AI-Enhanced Workshop Demonstrates 7 New Assessment Techniques for Mathematics Teachers in 2024
AI-Enhanced Workshop Demonstrates 7 New Assessment Techniques for Mathematics Teachers in 2024 - Interactive Grade Analysis Through QUICKComments Software For Math Teachers
Within the evolving landscape of math education, the QUICKComments software presents an intriguing approach to streamlining the assessment process for instructors. This freely accessible tool employs AI to suggest brief, insightful feedback on student work, potentially reducing the time teachers spend on providing responses. Its integration with ASSISTments, a platform offering immediate feedback to students, further strengthens the analytical capabilities, supplying teachers with a richer understanding of student performance. This integration allows for a more targeted approach to instruction, as teachers can adapt their teaching to individual student needs based on the data provided. Despite the potential benefits, the implementation of such AI-driven tools remains a work in progress. Educators must thoughtfully consider how to best integrate these new technologies into their classrooms, ensuring they enhance – rather than detract from – the learning experience. QUICKComments, despite its limitations, highlights a movement toward more refined assessment methods in math, a realm where efficient and informative feedback is crucial for fostering student understanding and growth.
QUICKComments is a tool being explored to streamline math teachers' grading process. It offers the capability to automatically generate specific feedback on student work, which has the potential to cut down grading time substantially, freeing up more time for teachers to focus on student comprehension. The system also attempts to identify prevalent errors across student assignments. This aggregated data can be used to uncover common areas of struggle within the class, providing valuable insights for instructional adjustments.
Furthermore, QuickComments allows teachers to modify feedback templates based on particular lessons, allowing them to tailor feedback to specific mathematical concepts and skills. The idea is to foster student self-reflection by having them review the feedback themselves. This self-reflection component promotes a more engaged approach to learning. QuickComments features analytics that can track individual student performance over time, which can help identify students needing additional support or those who might benefit from more challenging material.
Another interesting aspect of the software is its multilingual capability. It's designed to translate feedback into several languages, making it more accessible for students who are not native English speakers. Some teachers utilizing QuickComments have noted a possible improvement in student motivation due to the personalized nature of the feedback. It also learns over time. Its algorithm, based on past feedback given, proposes new feedback based on recurrent patterns of student error, which could have an impact on both instructional strategies and student understanding.
One of the potential benefits is that the platform can be linked to other commonly used educational platforms, facilitating a more integrated approach to student assessment. Moreover, data derived from QuickComments, if used effectively, can potentially contribute to broader educational research. It could assist in uncovering systematic challenges within math education across schools, potentially influencing curriculum design and instructional methods. The question still remains whether such systems will fundamentally change how educators approach feedback or if they remain largely supplementary tools.
AI-Enhanced Workshop Demonstrates 7 New Assessment Techniques for Mathematics Teachers in 2024 - Machine Learning Algorithms Track Student Progress In Algebra Word Problems
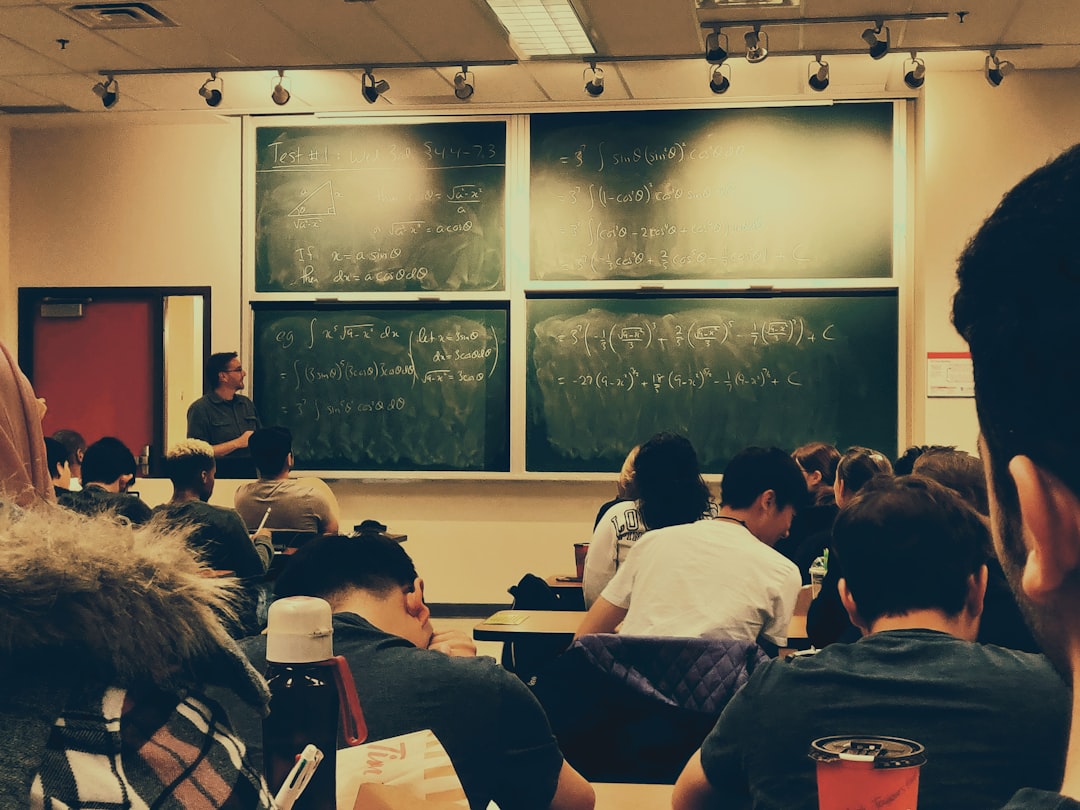
Within the evolving landscape of math education, machine learning algorithms are being explored as a means to monitor student progress in algebra, especially when tackling word problems. These algorithms employ intelligent assessment methods to track student understanding and performance, providing educators with a more detailed picture of individual student needs. By processing the language used in student responses and adapting the learning path, these algorithms can generate personalized learning experiences with real-time feedback and customized instruction. However, this development raises questions about the appropriate implementation of such AI tools in education. Teachers need to carefully consider how to integrate these tools effectively, making sure that they truly enhance – not detract from – the learning experience. There's a delicate balance to be found in leveraging technology without disrupting the core principles of effective teaching and learning. The future will likely see more refinements in how these AI tools can help educators better assess student needs and provide individualized instruction, but concerns over potential over-reliance on automated systems must also be addressed.
Machine learning algorithms are increasingly being used to analyze student work, especially in areas like algebra word problems. By examining patterns in student responses, these algorithms can potentially identify areas where students are struggling, even before teachers might notice. This capability allows educators to intervene earlier and provide more targeted support, hopefully leading to better outcomes.
Interestingly, machine learning can also analyze the language used in student responses, potentially detecting emotional cues that hint at a student's confidence and engagement levels. This is a novel approach to assessment that could yield insights into the psychological aspects of learning mathematics.
Moreover, these algorithms can assess the complexity of a student's written responses, going beyond just checking for numerical accuracy. They use techniques like natural language processing to evaluate a student's understanding of the word problem itself. The goal is to understand how well students comprehend the problem before attempting to solve it.
These systems have the ability to dynamically adjust the difficulty of problems based on a student's performance. This tailored approach to questioning aims to ensure that each student is constantly challenged at an appropriate level while also focusing on their specific areas of weakness. This adaptive feature offers the potential for personalized learning that continuously monitors and adjusts to the learner's needs.
Machine learning also provides tools to understand the effects of different teaching methods. By analyzing the data generated by students using a particular method, educators can get a better sense of its effectiveness. This data-driven approach can help teachers refine their teaching methods based on concrete evidence of what works best for their students.
When trained on a diverse set of data, these machine learning models can offer a unique lens into how different student demographics approach problem-solving in algebra. Such insights could help illuminate potential biases in existing educational materials or teaching practices. Some systems even allow teachers to compare a student's performance against their classmates, fostering both competition and collaboration.
The capabilities of these machine learning-based systems are evolving, with the potential to provide instant, formative feedback during lessons. This could fundamentally shift the assessment landscape from a focus on summative testing to continuous, dynamic feedback that fuels a student's engagement and adaptation to the learning material. However, it's important to remember that while the insights provided by machine learning are powerful, they must be validated carefully against actual classroom practices. This ensures that algorithmic recommendations truly align with sound educational principles and support genuine student learning. We need to ensure that these systems are enhancing – not hindering – the educational experience.
AI-Enhanced Workshop Demonstrates 7 New Assessment Techniques for Mathematics Teachers in 2024 - AI Generated Math Problems Adapt To Student Performance And Interest Level
AI is now being used to create math problems that change based on how well a student is doing and what they find interesting. This is a step forward in giving students a personalized education. These AI systems can figure out a student's strengths and weaknesses, and then adjust the difficulty and types of math problems given to them, which can keep them more interested in learning. This customized approach to learning helps students understand the material better, but also keeps them motivated by making sure the work is both relevant and challenging enough. However, there are questions about how well these AI-driven systems will work over time, and how they should fit in with how math is normally taught. Teachers must be careful to make sure that these new technologies actually help improve learning, and that they don't take away from core teaching methods.
In the realm of math education, AI is emerging as a powerful tool for tailoring learning experiences. AI-generated math problems are capable of adapting in real-time to a student's performance, ensuring they're challenged at an appropriate level. This dynamic adaptation takes into account a student's accuracy, speed, and engagement with the material. Furthermore, these systems can be designed to incorporate a student's individual interests, for instance by incorporating real-world scenarios or topics that resonate with them. This personalization can significantly enhance engagement and perceived relevance of math problems.
Beyond immediate adaptation, AI can also employ predictive analytics to anticipate a student's future performance on various concepts by examining their past work. This allows teachers to preemptively address potential struggles. Moreover, the sophisticated algorithms powering these systems are capable of recognizing common mistakes across students. This could highlight systematic issues in teaching methods or curriculum gaps that might otherwise go unnoticed.
Interestingly, these systems can even begin to delve into the emotional landscape of learning math by analyzing language and interaction patterns in a student's written answers. This offers a potential new dimension to assessment, potentially informing interventions designed to address issues like anxiety or a lack of confidence in mathematics.
Moving beyond simply checking if answers are right or wrong, AI can also evaluate the complexity and nuance of how a student expresses their understanding. This provides a deeper insight into their grasp of the underlying mathematical concepts involved. Additionally, AI-driven platforms can promote a competitive spirit by allowing students to compare their performance with their classmates. This could encourage a healthy drive for improvement while possibly fostering collaboration.
Importantly, AI-powered math problem generation is inherently designed to continuously refine itself based on its interactions with students. Over time, the quality and relevance of generated problems should improve. This means that the learning experience becomes increasingly tailored and customized to each student's unique learning journey.
Analyzing large volumes of student data offers another opportunity for educators and researchers. It can expose trends and indicators across various student demographics, potentially identifying biases or gaps in educational resources. This potential for understanding systematic problems offers a chance to work towards more equitable outcomes.
Finally, the increasing integration of AI-generated math problems marks a transition towards a greater emphasis on formative assessment. This implies a shift away from a primary reliance on infrequent, summative exams. Instead, AI allows for ongoing, immediate feedback and adaptive adjustments to learning strategies, promoting a more responsive and continuous approach to education. While this area of research is still evolving, the prospect of creating individualized math learning experiences holds significant potential for optimizing the way we teach and learn mathematics. However, careful consideration must be given to ensure the AI tools do not unintentionally undermine effective educational principles or create new forms of bias.
AI-Enhanced Workshop Demonstrates 7 New Assessment Techniques for Mathematics Teachers in 2024 - Voice Recognition Tools For Mathematical Equation Dictation And Grading
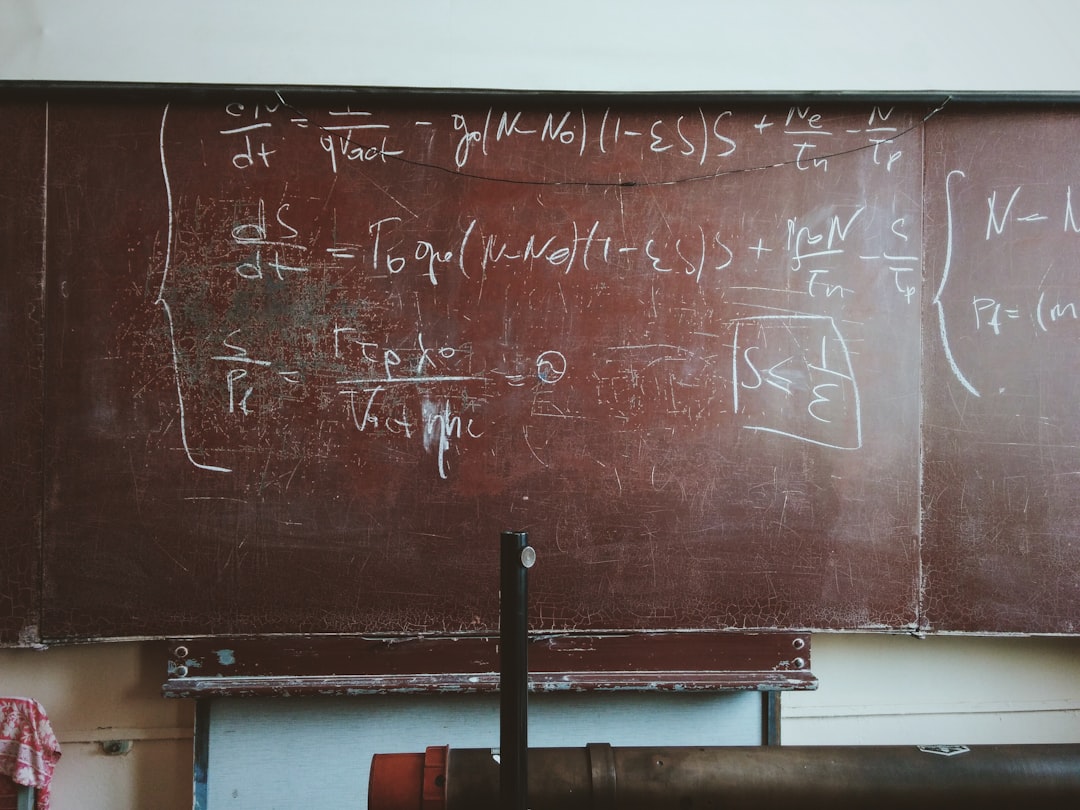
The integration of voice recognition tools is reshaping mathematics education, especially for the dictation and assessment of mathematical equations. Tools are now available that facilitate the process of converting spoken mathematical expressions into written format, like Mathfly and Talon. This can benefit students by providing a more accessible way to engage with mathematics, while freeing up educators to concentrate on teaching rather than transcribing equations. Furthermore, recent developments in AI are enabling innovative methods for delivering mathematical solutions and feedback, exemplified by tools such as MathGPT and DicMath. However, challenges persist within current voice recognition capabilities. These tools could be more accurate and user-friendly with the incorporation of a dedicated "math mode" that specifically addresses the unique nuances of mathematical notation. As these tools continue to improve, they are expected to not only enhance grading efficiency but also contribute to a more dynamic and inclusive learning environment. The potential for voice recognition to personalize feedback and cater to varied student needs is a promising aspect of this emerging field. It's crucial to monitor the development of these tools to ensure they are truly serving to enhance, rather than potentially hindering, a deep and robust understanding of mathematics.
Voice recognition tools are being developed to help with mathematical equation dictation and potentially grading. These tools aim to bridge the gap between spoken language and mathematical notation, converting spoken equations into formats like LaTeX for seamless use within educational platforms. One of the exciting advancements is the improved ability of these systems to understand the context of mathematical language. This means distinguishing between similar-sounding words, like "plus" and "minus", which greatly increases the accuracy of transcription. Some tools even personalize their recognition over time, learning individual pronunciation styles and making dictation even more precise.
These tools are becoming increasingly sophisticated in their ability to process mathematical symbols. They can now handle intricate equations, including those with fractions and radicals—aspects that were previously challenging for voice-based input. Beyond just dictating equations, some researchers envision voice recognition playing a role in real-time grading. Students could potentially speak their answers to open-ended math problems, and the system could evaluate the responses based on mathematical logic and concepts. This capability, if refined, could shift assessment towards a more dynamic approach.
The potential integration with other educational tools is noteworthy. Voice recognition could act as a grading assistant, gathering data from spoken answers for further analysis and improving feedback loops. This capability is in early stages, but hints at a more integrated assessment approach. Initial studies suggest that utilizing voice for mathematical input could increase student engagement, especially for students who find writing challenging. Their spoken contributions can be readily captured and evaluated, encouraging active participation.
However, these tools are not without their challenges. Some systems are starting to employ machine learning to identify patterns in common verbal mistakes, which is valuable data for teachers to address misconceptions. Maintaining accuracy in these systems requires continuous refinement. As mathematical language and teaching methods evolve, the training data these systems rely on needs constant updating. This raises questions about long-term sustainability. And, while potentially valuable, equitable access to this technology for all students needs careful consideration. Not everyone is equally comfortable using voice recognition, and incorporating these tools into the classroom must be done thoughtfully to avoid creating new barriers for students. The future of these tools in math education is still unfolding, but the potential to enhance assessment and create a more engaging experience for learners is promising.
AI-Enhanced Workshop Demonstrates 7 New Assessment Techniques for Mathematics Teachers in 2024 - Real Time Performance Analysis Dashboard For Group Mathematics Projects
A "Real-Time Performance Analysis Dashboard for Group Mathematics Projects" is a novel approach to monitoring student progress within collaborative learning environments. This type of dashboard, often built using tools like Power BI, provides teachers with dynamic visualizations of student performance data in real-time, during the course of a group project. This allows educators to quickly gain insights into the overall performance of a group, as well as the contributions of individual students. By readily displaying current performance data, the dashboard supports the concept of adaptive learning, giving teachers the ability to promptly identify areas where students might be struggling and adjust instruction as needed. This approach represents a move toward data-driven decision-making in mathematics education, but as with any technological innovation within education, it needs careful integration to make sure it enhances, rather than detracts from, the effectiveness of traditional teaching methods. It's a promising tool, but caution must be exercised during implementation to prevent the creation of an overly data-centric classroom that might neglect the human aspects of learning and teaching.
In the realm of collaborative math projects, a real-time performance analysis dashboard offers a dynamic way to visualize student progress and group interactions. This type of dashboard, potentially powered by tools like Power BI, could leverage visualizations to display a constant stream of data points related to student performance. Instead of relying on static reports, teachers can see the ebb and flow of a student's (or group's) progress within a project, which could allow for immediate adjustments to teaching methods.
For example, it might show which students or groups are excelling and which are struggling in specific areas of a project. This could highlight potential weaknesses or misunderstandings before they become major issues, allowing teachers to provide timely interventions. Furthermore, by analyzing the interaction within groups, the dashboard might identify individuals who aren't contributing adequately or who might be dominating the group.
While the concept is promising, there are questions about the accuracy and reliability of the data it uses to create these visualizations. There's a risk of over-reliance on such a system if it isn't rigorously tested and validated. Another point of concern is whether the creation of such dashboards will inevitably lead to comparisons between groups, potentially creating a more competitive environment rather than a collaborative one.
Despite these potential challenges, this approach presents a compelling opportunity for educators to refine group mathematics projects and foster more engaged learning. The concept of a continuous feedback loop, where teachers can adapt their methods based on real-time student progress, is a departure from traditional assessment models. The long-term success of this approach depends on how effectively these dashboards can incorporate a nuanced understanding of collaborative learning dynamics and promote a constructive learning environment. There is still much to learn about how best to integrate this level of data visualization and how it might change teacher-student relationships and the very way we understand learning.
Furthermore, the ability to customize these dashboards could be crucial. Teachers should be able to tailor the specific metrics displayed to match their specific projects and assessment methods. This would ensure that the dashboard aligns with the specific learning objectives of the class.
Additionally, integrating this dashboard with other educational technologies would allow educators to construct a more comprehensive picture of student progress. For example, it might be integrated with platforms that track student engagement outside of class or with specific learning tools used within the project. The real potential lies in creating a connected ecosystem of learning data that paints a fuller picture than any single data point could ever capture.
However, even as we envision these possibilities, we must remain cautious. The collection of such sensitive data necessitates robust safeguards to protect student privacy and ensure responsible use of information. The equitable access of such tools to all students is also an important ethical consideration, since technological disparities could inadvertently reinforce educational inequalities. The goal should always be to use AI to enhance the learning process, and not create new barriers to it.
AI-Enhanced Workshop Demonstrates 7 New Assessment Techniques for Mathematics Teachers in 2024 - Automated Pattern Recognition In Student Problem Solving Approaches
In mathematics education, automated pattern recognition is gaining traction as a means to understand how students approach problem-solving. These systems analyze student work, identifying recurring patterns in strategies and mistakes. This allows educators to pinpoint areas where students commonly struggle and design interventions that cater to individual learning needs. The use of AI within this context makes it possible to provide timely, formative feedback through adaptive learning systems that offer support at key points in the problem-solving process. This targeted approach could potentially strengthen both comprehension and retention of mathematical concepts. However, there's a need for caution when utilizing these AI-driven tools. It's essential to integrate them carefully, ensuring they augment – not replace – the core elements of effective teaching that depend on human interaction, understanding, and empathy. The field of AI in education is constantly changing, and educators need to constantly scrutinize how these automated systems contribute to meaningful learning outcomes. They must also be alert to potential biases within the data that these systems rely on, and carefully consider how to mitigate them.
In the realm of mathematics education, automated pattern recognition is increasingly being used to gain a deeper understanding of how students approach problem-solving. This technology can analyze a vast amount of student work, potentially revealing subtle patterns and approaches that might be missed by human observers. By automatically categorizing common mistakes, teachers can quickly identify prevalent misunderstandings and adjust their instruction accordingly.
This automated analysis can also go beyond simply identifying errors. It can help teachers recognize the variety of methods students employ when tackling mathematical problems, providing insight into the diverse ways students think. This can be particularly useful in understanding how students with different learning styles approach challenges. Furthermore, the ability to provide immediate feedback based on patterns in student responses presents a compelling opportunity for teachers to intervene in real-time, adjusting their instruction to better address the needs of individual learners.
These systems can also track a student's problem-solving skills over time. By monitoring the evolution of their approaches, teachers gain a longitudinal view of student development, making it easier to spot areas where a student might be struggling or where they are excelling. The integration of natural language processing allows the system to examine not only the numerical answers but also the clarity and coherence of students' explanations, providing a richer understanding of their grasp of the underlying mathematical concepts. Some systems are even beginning to analyze language patterns to understand student confidence levels, providing an interesting new dimension to assessments.
The ability to scale automated assessment tools across various classrooms and institutions allows for the collection of standardized data. This aggregated data can then be used to inform educational research, potentially identifying systematic issues or biases in current math education practices. This potential to examine student work across demographics can also contribute to fairer and more equitable educational practices and materials.
However, these tools also bring the challenge of fostering accountability within collaborative projects. While analyzing group dynamics can provide valuable insights into the individual contributions of group members, it's important to strike a balance between recognizing individual efforts and promoting a sense of collective responsibility for achieving project goals.
It's vital to approach the implementation of such tools with a critical lens. While offering considerable promise for improving educational practices, the potential for over-reliance or misuse of the data needs to be carefully considered and addressed. It's a promising area of research, but ongoing validation of these systems against existing teaching practices is essential to ensure that these technologies are truly supporting – and not hindering – effective teaching and learning in mathematics.
AI-Enhanced Workshop Demonstrates 7 New Assessment Techniques for Mathematics Teachers in 2024 - 3D Visualization Tools For Geometric Problem Assessment
The integration of 3D visualization tools into geometric problem assessment is ushering in a new era in mathematics education. Software like GeoGebra 3D and CalcPlot3D offers students dynamic, interactive ways to explore the intricacies of three-dimensional shapes and relationships. These tools go beyond the limitations of static diagrams and traditional teaching methods by providing immersive experiences, sometimes incorporating augmented or virtual reality to bridge the gap between abstract concepts and real-world application. The interactive nature of these tools allows students to actively manipulate and experiment with geometric figures, potentially improving their grasp of complex spatial relationships and bolstering both their retention and engagement with the subject matter. However, teachers need to be mindful when introducing these technologies. Simply adding them to the curriculum without careful planning and consideration might not maximize their impact. It's essential that their use effectively complements established teaching methods rather than becoming the sole focus, thereby creating a holistic and robust educational experience.
The integration of 3D visualization tools is emerging as a promising avenue for improving the assessment of geometric understanding. These tools can present complex shapes with greater accuracy than traditional 2D diagrams, allowing teachers to highlight intricate relationships between dimensions that students might otherwise miss. This precision can greatly aid in developing a stronger foundation for spatial reasoning.
One of the major advantages is the interactive nature of these tools. Unlike static images, students can directly manipulate 3D models by rotating, zooming, and even dissecting them. This interactive environment can foster a more engaged and curious approach to learning geometric properties. Furthermore, it opens doors to augmented learning experiences like virtual reality simulations that immerse students in geometric landscapes. Students can directly interact with 3D models within these environments, leading to a deeper understanding of complex concepts.
3D tools are also proving beneficial in understanding the patterns of common mistakes. By observing how students interpret shapes and spatial relationships, teachers can readily identify frequent errors. This awareness allows them to refine their teaching methods to specifically address areas where students tend to struggle, leading to more focused and effective instruction.
It's worth noting that 3D visualization has applications across numerous fields, including engineering, architecture, and physics. This cross-disciplinary nature can help students grasp the importance and real-world relevance of geometry beyond the confines of a mathematics classroom.
In terms of assessment, 3D visualization offers an alternative to traditional written responses. Students could showcase their knowledge by manipulating models to solve problems, actively demonstrating their understanding. This active approach to assessment may enhance learning by requiring students to apply their knowledge in a more dynamic manner.
Moreover, these tools seem to play a role in fostering better cognitive abilities related to spatial awareness. Using 3D visualization tools has been associated with improvements in critical thinking, problem-solving, and creativity, all vital skills for mathematical understanding.
For students with visual impairments, the pairing of 3D tactile models and digital visualizations offers a potentially powerful tool for learning. This multi-sensory approach could create innovative experiences where students can touch and manipulate objects while receiving enhanced visual feedback.
Interestingly, some 3D visualization tools include analytic capabilities that enable real-time tracking of student interactions. This feature allows teachers to monitor both individual and group performance during lessons, creating opportunities for implementing adaptive learning approaches.
The availability of these tools also allows for a new level of collaboration across geographical boundaries. Students can participate in collaborative geometric projects with peers from around the world, exposing them to different learning approaches and a broader understanding of mathematical concepts.
Although the promise of these technologies is substantial, we must be mindful of the challenges. There are questions about the long-term efficacy and appropriate implementation of 3D visualizations in various learning contexts. It is crucial that they supplement, not replace, the valuable aspects of human interaction and tailored feedback in math education. Careful consideration is needed to ensure these tools are seamlessly integrated into classroom environments in a manner that enhances, rather than potentially detracts from, effective teaching practices.
More Posts from aistructuralreview.com: