Revolutionize structural engineering with AI-powered analysis and design. Transform blueprints into intelligent solutions in minutes. (Get started for free)
New Advancements in CFD Software for Structural Analysis A 2024 Overview
New Advancements in CFD Software for Structural Analysis A 2024 Overview - Enhanced Combustion Modeling in Ansys Fluent 2024 R2
Ansys Fluent 2024 R2 has introduced some noteworthy updates to its combustion modeling capabilities. A new Char Burnout Kinetics (CBK) model has been added, which is expected to provide more accurate predictions in combustion simulations. Furthermore, the eddy dissipation model has undergone optimization, which should lead to better handling of a broader range of turbulent reacting flows. This includes an enhanced ability to predict both premixed and diffusion flames, potentially making it more useful in fields like aerospace and defense where these types of combustion phenomena are critical. Beyond these specific model enhancements, Fluent 2024 R2 has also incorporated improvements to numerical stability and solution algorithms. This should result in quicker solution convergence and a more resilient simulation process, addressing challenges common in complex combustion simulations. While these updates show potential, their effectiveness and real-world impact will need to be carefully evaluated in various practical applications.
The latest release of Ansys Fluent, version 2024 R2, has made strides in enhancing combustion modeling, particularly within its multi-GPU solver environment. One notable aspect is the expanded hardware compatibility, now including AMD GPUs, which could potentially offer improved performance for specific workflows. The development team has also worked on improving the meshing process in the pre-processing phase, potentially leading to more accurate simulations, though it remains to be seen how broadly this applies across different types of simulations.
Furthermore, updates to the combustion modeling toolkit itself are intriguing. The new Char Burnout Kinetics (CBK) model is particularly noteworthy, potentially offering better predictions for scenarios involving char combustion. The eddy dissipation model has undergone optimization, hopefully increasing its robustness for various turbulent combustion scenarios, including premixed and diffusion flames. Improvements in the numerical solvers, including enhanced stabilization and solution schemes, could result in faster convergence and more reliable solutions, though their real-world impact needs to be tested.
In addition to combustion-specific enhancements, there are some updates that might have indirect benefits for combustion studies. For example, improved in-flight icing prediction models might prove helpful in situations where combustion-related ice accumulation is a concern. The software maintains its focus on multiphysics connections, which is valuable given the complex interactions frequently observed in combustion systems. The backward compatibility of the software is also welcome, facilitating smooth transitions for existing user bases. However, it's crucial to note that the practical benefits of these advancements are context-dependent, and rigorous testing is needed to determine their full impact across different applications and operating conditions.
New Advancements in CFD Software for Structural Analysis A 2024 Overview - Multiphysics Simulation Advancements in Ansys 2024 R2
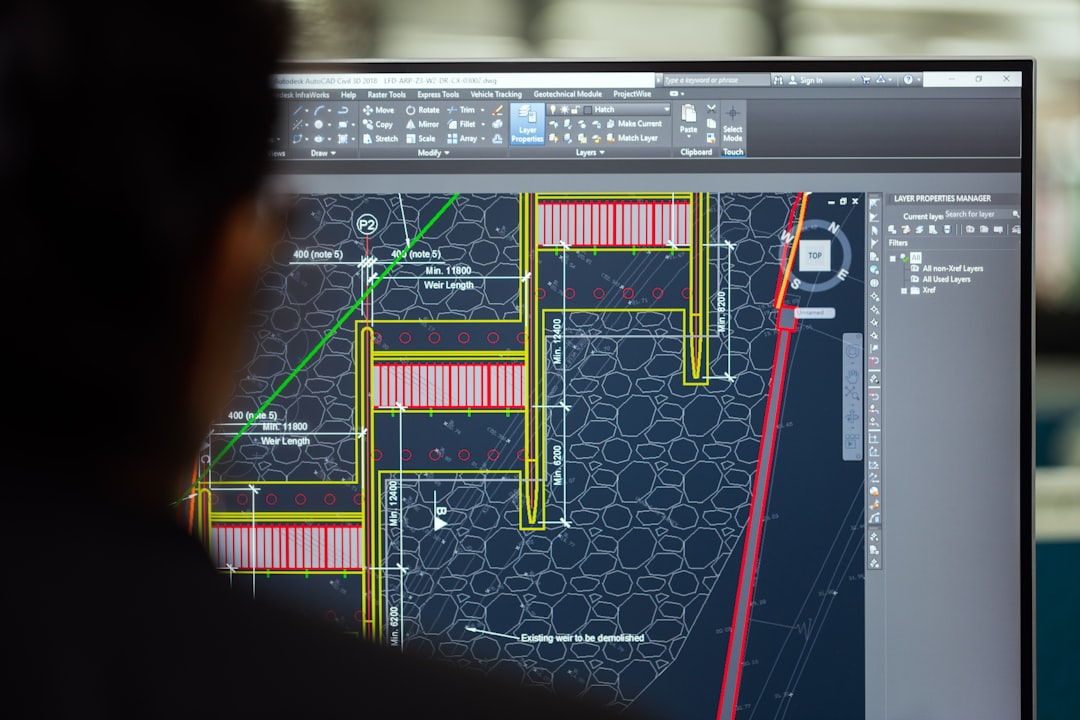
Ansys 2024 R2 introduces notable enhancements to its multiphysics simulation capabilities, aiming to provide a more integrated and comprehensive approach to product development. One key development is the improved ability to seamlessly connect different software technologies across various engineering domains. This streamlined workflow can lead to a more holistic understanding of complex systems where multiple physical phenomena interact. The software's focus has shifted towards multi-dimensional insights, advocating for a move beyond traditional, single-physics analyses to a more comprehensive view of product performance throughout its entire lifecycle. This includes aspects such as cost optimization and performance evaluation, relevant to a diverse range of applications, from microchips to electric vehicle powertrains.
The new release also features updates in specialized areas, such as sensor technology for enhanced radar digital twins and improvements within the operational design domain for autonomous vehicles. These innovations illustrate how the software adapts to emerging engineering challenges, particularly in safety-critical applications. The overall goal is to enable a deeper, more thorough evaluation of product performance, which in turn can lead to more innovative and reliable designs. However, the real-world practicality of these advances remains to be seen, as the effectiveness of these improvements needs to be thoroughly assessed across a variety of complex use cases. Ultimately, the true impact of these updates will depend on how they translate to tangible benefits in actual design and engineering processes.
The latest release, Ansys 2024 R2, has brought about a number of interesting developments in its multiphysics simulation capabilities, pushing beyond just traditional single-physics approaches. One notable feature is the inclusion of more sophisticated nonlinear material models, which can better represent the behavior of materials under complex loading conditions. This could potentially lead to more realistic predictions for structures operating in challenging environments.
Another area of improvement is in the coupling between electromagnetic fields and fluid dynamics, making it more convenient to simulate systems like electric motors or transformers where interactions between thermal, fluid, and magnetic effects are crucial. It will be interesting to see how this affects the accuracy of these types of simulations.
The integration of cloud computing resources for real-time simulations is also noteworthy. While the concept is not entirely new, the move towards enabling faster design cycles through readily available computing power is an important development. It would be interesting to compare the performance of these cloud-based simulations with conventional methods.
Furthermore, the new release allows users to develop their own custom physics interfaces, offering more flexibility in modeling unique engineering challenges. However, the ease of use and accessibility of this feature remain to be seen.
Another enhancement is the improvement to dynamic mesh handling. This advancement could facilitate more accurate modeling of scenarios with moving boundaries, like fluid-structure interactions, particularly in rotating machinery. The effectiveness of these enhancements in various simulation scenarios will be critical to evaluating their overall utility.
There's also an increased emphasis on the role of machine learning in simulation. Ansys 2024 R2 can leverage historical data to predict simulation outcomes and potentially optimize designs, hinting at a future with more automated design processes. It will be important to assess the robustness and accuracy of these machine-learning driven predictions, particularly when dealing with complex scenarios.
The post-processing capabilities have also seen a boost, with enhanced visualization tools designed to aid engineers in understanding and interpreting simulation results. However, the impact of these improvements in practical scenarios is still to be evaluated.
Another interesting aspect is the ability to directly incorporate Particle Image Velocimetry (PIV) data into simulations. This feature should facilitate more seamless validation processes by allowing a more straightforward comparison between experimental and simulated data.
The turbulence models have also been refined, potentially extending the range of flow structures that can be effectively analyzed. The true value of these improvements will likely depend on the complexity of the flow phenomena being modeled, and it will be interesting to see how well they handle flows with strong separation or complex turbulence structures.
Finally, the software now includes capabilities for supply chain-related simulations. This aspect offers the potential to holistically evaluate project feasibility by incorporating factors like materials and logistics into the design process. However, the true value of these simulations in the decision-making process of real-world engineering problems requires further analysis.
Overall, Ansys 2024 R2 presents a collection of enhancements to multiphysics simulation capabilities. While many of these developments appear promising, their effectiveness and impact in various applications need to be carefully studied and validated. The future of engineering design may very well depend on the extent to which these advancements enable more accurate, efficient, and innovative solutions.
New Advancements in CFD Software for Structural Analysis A 2024 Overview - Machine Learning Integration Boosts CFD Software Capabilities
The incorporation of machine learning (ML) techniques into computational fluid dynamics (CFD) software is significantly improving the ability to perform simulations. These advancements are being implemented through two primary approaches: using ML within the simulation loop itself (ML-in-the-loop) and using ML to analyze and optimize simulations outside the main CFD loop (ML-outside-the-loop). ML is proving particularly valuable for speeding up simulations involving turbulent flows, weather forecasting, and aerodynamic studies. By leveraging various ML methods, CFD can better handle complex fluid dynamics and reduce the computational overhead associated with complex simulations. This trend suggests that ML will be a driving force behind future advancements in scientific computing, particularly within the realm of CFD, potentially unlocking new applications across various engineering disciplines. Nonetheless, the extent to which these integrated ML approaches truly enhance the effectiveness and reliability of CFD in real-world applications still requires careful investigation and testing.
The integration of machine learning (ML) into Computational Fluid Dynamics (CFD) software is rapidly reshaping the field, offering a new set of tools to tackle complex fluid flow problems. This integration is primarily focused on enhancing simulation accuracy and significantly reducing computational demands. We're seeing two primary approaches: ML-in-the-loop and ML-outside-the-loop. In the latter, ML models are trained on data generated by traditional CFD methods with minimal interaction during the actual simulation.
ML can significantly accelerate various CFD tasks, such as turbulent flow simulations, weather and climate modeling, and aerodynamic analyses, all of which were previously incredibly computationally intensive. The ability to leverage ML to quickly analyze a large number of potential designs provides an important advantage in the design process. A range of machine learning techniques are being applied to improve upon existing CFD approaches like finite differences, finite volumes, and finite elements.
A recent surge in published research has looked back at the previous five years of advancements in CFD and examined the ways ML is contributing to better outcomes. Overall, this development reflects ML's growing importance in scientific computing, opening up opportunities to broaden the scope and utility of CFD. For example, the design of scenarios for practical applications in areas such as aerospace engineering can be improved by utilizing ML algorithms integrated into CFD software.
Some of the challenges currently being faced in CFD research, such as capturing highly complex turbulent flows, might be addressed by further development of ML techniques. The field is evolving and we anticipate that ongoing advancements in ML methods will drive new innovation and capabilities within CFD. It will be fascinating to see the innovations that emerge in this field over the coming years. It's worth noting that there are still considerable hurdles to overcome, particularly in the realm of model validation and the integration of ML approaches within existing CFD workflows.
New Advancements in CFD Software for Structural Analysis A 2024 Overview - Expansion of CFD Applications Beyond Traditional Engineering Fields
Computational Fluid Dynamics (CFD) is no longer confined to traditional engineering domains like aerospace or mechanical engineering. Its applications are expanding into diverse fields, including energy research, environmental science, and even areas like financial modeling. This wider adoption reflects a growing understanding of CFD's potential to tackle complex problems across a range of disciplines.
The integration of machine learning within CFD is particularly noteworthy, enabling enhanced predictive capabilities and optimization strategies. These developments are opening doors for new avenues of research and application. As society faces challenges like climate change and energy scarcity, the ability of CFD to model and understand these complex systems is becoming increasingly crucial. The future of CFD appears to lie in its capacity to facilitate interdisciplinary collaborations and tackle problems beyond its traditional boundaries.
While the potential of CFD's expansion is exciting, the practical implications and limitations of these new approaches must be carefully scrutinized. The efficacy and reliability of these evolving techniques in diverse real-world scenarios still needs rigorous evaluation and validation. This critical assessment is crucial to ensure that these promising advancements truly deliver their intended impact.
CFD, a tool initially confined to traditional engineering domains, has expanded its reach into a diverse array of fields. Its application in understanding blood flow within the human body is a notable example, impacting the design of medical devices and offering insights into conditions like cardiovascular disease. Interestingly, the principles of CFD have also found their way into art and architecture, aiding in the design of aesthetically pleasing and functionally sound structures through simulating airflow and thermal comfort.
The food industry is increasingly reliant on CFD to optimize processes like mixing and drying in food production, helping ensure consistent and high-quality food products. Similarly, the realm of sports engineering leverages CFD to optimize athletic equipment design, reducing drag in cycling or enhancing performance in swimming.
At the microscopic level, CFD is fundamental to microfluidics, enabling the design of intricate microfluidic devices used in lab-on-a-chip technologies for diagnostics and drug development. In the context of energy harvesting, CFD aids in the design of systems like piezoelectric sensors, maximizing their efficiency in diverse environments.
Urban planning now incorporates CFD to model wind patterns and pollutant dispersion, which is crucial for designing cities that promote air quality and thermal comfort. Moreover, the potential of CFD to simulate wildfire behavior holds significant promise for improving emergency response and risk assessment in fire-prone areas.
The ever-growing complexity of consumer electronics necessitates efficient thermal management, with CFD playing a critical role in simulating heat dissipation to improve product longevity. While traditionally used in aerodynamics, CFD is now contributing to the optimization of aircraft paint application, ensuring both performance enhancement and reduced weight for improved fuel efficiency.
While the accuracy and efficiency of CFD techniques continue to benefit from advancements in turbulence modeling and algorithmic development, its digital integration across these various fields has paved the way for novel research and applications beyond the conventional engineering space. The potential of CFD to address problems in areas as diverse as healthcare and wildfire simulation indicates a growing trend towards broader adoption, but careful evaluation and validation will remain crucial to ensure these novel applications offer tangible benefits. The integration of CFD with digitization and machine learning promises to open even more avenues for exploration, further expanding its usefulness in a growing number of fields.
New Advancements in CFD Software for Structural Analysis A 2024 Overview - Cadence Acquisition of BETA CAE Systems Reshapes Structural Analysis Landscape
Cadence's acquisition of BETA CAE Systems signifies a notable change in the field of structural analysis. This deal, valued at around $1.24 billion, strengthens Cadence's ability to perform multiphysics simulations, enabling a more comprehensive approach to engineering design. BETA CAE's established expertise in engineering simulation software is expected to help Cadence expand its reach into the structural analysis market, a sector estimated to be worth billions of dollars. The integration of these technologies into Cadence's existing offerings aims to broaden the company's impact in this domain. However, it's crucial to recognize that the structural analysis landscape is already quite competitive. Cadence will need to prove the value of this acquisition by delivering meaningful innovations and addressing the diverse and evolving demands of engineers. Successfully navigating the challenges of a rapidly changing and complex engineering environment will be key to realizing the full potential of this merger.
Cadence's acquisition of BETA CAE Systems signifies a notable shift in the field of structural analysis. It seems like a strategic move to bring together advanced simulation technologies and create a more unified approach to engineering challenges. The combination of BETA's strengths in finite element analysis and Cadence's expertise in areas like circuit design holds the potential for more complete multiphysics simulations, where multiple physical aspects can be considered simultaneously.
One can anticipate improvements in the user interface of the resulting software, hopefully making it easier to use and more efficient for engineers. This acquisition appears to be fueled by a shared desire for innovation, as both companies have expressed plans for collaborative research and development. We might see Cadence's footprint in the structural analysis market expand, potentially reaching new industries like automotive or aerospace where sophisticated simulations are increasingly important.
It's reasonable to expect that the integrated software will deliver enhanced performance, particularly as BETA's algorithms are incorporated into Cadence's hardware-focused design approach. This might translate to faster and more precise simulations. There's also a possibility that the combined software offers a greater degree of customization, allowing engineers to fine-tune their simulation environment for specific projects.
The acquisition also appears to be coupled with increased investment in research and development from Cadence, hinting at a long-term vision for further refining their structural analysis offerings. Furthermore, this combination might pave the way for exploration of new application areas, such as additive manufacturing and the design of smart materials—fields that depend heavily on detailed structural analysis.
However, it's worth acknowledging that integrating two companies' technologies and workflows can be a complex process. There's a possibility of integration hurdles that will need to be addressed carefully to ensure that the full potential of this merger is realized in actual engineering projects. The success of this acquisition hinges on effective management of these challenges to maximize the combined capabilities for the wider engineering community.
New Advancements in CFD Software for Structural Analysis A 2024 Overview - Future Trends in CFD Software Development for 2025 and Beyond
Looking ahead to 2025 and beyond, CFD software development is expected to undergo significant changes. One major trend is the growing integration of sophisticated machine learning algorithms. This integration has the potential to dramatically speed up simulations, boost accuracy, and improve user-friendliness, potentially making CFD accessible to a wider range of engineers. Furthermore, we anticipate CFD's use expanding beyond its traditional applications in fields like aerospace and mechanical engineering. It's likely to become a valuable tool in areas such as energy, environmental science, and even finance, as researchers and engineers discover new ways to apply its capabilities.
The trajectory of CFD software development suggests a future where it's more deeply integrated with other engineering technologies. We can expect to see tighter links with technologies like Engineering Virtual Reality (EVR) and enhanced capabilities due to ongoing developments in GPU technology, all of which may contribute to faster and more extensive design cycles. Additionally, the move towards integrating CFD with digital order models aims to streamline collaboration between experts and those with less CFD experience. While all these innovations are exciting, it's important to approach them critically. The practical value of these new features must be rigorously tested across a wide array of applications to ensure they deliver the promised improvements in accuracy and reliability. The validation process will be crucial for maximizing the impact of these advancements in diverse engineering disciplines.
The landscape of CFD software is poised for significant change in the coming years, with several intriguing trends on the horizon. We can expect to see a greater emphasis on the role of machine learning in enhancing simulation speed and accuracy, potentially making CFD more accessible to a wider range of engineers. While the core principles of CFD, rooted in the Navier-Stokes equations, will remain fundamental, the incorporation of ML promises to improve simulation outcomes for complex flow phenomena like turbulent flows.
It's likely that CFD will move beyond its traditional stronghold in sectors like aerospace and mechanical engineering, branching into a broader range of industrial applications. We may see it integrated into tools like Engineering Virtual Reality software, offering new avenues for visualization and user interaction. The goal appears to be to democratize CFD, making it more accessible for engineers who aren't necessarily CFD experts, while simultaneously allowing specialists to push the boundaries of high-fidelity simulations.
The driving force behind many of these developments seems to be the constant pursuit of faster and more accurate design cycles, fueled by advancements in GPU technology. Initiatives like CFD Vision 2030 are trying to guide this development, focusing on addressing challenges and charting a path towards future milestones.
The integration of CFD within digital models is also likely to become increasingly important, potentially leading to easier implementation by a larger pool of engineers. The hope is to streamline the design process by enabling seamless transitions between design stages and simulations. There's a strong possibility that CFD will eventually become a universally applicable tool, benefiting from ongoing progress in the field.
Looking ahead, it's crucial that progress towards CFD's various goals is closely monitored and assessed. The CFD Vision 2030 roadmap is a valuable resource for guiding development and facilitating industry collaboration, ensuring that these innovations translate into real-world benefits. Ultimately, this continuous tracking of milestones will help to steer the development of CFD in a direction that optimally serves the engineering community. While it's hard to predict the exact path, one thing is certain: the future of CFD will be shaped by these exciting developments, making it an increasingly powerful tool for engineers across disciplines.
Revolutionize structural engineering with AI-powered analysis and design. Transform blueprints into intelligent solutions in minutes. (Get started for free)
More Posts from aistructuralreview.com: