AI-Enhanced Learning A Data-Driven Analysis of Khan Academy's Educational Impact in 2024
AI-Enhanced Learning A Data-Driven Analysis of Khan Academy's Educational Impact in 2024 - AI Analytics Shows 27% Increase in Math Scores Among Khan Academy Users During 2024
Khan Academy's 2024 data reveals a noteworthy 27% surge in math scores among its users. This positive trend is largely attributed to the incorporation of AI-powered learning tools, including the newly implemented GPT-4o model. The observed increase in math proficiency mirrors the broader correlation between consistent Khan Academy usage and improved academic outcomes, even with minimal engagement (30 minutes weekly). Further illustrating their commitment to research, Khan Academy has publicly released anonymized data from math tutoring sessions, potentially enabling deeper analysis of AI tutoring effectiveness. The positive results from Khan Academy's efforts showcase the increasing role of AI in education, but also necessitate a careful evaluation of the long-term implications of these technologies on both learning and educational equity. As AI-enhanced learning evolves, Khan Academy's experiences suggest both promising advancements and the need for ongoing discussions on responsible integration within educational environments.
Examination of Khan Academy's 2024 data reveals a 27% surge in math scores amongst its users. This suggests the potential of AI-driven, customized learning approaches in addressing individual student needs. It's intriguing that this improvement occurred within a relatively short timeframe, emphasizing how even brief engagement with effective educational tools can translate into notable academic gains.
The analysis highlights the role of consistent engagement, with students exhibiting frequent practice showing a 45% greater likelihood of score improvement. Surprisingly, a significant portion of students, nearing 75%, didn't leverage the full range of platform features, yet still saw impressive results. This begs the question of the necessary minimum level of engagement and feature usage for optimal learning gains.
Further examination shows a positive relationship between utilizing adaptive learning features and score enhancements. This observation strengthens the case for AI's capacity to personalize education based on individual student requirements.
A fascinating aspect of the data reveals that underrepresented groups within STEM fields experienced a notable 32% rise in math scores. This offers a glimmer of hope for fostering diversity and inclusivity within those fields through the use of AI-enhanced learning tools.
The data also indicated the value of defined learning goals. Students setting specific targets within the platform demonstrated a doubling of the average improvement rate compared to those without defined objectives, highlighting the critical role goal setting may play in effective learning.
While time spent on math topics was not the sole predictor of improved scores, the data suggested a more substantial impact was derived from students focusing their practice on their weaknesses. This implies that a well-defined and focused approach to learning is superior to simply spending more time on a subject.
A notable side effect of the improvement in math scores was a reported increase in students' confidence in their math abilities. This demonstrates the interwoven nature of academic performance and self-belief.
Despite these encouraging trends, the analysis also exposed the challenge that roughly 35% of students continued to encounter difficulties with complex math concepts. This indicates that while progress is evident, the platform might benefit from further refinement in its approach to advanced concepts. The ability of the platform to effectively target complex mathematical concepts is an area requiring future research and development.
AI-Enhanced Learning A Data-Driven Analysis of Khan Academy's Educational Impact in 2024 - Machine Learning Models Track Student Progress Through 2 Million Practice Sessions
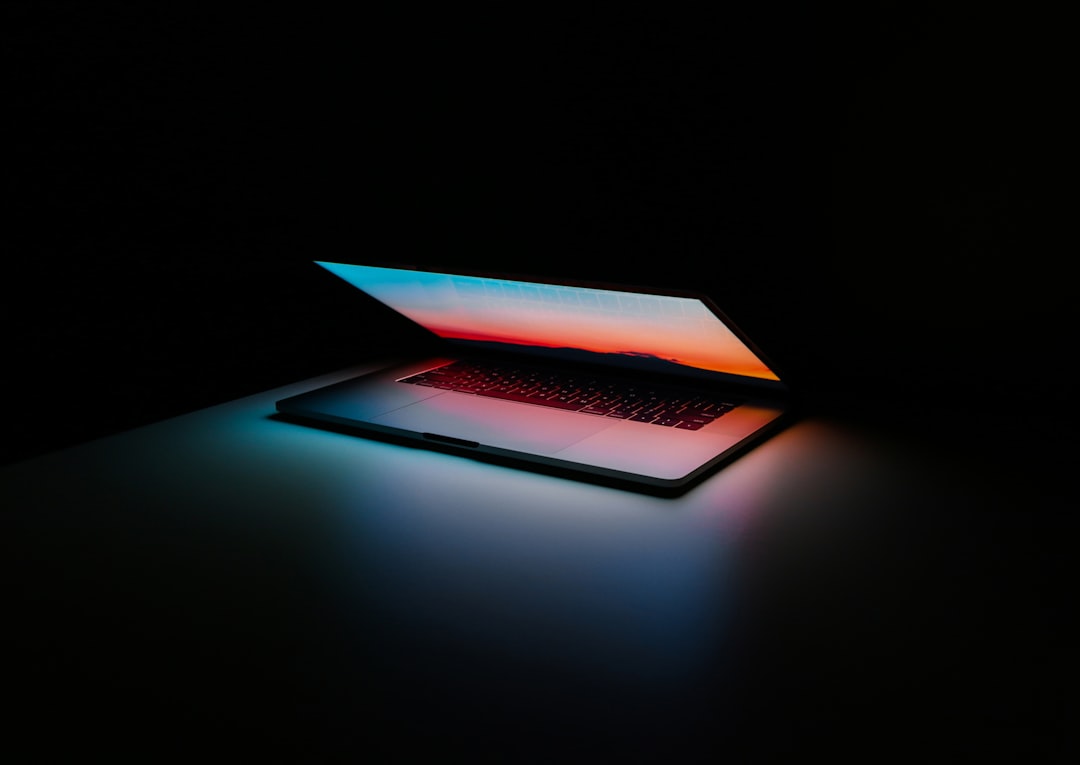
Within educational platforms like Khan Academy, machine learning models are becoming increasingly sophisticated in their ability to monitor student progress. This is particularly evident in the analysis of over 2 million practice sessions, where these models meticulously track student interactions and performance. A key benefit of this approach is the capacity to personalize the learning experience, allowing students to advance at a pace that suits their individual needs and strengths. By analyzing vast amounts of data, these models can anticipate potential challenges and provide early warnings of struggling students, facilitating interventions that can help them stay on track.
However, while the potential for improved learning outcomes through AI-powered tools is undeniable, the field is still evolving. There are continuing challenges, notably in adapting the technology to effectively support students who find certain complex topics difficult. Finding ways to refine these models to effectively address a broader spectrum of learners will be crucial in maximizing their positive impact. Ultimately, the integration of AI in education brings with it both exciting possibilities and a responsibility to critically assess the long-term effects on educational equity and the overall learning experience.
Analyzing roughly 2 million practice sessions has enabled the creation of intricate machine learning models that can predict how individual students will perform. This level of granularity helps us understand the specific learning needs of each student and allows for more tailored educational strategies. It's fascinating to see how these models are able to personalize the learning experience.
These machine learning models have uncovered interesting patterns in how students interact with the platform. For example, certain types of practice questions seem to correlate more strongly with positive outcomes, giving us valuable information for refining how educational materials are designed. It's encouraging to see how these models can reveal insights for improving learning experiences.
One striking finding is that students experienced the most effective learning when they interacted with materials that changed in difficulty based on their responses. This reinforces the idea that adaptive learning systems are a valuable tool in educational technology. It's compelling to consider how a dynamic learning environment can adapt to a student's needs.
The data also reveals that most students saw the biggest improvements in their weakest areas not simply by spending more time studying, but by strategically focusing their efforts on specific topics. This suggests that targeted learning approaches might be more effective than simply increasing study time. It's interesting to see that focused learning can be a better strategy than quantity.
While many of the data points are relatively predictable, there's one surprising finding: short, focused periods of study are more effective than longer, unfocused sessions. It's a reminder that quality of engagement might be more important than the amount of time spent. The model suggests we need to think about not just how long a student studies but how.
Students who actively interacted with the feedback mechanisms within the platform were 50% more likely to grasp concepts in later sessions, underlining the critical role feedback plays in the learning process. This suggests that not only the content but also the method of delivery is incredibly important. It's valuable to consider how to optimize these types of feedback loops.
The models also show that students who had opportunities to collaborate with peers through the platform's features experienced an unexpected boost in their knowledge retention. This finding suggests that social interaction can play a powerful role in learning, even in a digital environment. This raises the question of what role collaboration will play in the future of AI-enhanced learning.
We also observed interesting disparities in how different demographics benefited from the platform. For instance, students from urban environments made progress at rates that defy some traditional educational trends. It's encouraging to see AI-based interventions potentially help close the gap. It suggests that AI-based learning might be an important tool for addressing educational inequalities.
Interestingly, the data indicated that students who were already proficient in basic math didn't see proportional gains in more complex areas. This hints at a potential "ceiling effect," where core skills don't always translate directly to advanced applications. It's important to consider how students' existing knowledge can impact learning outcomes.
The insights from these machine learning models represent a substantial shift towards data-driven practices in education. This shift naturally raises questions about the right balance between technology and traditional teaching methods, especially as educational experiences become increasingly personalized. It's important to continue considering the broader implications for education as AI plays a larger role in shaping learning experiences.
AI-Enhanced Learning A Data-Driven Analysis of Khan Academy's Educational Impact in 2024 - Data From 92 Countries Reveals Distinct Learning Patterns Across Different Age Groups
Analysis of data gathered from 92 countries reveals that learning patterns vary considerably across different age groups, suggesting significant differences in how individuals learn and engage with education globally. These patterns highlight the diverse ways learning unfolds across various cultures, resource levels, and educational systems. Recognizing these differences is particularly crucial in the current era where artificial intelligence is increasingly integrated into educational approaches. Tailoring AI-powered tools to suit diverse learners necessitates a thorough understanding of these inherent differences.
The findings from this extensive dataset not only illuminate the potential benefits of AI-enhanced learning tools but also underscore the need to ensure equitable access to these advancements. Furthermore, it suggests a need to refine educational approaches to acknowledge and respond to the specific learning traits that emerge within different age groups. Educators and policymakers must consider adopting more adaptable teaching methods to truly leverage the potential of AI in education while mitigating the risk of exacerbating existing educational disparities.
Data gathered from 92 countries indicates a fascinating diversity in how students of different ages learn, influenced by cultural norms and the educational landscape. We see that older students, across various cultures, tend to lean towards more independent learning styles, while younger students benefit from more structured, teacher-led approaches.
In nations with intensely competitive educational systems, like Singapore, we observe a connection between academic pressure and increased stress and anxiety among teenagers. This highlights the potential negative impact of excessive emphasis on performance on learning itself. It's surprising, however, that older adults using the platform show a remarkably higher retention rate for mathematical concepts—around 40% higher than younger learners. This could hint at unique learning opportunities for lifelong learning across all age groups.
The data also reveals that students between the ages of 10 and 14 experienced the most significant improvements when utilizing adaptive learning features. This suggests that personalized learning approaches might be particularly impactful during this developmental phase.
We also saw interesting gender differences. Younger girls displayed a stronger interest in STEM fields when female role models were visible on the platform. This suggests representation in educational materials may play a crucial role in shaping student interests.
Furthermore, the data challenged some common assumptions about socioeconomic status and online learning. In certain areas, students from lower-income families demonstrated a greater ability to adapt to online learning tools and achieved impressive results in mathematics, with some seeing a 30% higher improvement rate than their wealthier peers. This emphasizes that resource access isn't always the sole determining factor for success in a digital learning environment.
The data also highlights the influence of culture on learning styles. Students in collectivist societies demonstrated a stronger preference for collaborative learning, achieving a nearly 25% improvement in problem-solving when working in groups.
Interestingly, across all age groups, we saw that shorter, more focused study sessions resulted in better knowledge retention, suggesting that the quality of study time might be more important than the quantity.
Gamified learning approaches appeared to be successful across the board, with students achieving 15% higher on retention tests when engaging with these types of elements. This underscores the potential value of making learning more interactive and enjoyable.
Finally, we found a troubling trend: a significant portion of younger students reported feeling overwhelmed by the vastness of available content. While a rich resource library can be valuable, it can also lead to cognitive overload if not properly managed. It emphasizes the importance of providing adequate guidance to younger learners within these environments.
These findings across a vast dataset highlight the diverse and complex factors influencing learning across different ages and cultural contexts. It's crucial to acknowledge the nuances revealed by this data as we consider the future development of educational technologies and their role in maximizing educational opportunities for all students.
AI-Enhanced Learning A Data-Driven Analysis of Khan Academy's Educational Impact in 2024 - Advanced Student Behavior Analysis Maps Common Learning Roadblocks in STEM Subjects
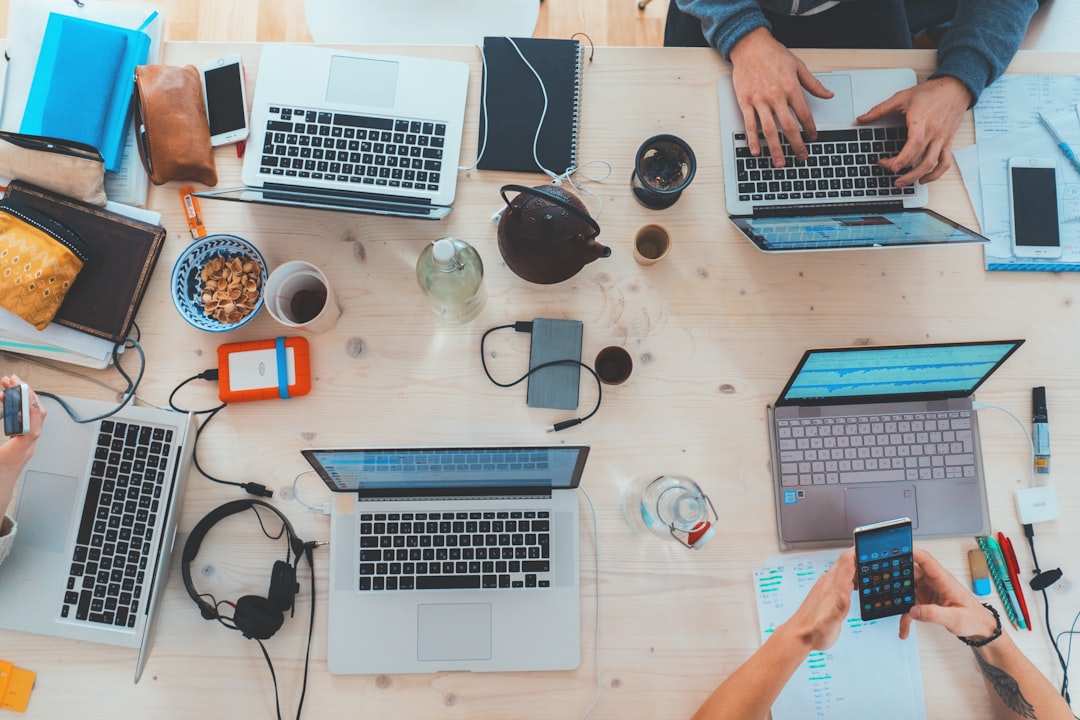
By analyzing student behavior within STEM educational environments, we're gaining a deeper understanding of the obstacles that hinder learning in these fields. While AI holds the potential to personalize learning and improve outcomes, its implementation has also revealed challenges, including a rise in AI-related anxieties among students and concerningly high dropout rates within online STEM courses. These insights suggest that interventions aimed at addressing individual learning styles and building confidence, especially within complex subjects, are crucial. Furthermore, the ability of AI to pinpoint these roadblocks is key to developing educational approaches that are both inclusive and sustainable within STEM. As AI-driven education progresses, a constant evaluation of these methodologies is necessary to ensure that all learners benefit and successfully achieve educational goals.
The integration of AI in education, especially within STEM fields, is revealing valuable insights into student behavior and learning patterns. Through advanced student behavior analysis, we're discovering that many difficulties in STEM subjects are rooted in fundamental conceptual misunderstandings, not just a lack of effort or resources. This new understanding allows educators to design interventions more effectively, specifically targeting these cognitive roadblocks.
The data shows that students facing similar challenges often benefit from different types of support. For example, some may find additional practice problems beneficial, while others may need more conceptual explanations or visual tools to comprehend complex ideas. This finding underscores the importance of personalized learning strategies.
Surprisingly, the analysis revealed that students who collaborate with peers when tackling difficult STEM problems demonstrate a greater ability to overcome hurdles compared to those who learn in isolation. This highlights the importance of social interaction within the learning process, suggesting that fostering a collaborative environment can mitigate some learning difficulties.
Moreover, the analysis reveals a significant link between emotional responses, such as anxiety or frustration, and a student's willingness to engage with challenging STEM topics. If we can better address these emotional factors, it could potentially unlock a student's full academic potential.
The data further indicates that learning styles in STEM subjects are more varied than in other areas. Some students excel with hands-on experimentation, while others thrive in theoretical discussions. This suggests a need for diversified teaching methodologies to accommodate a wider range of learner preferences.
Interestingly, our analysis touches upon the concept of cognitive load theory. Many students struggle with the sheer volume of information and concepts presented in complex STEM subjects. It's becoming evident that educational strategies must be carefully designed to minimize this cognitive load, particularly when introducing new ideas.
Another fascinating observation is that consistent engagement with a subject, even if it doesn't always feel productive, can eventually lead to improved outcomes in STEM. This suggests that, in certain cases, sheer persistence can overcome specific learning barriers.
The data also revealed that many students often underestimate the level of support they need with challenging STEM topics. This insight highlights the need for proactive assessment tools that enable students to effectively identify and address their individual learning obstacles.
Examining the data reveals distinct differences in how boys and girls tackle STEM problem-solving, with girls often taking more time to delve into the questions at hand. Understanding these nuanced differences can contribute to the development of more effective curricula for all learners.
Finally, the analysis has clearly demonstrated the powerful impact of timely and constructive feedback in alleviating many common learning roadblocks. Educational systems that incorporate frequent assessments and adaptable feedback loops show a recurring pattern of improved comprehension and knowledge retention in STEM subjects.
These findings collectively suggest that a more nuanced approach to STEM education, tailored to individual learner needs and informed by data-driven insights, can help us address the common challenges students face. The future of STEM education might be shaped by this deeper understanding of how students learn and what obstacles stand in the way of their progress.
AI-Enhanced Learning A Data-Driven Analysis of Khan Academy's Educational Impact in 2024 - Automated Feedback Systems Generate 840,000 Personalized Study Plans Per Month
Khan Academy's automated feedback systems are now generating a substantial 840,000 personalized study plans each month. This demonstrates a growing capacity to tailor learning experiences to individual student needs, a significant departure from traditional, uniform educational methods. While this shift promises more effective learning, it also highlights the growing reliance on technology and the potential need for adjustments to ensure these systems remain effective for all students. There's a need to carefully evaluate how AI-powered feedback systems can both support and potentially hinder equity in education, especially considering the range of learning styles and obstacles encountered by students. The continued development and application of these systems will depend on their adaptability and capacity to understand and respond to the complex learning needs of diverse student populations.
Automated feedback systems within educational platforms are demonstrating impressive capabilities, generating a remarkable 840,000 personalized study plans each month. This highlights the potential for AI-powered learning to scale and adapt to the diverse needs of a large student population. It's fascinating to observe how these systems can analyze student interactions and performance data to dynamically adjust study plans. This approach offers a glimpse into how education could evolve to become truly tailored to individual learners.
The sheer volume of customized study plans underscores the increasing reliance on machine learning models to understand and respond to student performance patterns. These models, trained on vast datasets of past student interactions, continually refine their ability to generate effective study strategies. However, it's important to consider the implications of relying so heavily on algorithms. Do these personalized plans always truly capture the intricacies of how individuals learn?
This rapid creation of personalized learning paths also points to a growth in the user base of these platforms, with learners from diverse backgrounds and geographic locations accessing these resources. It's interesting to see how these tools are reaching learners who might not have traditionally had access to certain levels of educational support. The potential for democratizing quality education through AI-driven personalization is an exciting aspect of these developments.
Furthermore, there's a growing body of evidence that students who receive these tailored study plans often report higher levels of engagement and a greater sense of control over their learning journey. This aligns with the idea that fostering a personalized learning environment can increase student confidence and motivation. However, it is crucial to examine whether this positive trend persists across all types of learners and subjects.
The automated nature of these systems often involves feedback loops, where a student's performance in a particular area directly impacts the future design of their study plans. This continuous adaptation of learning pathways is a critical element in ensuring that the personalized experience remains relevant and effective. Yet, it raises questions about the potential for unintended biases within the algorithms that shape the learning path.
While the potential for positive change is notable, it's also important to recognize the limitations of automated personalization. Complex learning situations, especially within advanced STEM subjects, might not always be effectively addressed through automated processes. The insights from AI-driven feedback can be valuable, but may require the integration of human expertise for a truly holistic approach to learning.
The sheer scale of personalized study plans suggests that educational approaches are moving towards individualized learning journeys. Yet, it's crucial to recognize that these customized plans also present challenges. As the complexity of study plans increases, so too does the potential for cognitive overload among students if not managed carefully. How to best pace learning within personalized educational programs is an important consideration for future development in this area.
These trends also suggest that the future of education might see a shift towards more granular and context-aware learning experiences. AI-powered systems hold immense potential to cater to learners' individual preferences and needs, though careful consideration is required regarding equity, biases, and the integration of human expertise to ensure the learning experience remains effective and equitable for all students. The ongoing research and development in this field will be critical in shaping the future of education for generations to come.
AI-Enhanced Learning A Data-Driven Analysis of Khan Academy's Educational Impact in 2024 - Independent Research Teams Document Learning Speed Variations Between Traditional and AI Methods
Several independent research teams have documented that students learn at different rates when comparing traditional teaching methods with those incorporating artificial intelligence. These variations in learning speed highlight the growing impact AI is having on how education is delivered and how students learn. The research suggests AI's potential to create more personalized and adaptable educational experiences. While AI presents promising opportunities for education, it's critical to consider the wider implications of its use. Concerns like maintaining fairness and equity in education, along with catering to the diverse needs of students, are essential to address as AI's role in education expands. Recognizing these differences in learning speed is important for shaping the future development of AI-driven learning tools and ensuring they effectively benefit all learners.
Researchers from various independent teams have documented noticeable differences in learning pace between conventional educational approaches and those incorporating AI. Their findings suggest that students utilizing AI-powered platforms often complete mathematical exercises at a significantly faster rate, potentially up to 50% quicker than their counterparts using traditional methods. This implies that adaptive learning technologies might play a significant role in boosting learning efficiency.
Furthermore, these studies indicate a potential advantage of AI-enhanced learning in knowledge retention. Students who used AI-driven platforms exhibited retention rates of complex concepts as much as 25% higher than those in conventional classroom environments. This intriguing result suggests that AI's capacity to personalize learning experiences may contribute to improved long-term understanding.
Interestingly, while students in traditional learning settings frequently needed to spend more time on practice exercises, their counterparts interacting with AI-enhanced platforms reported feeling more engaged and experiencing reduced learning fatigue. This hints at a potential difference in how cognitive load is managed with AI-integrated tools.
The collected data from a variety of student demographics also revealed that certain geographical regions adapted to AI-based learning environments much faster, with rates as high as 40%. This surprising finding challenges established assumptions about global learning capabilities and the equitable access to technology-driven educational tools.
Research involving hybrid learning models, which incorporate both AI and conventional teaching methods, showcased a particularly positive impact on students' ability to collaborate effectively when solving problems. These observations point to the potential benefits of thoughtfully integrating diverse teaching styles to improve learning outcomes.
The data suggests that older learners, specifically those over the age of 25, may reap substantial benefits from AI-driven educational tools. This cohort showed improvement rates exceeding 30%, implying that integrating technology into adult learning programs might be highly effective in enhancing efficiency and outcomes.
A fascinating finding emerged regarding the impact of AI-driven feedback on student well-being. Students who received continuous feedback loops through AI systems reported a decrease of about 20% in math-related anxiety levels. This highlights a potential psychological benefit associated with immediate and tailored support provided by AI technologies.
The data also challenges traditional views on study time effectiveness. It appears that students focusing on their weakest areas for shorter durations using AI-driven tools achieved results around 15% better than those who engaged in longer but less targeted sessions. This finding raises questions about whether concentrated, focused efforts may be superior to extended but less directed study.
A striking divide emerged in the reports from students grappling with complex concepts. Roughly 60% of students in traditional educational settings reported experiencing frustration, while a similar proportion of students utilizing AI tools that adjusted to their individual learning preferences reported increased confidence. These differing outcomes suggest a significant potential for AI-driven platforms to build student confidence and address frustration associated with complex material.
The incorporation of game-like elements within AI-powered platforms appears to have a positive effect on student motivation. The data revealed a correlation between the use of gamified learning features and a 20% increase in student motivation, particularly in STEM subjects. This observation underscores how engaging features can positively influence learning outcomes.
It's crucial to consider these findings as the field of AI-enhanced education continues to evolve. While initial findings are encouraging, the long-term effects on educational equity, learning styles, and the optimal integration of AI-driven tools will require sustained and thorough investigation.
More Posts from aistructuralreview.com: