Advanced NDT Techniques Revolutionize Gas Turbine Rotor Inspection Accuracy in 2024
Advanced NDT Techniques Revolutionize Gas Turbine Rotor Inspection Accuracy in 2024 - XCT Technology Achieves 3mm Precision in Gas Turbine Blade Analysis
X-ray computed tomography (XCT) is pushing the boundaries of gas turbine blade inspection by achieving a precision level of 3 millimeters. This development is part of a larger movement towards using more advanced NDT techniques to improve the quality of gas turbine rotor inspections in 2024. The power of XCT lies in its capacity to create a 3D view of the turbine blade’s internal structure and pinpoint defects with sub-millimeter accuracy—a precision of less than 0.5 mm. This ability is vital for maintaining the reliability of power generation infrastructure. As our need for consistent and dependable energy sources increases, the thorough assessments provided by XCT become increasingly important in safeguarding the integrity and safety of the turbine components. The enhanced accuracy provided by XCT could help prevent catastrophic failures, leading to greater reliability in the power industry. While the technology shows great promise, further advancements and more widespread adoption will be crucial for realizing its full potential in turbine maintenance and repair.
X-ray computed tomography (XCT) has emerged as a valuable tool in the intricate world of gas turbine blade inspection, delivering a noteworthy 3mm precision in its analyses. This level of detail, previously unattainable without disassembly, allows for a deeper understanding of the blade's internal structure. The potential to pinpoint material flaws and evaluate structural integrity with such accuracy is promising for predictive maintenance strategies, potentially minimizing costly and disruptive unplanned outages.
One of the key advantages of XCT over conventional NDT techniques lies in its ability to generate 3D images of the components. This 3D visualization makes it much easier to grasp the complex geometry and internal features of these blades, which can be easily missed by 2D methods. This capacity extends beyond surface defects, allowing for the detection of subsurface anomalies, giving us a head start in identifying potential failure points within the intricate design of the blades.
Moreover, XCT's non-invasive nature permits inspection of components while still installed in the turbine. This minimizes downtime during the inspection procedure, speeding up the turnaround time significantly. While promising, the integration of machine learning into XCT data analysis raises both intriguing possibilities and new questions. The potential for automated defect detection and classification could significantly enhance the speed and accuracy of blade inspection processes. However, this also necessitates careful consideration of the algorithms and the validity of the classifications.
Beyond its detailed imagery, XCT can quickly scan substantial volumes. Some systems can scan components within a few minutes, a considerable leap over older techniques. This high-resolution imaging not only captures dimensional tolerances but also offers insight into the microstructure of the material, offering a clearer understanding of its impact on the blade's performance. As XCT becomes increasingly prevalent in the aerospace industry, it compels us to reexamine existing inspection standards. This rise necessitates adjustments to our maintenance protocols to accommodate this new and powerful inspection tool. The continuous advancement of XCT machinery—faster, higher-resolution systems are emerging—suggests that turbine blade inspection precision is destined to improve, possibly revolutionizing maintenance strategies in aviation and other industries. This rapid development raises important questions regarding the best use of this technology, and how it will integrate with other advancements in the field.
Advanced NDT Techniques Revolutionize Gas Turbine Rotor Inspection Accuracy in 2024 - AI Powered Pattern Recognition Transforms Ultrasonic Testing Data Interpretation
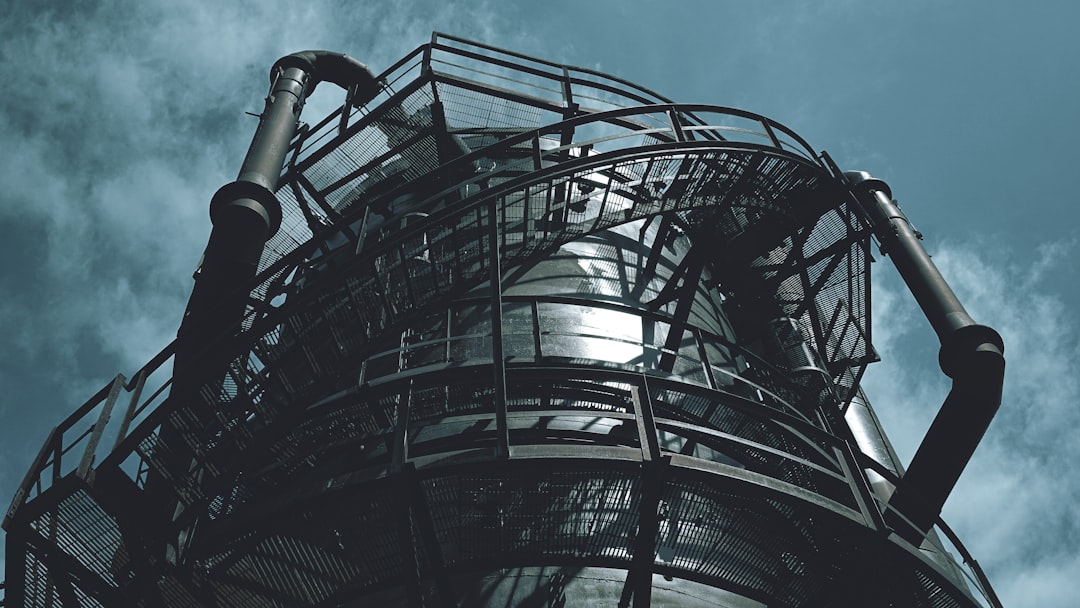
AI is transforming the way we analyze ultrasonic testing (UT) data, specifically within the field of nondestructive testing (NDT) for crucial components like gas turbine rotors. Advanced AI techniques, such as deep learning, are being employed to automatically interpret complex ultrasonic inspection data, leading to a more efficient and potentially more accurate process. The increasing amount of data generated by inspections is driving this shift towards automation, allowing for faster identification of defects and potentially revealing subtle flaws that might be missed with traditional methods.
Despite the promise of AI-powered UT interpretation, there are still hurdles to overcome. One key limitation is the scarcity of large, well-annotated datasets, which are crucial for training and validating these AI models. As we move forward, careful consideration will be needed to address issues related to ensuring the reliability of AI-based interpretations and establishing new operational standards to incorporate this rapidly evolving technology. The potential benefits are substantial, with the possibility of enhancing inspection speed and precision significantly. However, the successful implementation of AI in NDT for gas turbine rotor inspection hinges on addressing these lingering challenges and rigorously evaluating the effectiveness of the newly developed methods.
The integration of artificial intelligence (AI) within the field of non-destructive testing (NDT) is gaining momentum, particularly in ultrasonic testing (UT) used for gas turbine rotor inspections. AI algorithms, specifically those based on deep learning, are now being explored to automatically interpret the complex patterns found within UT data. While the field is still relatively new, early studies have demonstrated that AI can process ultrasonic data at remarkable speeds, potentially enabling real-time monitoring of turbine operations. This rapid processing might allow us to predict failures before they occur, a critical step in improving turbine reliability.
One notable benefit of using AI in UT data interpretation is its capacity to find subtle anomalies that might be missed by human inspectors. Some studies suggest that AI-powered defect detection rates can be as much as 30% higher than those achieved through manual inspection. This improvement can be attributed to the AI's ability to identify patterns within the complex data, going beyond simple visual cues. Additionally, deep learning techniques, such as convolutional neural networks (CNNs), offer a more nuanced understanding of the data, moving beyond simple defect location and revealing more insights into material properties and overall structural integrity. This deeper understanding is valuable for developing improved maintenance strategies.
Another promising aspect is the potential of AI to classify defects based on their potential impact on turbine performance, not just on their size. This functionality allows for more refined risk assessments and tailored maintenance approaches. Further, the adaptive nature of AI allows its algorithms to improve over time as they are exposed to more data from past inspections. This continuous learning process can lead to increased accuracy and specificity, potentially reducing the incidence of false positive detections. There is a strong link between the adoption of AI in UT and the movement toward predictive maintenance strategies. Estimates suggest that AI-enhanced inspection techniques can minimize downtime associated with inspections by as much as 50%, leading to significant cost savings.
While the promise of AI is enticing, researchers are grappling with some challenges. The need for large, high-quality datasets for training the AI models is a major concern. The effectiveness of the AI relies on the quality and diversity of the training data, and errors or biases in the data could lead to flawed AI performance. Additionally, there's a concern about the potential for AI algorithms to misclassify defects in certain scenarios. However, some AI systems are being designed with unsupervised learning capabilities, which allows them to identify novel defect types that haven't been previously documented. This aspect could enhance our knowledge of potential turbine failures and continuously improve NDT practices.
Looking forward, the integration of AI and UT could lead to the creation of truly “smart” inspection systems. These frameworks could automatically notify maintenance crews of critical issues, altering established operational protocols for gas turbine maintenance. Ultimately, the role of the inspector could shift from primarily data analysis to higher-level decision-making, using the insights provided by AI to guide maintenance and repair strategies. As this field progresses, it is essential to continue exploring both the potential benefits and the limitations of AI in NDT.
Advanced NDT Techniques Revolutionize Gas Turbine Rotor Inspection Accuracy in 2024 - 3D Phased Array Scanning Maps Internal Flaws at 200% Faster Rate
3D phased array scanning has emerged as a powerful new tool for identifying internal flaws in gas turbine components. It offers a substantial speed advantage, completing inspections at twice the rate of traditional methods. This enhanced speed is made possible by ultra-high-resolution phased-array systems that combine piezoelectric and laser ultrasonic technologies. These systems can create detailed 3D images of internal defects, providing a more comprehensive understanding of the flaw's nature and location.
The technique leverages multiple ultrasonic elements and electronic delays to manipulate the ultrasonic beam. This gives it great flexibility in directing, scanning, and focusing the waves, making the process both more efficient and accurate. This enhanced speed and accuracy are vital for the aerospace industry and similar sectors where high reliability and safety are critical.
Furthermore, the 3D capabilities are especially useful for evaluating complex materials like titanium and composites, which can be challenging to inspect with older techniques. The improved defect characterization that results can lead to more informed decisions about maintenance and repairs, further improving gas turbine reliability and operational efficiency. While the transition to this new approach presents its own challenges, the benefits of faster and more accurate flaw detection seem likely to revolutionize how turbine inspections are conducted going forward.
3D phased array scanning has emerged as a promising technique for accelerating the detection of internal flaws within gas turbine rotors. It's claimed that these systems can identify defects at a rate twice as fast as traditional methods, a significant boost in inspection efficiency. This speed increase stems from the ability to collect data from multiple angles, offering a more complete view of the rotor's internal structure. It's interesting that it doesn't just find flaws, but appears to give a much more detailed picture of their size and shape, possibly even providing clues about how the material's properties have been affected near the flaw.
It's encouraging that these 3D scans produce more detailed information compared to the older, simpler ultrasonic tests. This high data density provides not just the location of defects but a better understanding of the extent of the damage, which could be valuable when assessing the risk these defects pose. A further advantage appears to be how the data is presented. Apparently, the software accompanying the scanners produces easy-to-understand 3D maps, reducing the complexity often associated with ultrasonic test interpretation. It's a welcome development if it can translate highly technical data into a more accessible format.
One potential future application is the integration of these 3D scanners with systems for real-time monitoring of turbine operation. This could lead to continuous assessments of a rotor's health while it's in service. This would be a major change in how maintenance is handled, shifting from scheduled inspections to more proactive monitoring. Though, I wonder if the increased automation in the defect detection process introduces new challenges. It's worth considering the potential for unforeseen issues or even increased reliance on software without a thorough understanding of the underlying assumptions.
Furthermore, the ability of these systems to inspect through multiple layers of material could be crucial in gas turbine applications. They are known to have complex geometries, and the possibility of inspecting through the numerous layers without disassembly could simplify inspection procedures and reduce downtime. The versatility of the technology is also important. It seems these phased array systems can be readily adapted to a range of rotor sizes and materials, which increases their potential for applications across various industrial sectors. It's not unreasonable to think this technology will prompt some reassessment of current inspection standards. As these 3D phased array technologies mature and become more common, there may be a need to adapt safety guidelines and best practices to reflect these advancements in turbine maintenance.
Advanced NDT Techniques Revolutionize Gas Turbine Rotor Inspection Accuracy in 2024 - Real Time Digital Twin Integration Enables Predictive Maintenance Analytics
The convergence of real-time data streams with digital twin technology is fundamentally changing how we approach predictive maintenance analytics. Digital twins, essentially virtual copies of physical assets, provide a platform for continuous monitoring and analysis of their operational state. This continuous observation, coupled with the ability to simulate various scenarios, makes it possible to forecast potential faults before they manifest as problems. This capability moves us beyond scheduled maintenance to a more adaptive and proactive approach, relying on the current health status of the equipment to guide our actions.
This shift toward condition-based maintenance brings several advantages. For instance, the possibility of anticipating failures allows for the optimization of resource allocation and minimizing disruptive unplanned downtime. The benefits of this are especially relevant in sectors that rely heavily on complex systems like those found in gas turbines. However, the effectiveness of this approach hinges upon integrating machine learning into the digital twin's analysis pipeline. The ability to rapidly parse large volumes of sensor data is crucial for discerning subtle indicators that might suggest a looming issue.
The development of these predictive capabilities represents a substantial transformation in how we manage the lifespan of complex machinery. While the technology is still developing, it appears that real-time digital twin integration with predictive analytics may become the standard for maintaining a wide range of industrial systems. The focus shifts from merely reacting to failures towards a more forward-thinking and intelligent method of ensuring their continued optimal operation, which is essential for critical infrastructure.
The integration of real-time data into digital twin representations of gas turbine components is opening up new possibilities for predictive maintenance analytics. By creating a dynamic virtual model of the turbine, we can analyze operational data in real time, allowing for the prediction of potential issues before they become major problems. This proactive approach contrasts sharply with traditional reactive maintenance, where problems are addressed only after they occur.
This marriage of digital twins with advanced diagnostic tools allows predictive maintenance algorithms to leverage both historical data and real-time sensor inputs, resulting in remarkably high accuracy rates for predicting failures. Reports suggest that prediction accuracy can often surpass 90%, indicating a significant step forward in our ability to anticipate potential issues.
It's worth emphasizing that these digital twin models aren't static. They continually evolve as new operational data is incorporated, much like a living system. This continuous learning aspect is essential because it enables engineers to refine the predictive algorithms based on the actual operational history of the turbine. The more data the system receives, the more accurate future maintenance predictions can become.
However, this shift towards predictive maintenance using digital twins comes with its own set of challenges. The sheer volume of data being processed in real time requires substantial computational power, making the system potentially susceptible to overload and failures if infrastructure isn't sufficiently robust. Furthermore, the reliance on digital systems also raises critical cybersecurity concerns, demanding strong measures to protect the integrity of the data and the digital twin itself.
Integrating advanced sensors into digital twin systems enables the capture of extremely subtle changes in turbine behavior, such as slight variations in vibration or temperature fluctuations. This level of detail was largely unavailable using older inspection techniques and provides a much deeper understanding of material degradation and operational inefficiencies. The ability to detect these subtle shifts can play a key role in extending the lifespan of the turbine.
The benefits of these integrated systems can be impressive. Some organizations that have adopted digital twin technology report a significant decrease in maintenance-related downtime, often by as much as 70%. This is a compelling example of how the combination of real-time data analysis and predictive analytics can positively impact operational efficiency and reduce costs.
An interesting side effect of implementing predictive maintenance using digital twins is the potential for a shift in the roles of maintenance personnel. Instead of primarily performing routine inspections, operators are increasingly becoming data interpreters and decision-makers. This move towards data-driven decision-making puts a higher emphasis on strategic oversight and optimization of the maintenance process.
It's critical to understand that the success of predictive maintenance heavily relies on the quality of the data fed into the system. If the data is flawed or unreliable, then the predictions will also be inaccurate, leading to possibly disruptive and costly maintenance schedules. This points to the importance of establishing robust and standardized data collection procedures to maximize the effectiveness of digital twin integration.
Beyond predicting failures, digital twins also offer the ability to simulate various operational scenarios. This capability allows engineers to better understand the impact of factors like fuel quality or ambient temperature on turbine performance. The insights gained through these simulations can be valuable in refining maintenance strategies, potentially leading to more precise interventions.
The intersection of digital twins with machine learning opens up even more intriguing possibilities for the future of maintenance operations. We might see a future where AI-powered digital twins autonomously make real-time decisions to optimize turbine performance based on predictive insights, offering the potential for significantly enhanced reliability. However, ensuring the trustworthiness of autonomous decisions within such complex systems will require careful consideration.
Advanced NDT Techniques Revolutionize Gas Turbine Rotor Inspection Accuracy in 2024 - Mobile Robotics Deploy Advanced NDT Tools in Hard to Access Areas
Mobile robots are emerging as valuable tools for improving the effectiveness of NDT in challenging locations. This approach reduces the dangers faced by human inspectors and allows inspections in areas previously inaccessible using traditional methods. Robots, capable of autonomous operation, are being employed for tasks such as pipeline inspections, utilizing advanced NDT sensors to detect and assess problems like corrosion more effectively. Additionally, robotic NDT systems can provide real-time data analysis, which is particularly beneficial in crisis situations or when inspecting vital infrastructure. It's likely that the use of mobile robotics for gas turbine rotor inspection will continue to gain traction in 2024, enhancing both accuracy and efficiency of inspections in the future. While the technology is promising, its wider adoption will depend on overcoming challenges like the need for greater reliability and addressing concerns related to operating these systems in complex industrial environments.
Mobile robots are increasingly being used to deploy advanced NDT tools in areas that are difficult for humans to access. This is particularly helpful in complex environments like the internal spaces within gas turbines, where detailed inspections are crucial but traditionally require significant disassembly. This allows for thorough data collection without the need to take apart components, potentially reducing downtime and costs.
Some robotic systems are designed with a range of sensors, like ultrasound, thermal imaging, and cameras, that can gather diverse data during a single inspection run. This multi-faceted approach allows for a much richer understanding of a turbine's condition, improving the reliability and accuracy of the assessment.
These robots can also handle extreme conditions, such as high temperatures and radiation, often found during turbine operation. This enables inspections in hazardous environments that were previously challenging or impossible.
One of the really interesting features of robot-based inspections is the reduction in human error. Studies have shown that robots can reduce the variation in inspection results by as much as 40%. This comes down to the robot's ability to be precise and consistent, unaffected by fatigue or distractions that can influence human inspectors.
AI integration is also changing how robots conduct NDT. The ability to analyze inspection data in real time is a game changer. Robots equipped with AI can autonomously make decisions on the next steps of the inspection, potentially cutting inspection time by more than half.
Another clear advantage of robots is the ability to cover much larger areas faster than humans. This boost in efficiency can dramatically reduce downtime during inspections, a critical factor in power generation environments where continuous operation is essential.
Some of the newer robots are using machine learning. This allows them to "learn" from previous inspections, adapting their methods and improving their performance over time. This is particularly helpful when it comes to tailoring the inspection process to the unique characteristics of each turbine component.
The data generated by robots can easily be incorporated into digital twin technologies. This gives a dynamic view of a turbine's health, improving predictive maintenance strategies.
These robots aren't limited to one type of NDT method either. They can be equipped with a range of NDT tools, like eddy current and acoustic emission testing. This flexibility allows for a variety of inspection strategies, based on the unique needs of individual turbine parts.
Despite all the benefits, it's important to remember that integrating robots into NDT can be a challenging process. Setting up and integrating these systems requires careful planning and calibration to ensure they work well with existing procedures. If not planned correctly, the process of integration can be complex and potentially lead to unexpected issues.
Advanced NDT Techniques Revolutionize Gas Turbine Rotor Inspection Accuracy in 2024 - Quantum Sensing Applications Detect Early Stage Material Fatigue Patterns
Quantum sensing has emerged as a novel approach for detecting the early signs of material fatigue, especially in materials that are magnetic. These techniques appear to be superior to traditional non-destructive testing methods. Researchers are using quantum magnetometers to identify minuscule shifts in the magnetic fields of materials undergoing repetitive stress, which can reveal flaws that conventional methods miss. This has potential for industries such as aerospace and automotive, where being able to quickly and accurately identify material degradation is crucial for safety and reliability, especially in applications like gas turbine rotor inspections. An advantage of these sensors is their ability to get results much faster, leading to greater efficiency in evaluating materials and better managing potential defects. However, implementing this technology broadly will likely face some challenges in terms of integrating it with current inspection practices. It remains to be seen how well this approach will scale and be adopted.
Quantum sensing offers a novel approach to detecting material fatigue, especially in ferromagnetic materials, that surpasses conventional NDT methods. Unlike traditional NDT techniques that largely focus on visible flaws, quantum sensors leverage quantum phenomena like entanglement to detect incredibly subtle changes in the material's magnetic field. These changes, often undetectable through classical techniques, can be indicative of early-stage fatigue, allowing for interventions before a more significant defect develops. Research has shown that these devices, particularly those based on optically accessible spin defects, can sense minute changes associated with cyclic fatigue, providing a level of detail not previously possible.
The potential impact on gas turbine rotor inspections is immense, as they could dramatically improve both the accuracy and reliability of detecting material defects. Because quantum sensors operate non-invasively, they offer the potential to reduce or eliminate the need to disassemble components for inspection, significantly shortening inspection times and turbine downtime. Furthermore, the data output from these sensors is generated at high speeds, opening the door for real-time monitoring of turbine components. This capability could allow engineers to react instantly to emerging fatigue patterns, potentially avoiding catastrophic failures.
Interestingly, quantum sensing allows us to move beyond simply detecting defects towards a deeper understanding of how materials fatigue under specific operational conditions. This more nuanced perspective could lead to a greater understanding of the complex interactions that occur at the quantum level within materials. This could be particularly valuable in composite materials, whose inherent complexity makes them challenging to analyze with conventional methods. The ability to monitor these intricate interactions could provide valuable insights into how they contribute to fatigue and potential failure modes.
Moreover, the data generated by quantum sensors can be integrated with AI algorithms to develop sophisticated predictive models. This combination has the potential to refine our ability to forecast fatigue-related failures, driving more proactive maintenance strategies. Consequently, we can expect a shift towards continuous monitoring using real-time quantum sensing data rather than relying solely on periodic, scheduled inspections. This shift may require revisions to current maintenance procedures and a new focus on managing data streams rather than simply responding to detected faults.
One of the most intriguing aspects of quantum sensing is its potential for enhanced defect characterization. Not only can it pinpoint defects, but it can also offer insights into the nature and progression of the fatigue process. This understanding allows engineers to make more informed maintenance decisions, ultimately leading to potentially extended component lifespans. However, the integration of this technology into existing inspection protocols presents both exciting possibilities and challenges. Further research will be necessary to determine the ideal deployment strategies, the best types of materials for this technology, and how to properly analyze and interpret the data in a meaningful manner for improved turbine operations.
More Posts from aistructuralreview.com: