally lengthy design iterations that often add considerable time and cost to projects. The reported capability to process complex scenarios far faster than conventional tools—perhaps reducing tasks from weeks to mere days or even hours—is a tangible benefit worth exploring further to understand the computational power and algorithmic efficiency involved.
Furthermore, modeling the nuanced interactions between diverse structural materials offers a more sophisticated understanding than simplified analyses might provide. Exploring design possibilities via generative principles, automatically testing numerous configurations, has the potential to uncover novel, perhaps non-intuitive, solutions that manual processes might overlook. It's interesting that the simulations reportedly consider factors beyond mere load bearing, incorporating aspects like energy performance and material efficiency – moving towards a more holistic design assessment. While collaboration with industry partners is valuable for keeping simulation models grounded in practical relevance and accessing current challenges, one might pause to consider how this relationship influences research priorities and the dissemination of findings. Naturally, exposing engineering students to this kind of AI-enhanced simulation capability equips them with skills rapidly becoming essential for navigating the complexities of modern structural engineering challenges.
7 Engineering Master's Programs Leading AI Integration in Structural Design Through 2025 - ETH Zurich Machine Learning Program Completes First AI Generated Bridge Design in Swiss Alps
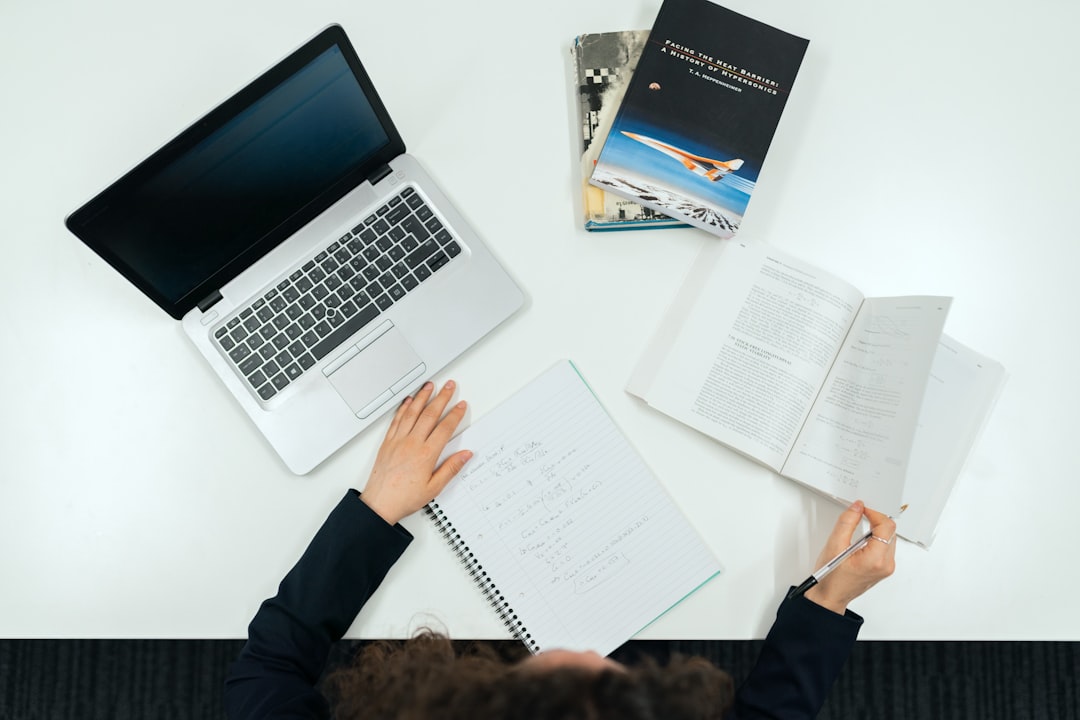
Researchers at ETH Zurich have successfully realized an AI-generated bridge design, reportedly the first of its kind located within the Swiss Alps. This effort sits within a broader movement aimed at embedding machine learning more deeply into structural engineering curricula and practice by 2025. A significant part of the work involves collaboration, including with Swiss Federal Railways, where the focus is on leveraging AI to develop models that can predict the lifespan of existing railway bridges and potentially conserve resources through better maintenance planning.
Furthermore, the program is exploring the use of AI as an assistant for civil engineers during the design phase of new bridges, suggesting a push towards streamlining the initial stages and potentially avoiding costly modifications later in a project's lifecycle. This integration also extends to using AI tools to assess the structural condition of aging bridges, particularly critical railway infrastructure nearing the end of its intended service life, to help prioritize necessary interventions and inspections. While these developments signal promising steps towards enhancing efficiency and safety in bridge engineering, particularly concerning aging infrastructure and initial design workflows, the ultimate reliability and comprehensive validation processes for AI-driven designs in diverse, unpredictable environments like mountain regions remain key considerations as these technologies mature. The ambition is clear: to utilize advanced computational methods to improve outcomes in architecture, engineering, and construction, but the path forward involves navigating the complexities of real-world structural demands and establishing robust trust in algorithmic outputs.
1. Researchers within ETH Zurich's machine learning program have reportedly completed the first design for a bridge structure generated predominantly by artificial intelligence algorithms, intended for a location in the Swiss Alps. This work aimed to demonstrate that AI could not only handle the structural complexities but also explore design spaces that potentially integrate aesthetic considerations alongside pure engineering requirements.
2. The process leveraged generative design techniques, where the AI sifted through numerous potential structural configurations. While computational speed is often cited, the more compelling aspect here is the exploration of design variations potentially beyond traditional parametric or iterative human approaches, raising questions about design innovation.
3. The AI-generated design was reportedly benchmarked against conventional engineering criteria for structural integrity, load bearing, and even environmental impact. This suggests the AI was tasked with mimicking or learning the decision-making heuristics experienced engineers apply, albeit based on available data and defined parameters.
4. The project's significance appears to lie in moving beyond purely theoretical exercises, aiming for a design intended for practical application within challenging real-world conditions like those in the Swiss Alps. This provides a tangible case study for the feasibility of implementing AI-assisted designs in critical infrastructure.
5. This initiative highlights a potential shift from design processes heavily reliant on an engineer's accumulated intuition towards approaches significantly informed, or even primarily driven, by data analysis and algorithmic outputs. This naturally prompts discussion about how the role of the structural engineer evolves from primary designer to validator, interpreter, or supervisor of AI systems.
6. A critical point arising from such projects concerns the necessary validation processes and accountability frameworks. If an algorithm makes design choices, how is reliability ensured, and who is responsible should issues arise? The need for rigorous, transparent validation exceeding current standards seems paramount.
7. Reports indicate a form of feedback loop was incorporated during the design phase, allowing the AI to refine its structural proposals based on ongoing analyses or perhaps simulated performance data. This iterative refinement capability, if robust, could offer new pathways for optimizing designs in response to real-time or simulated conditions.
8. This work at ETH Zurich mirrors a broader trend across engineering education globally, where integrating machine learning and AI tools into the curriculum is seen as essential for equipping future engineers with the necessary skills for an increasingly automated design landscape.
9. Success in such complex projects likely hinges on close interdisciplinary collaboration, specifically between computer scientists developing the algorithms and structural engineers providing domain expertise, validation logic, and practical constraints. This interdisciplinary model seems increasingly vital.
10. The completion of an AI-generated bridge design sets a precedent but simultaneously underscores the necessity for ongoing, perhaps more urgent, dialogue within the engineering community regarding the ethical implications of deploying AI in critical infrastructure where safety and public trust are non-negotiable.
7 Engineering Master's Programs Leading AI Integration in Structural Design Through 2025 - MIT Cross Platform Hub Links 1500 Global Structural Engineers Through Neural Network Project
MIT is reportedly undertaking a significant project aimed at fostering connectivity among structural engineers globally. Termed the "Cross Platform Hub," this initiative is said to link over 1,500 professionals worldwide, partly through leveraging a neural network project. The stated purpose is to enhance collaboration across the field. Parallel to this network effort, the university is emphasizing artificial intelligence integration within seven of its engineering master's programs, targeting a more widespread incorporation of AI in structural design practices by 2025. While connecting a large group of engineers is an ambitious step, the true measure of success will be whether this digital framework and its underlying neural network lead to concrete advancements in how structures are actually designed and built, rather than simply facilitating communication channels. The emphasis on integrating AI into the curriculum through these master's programs certainly aims to prepare future engineers, but the practical outcomes of the "Hub" itself on real-world structural engineering challenges remain to be demonstrably proven.
The MIT Cross Platform Hub reportedly links over 1,500 structural engineers from around the world via a neural network project. The stated aim is to facilitate collaboration, potentially accelerating the exchange of knowledge and insights compared to established, often much slower, methods.
This neural network initiative is exploring machine learning applications beyond just refining structural forms; it appears to be looking into real-time monitoring of asset condition, potentially enabling earlier detection of performance deviations before they escalate into significant issues. Engineers utilizing the platform have apparently noted faster project progress, suggesting that accessing shared information and collective experience might indeed streamline certain aspects of design and decision-making, perhaps highlighting existing disconnects in traditional workflows.
The system is said to employ algorithms capable of processing extensive datasets drawn from various projects. The idea is that this can reveal underlying trends or unusual patterns not readily discernible through conventional examination, potentially bolstering predictive maintenance strategies. A particularly interesting feature mentioned is a mechanism allowing engineers to feed back project outcomes into the system, ostensibly refining the underlying algorithms based on actual structural behavior over time.
The platform is also noted for its capacity to connect engineers from diverse backgrounds—civil, mechanical, and others—on complex problems, raising the prospect of novel integrated approaches that might not emerge from discipline-specific silos. A critical consideration for such a system, however, remains the management of sensitive data; balancing the benefits of shared knowledge with the necessity of protecting proprietary information is clearly a significant ongoing challenge, prompting important ethical discussions within the profession.
The hub reportedly also serves as a repository for analyzing past structural failures, transforming them into shared case studies. This suggests an effort to institutionalize learning from mistakes, making lessons widely accessible rather than remaining isolated within individual firms or regions. Furthermore, the platform is presented as a means to explore generative design possibilities, allowing engineers to computationally investigate a broader spectrum of design alternatives than might typically be feasible, with the potential to uncover innovative structural typologies. Consequently, this initiative is said to be influencing academic programs, signaling a potential shift in how future engineers might be trained to operate within increasingly connected and computationally augmented environments.
7 Engineering Master's Programs Leading AI Integration in Structural Design Through 2025 - NTU Singapore Students Deploy Earthquake Response AI System During April 2025 Taiwan Event
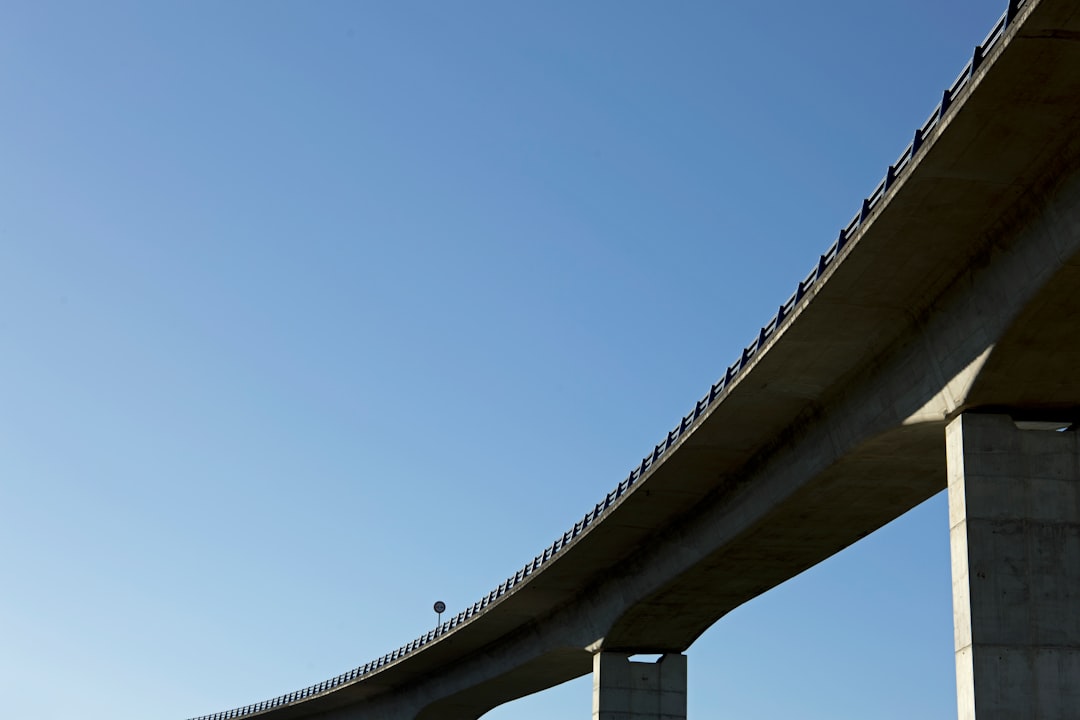
In April 2025, students affiliated with Nanyang Technological University (NTU) Singapore reportedly deployed an artificial intelligence system intended for earthquake response during a seismic event that affected Taiwan. This initiative falls within the broader context of university efforts, including those at NTU, to incorporate AI applications into engineering curricula, particularly for fields like structural design and disaster preparedness. The deployment in a real-world scenario highlights the ongoing drive to move AI from theoretical study to practical application in critical areas. NTU Singapore has been identified as a key institution integrating AI into its engineering programs through 2025, aiming to prepare graduates for an evolving industry. However, the actual performance and limitations of such AI systems when faced with the unpredictable complexities of a major earthquake require thorough evaluation beyond the initial deployment phase.
During the significant seismic activity near Taiwan in April 2025, engineering students from NTU Singapore reportedly deployed an artificial intelligence system aimed at aiding earthquake response efforts. Initial accounts suggest the system focused on analyzing real-time data to rapidly assess structural integrity, potentially offering a much quicker initial assessment compared to solely relying on traditional manual inspection methods.
This deployment seemingly involved working alongside relevant local response entities, providing the students an opportunity to apply theoretical knowledge in a dynamic, high-stakes emergency setting – bridging a notable gap between academic study and practical disaster scenarios.
The AI system was described as being capable of processing diverse sensor inputs, including data typically generated by accelerometers and strain gauges installed on structures, consolidating this information to build a picture of building conditions. This indicates an exploration into leveraging multi-source data streams for structural evaluation under stress.
An interesting reported capability was the AI's programming to identify specific data patterns potentially indicative of impending or current structural failure, raising questions about the system's ability to potentially augment, or in rapid situations, potentially streamline the initial diagnostic tasks often left solely to expert human judgment during a crisis.
Furthermore, there was mention that the system utilized algorithms designed to forecast potential aftershocks based on characteristics of the initial tremors. If reliable, integrating such predictive analytics could offer a valuable layer to public safety protocols and coordinated evacuation efforts in seismically active urban environments.
The project reportedly benefited from an interdisciplinary approach, with students from various engineering backgrounds collaborating to refine the AI's functionalities, highlighting the increasing necessity for diverse technical perspectives when developing complex AI tools for real-world applications.
The real-time data processing and subsequent updates provided by the AI system suggest a move toward more dynamic structural health monitoring capabilities, particularly crucial in unfolding situations where conditions can change rapidly, potentially offering continuous situational awareness rather than relying on static assessment snapshots.
Sources indicated that the AI's suggestions for immediate structural actions were informed by historical seismic data and machine learning models trained on information from past earthquakes. This points to the value being placed on leveraging historical context and pattern recognition for informing critical, time-sensitive decisions during an emergency.
Using a live seismic event for this type of AI system deployment would undoubtedly provide students with a profound, albeit challenging, learning experience, pushing them beyond typical coursework into areas like crisis management and rapid problem-solving under pressure – skills perhaps not always sufficiently emphasized in conventional engineering programs.
Finally, subsequent discussions around this deployment naturally circled back to the significant ethical considerations inherent in depending on AI systems for critical decisions in disaster scenarios, particularly concerning clear accountability if algorithmic errors occur and the need for transparency in how these systems arrive at their structural assessments.
More Posts from aistructuralreview.com: