Revolutionize structural engineering with AI-powered analysis and design. Transform blueprints into intelligent solutions in minutes. (Get started for free)
New Polarization Fraction Measurement Technique Enhances AI-Driven Structural Analysis
New Polarization Fraction Measurement Technique Enhances AI-Driven Structural Analysis - Polarization Fraction Measurement Revolutionizes AI Structural Analysis
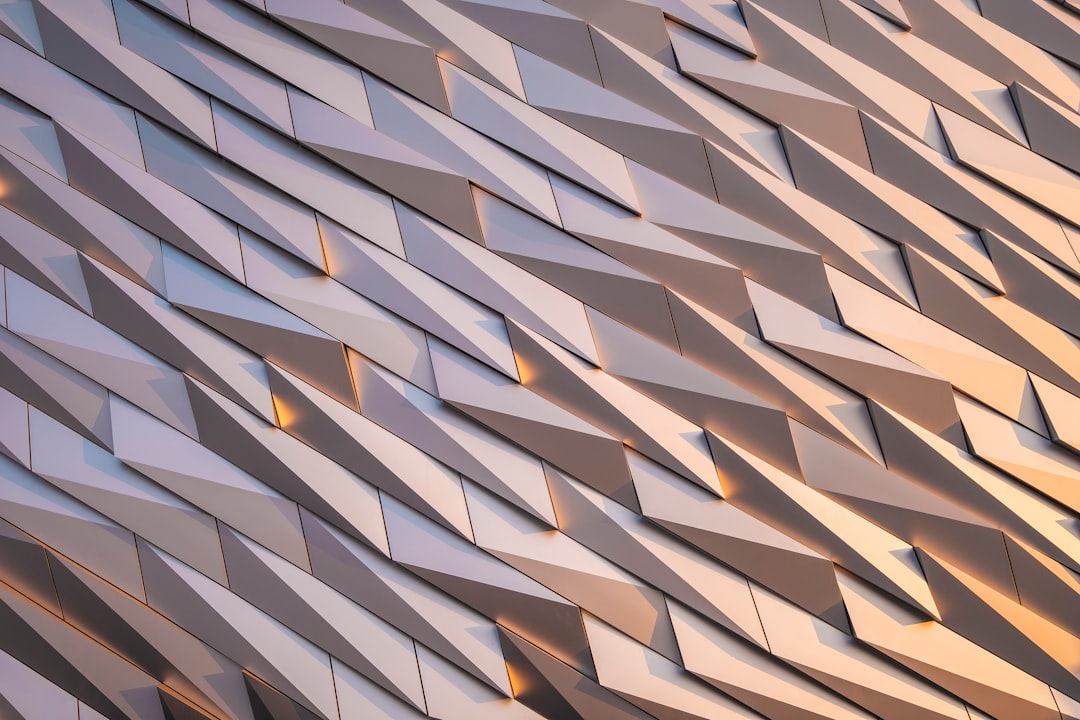
A new approach to measuring polarization fraction has emerged, significantly impacting how AI analyzes structures. This technique, relying on advanced deep learning, provides a more accurate assessment of polarization fractions in diverse fields like particle physics and optics. The accuracy improvements are particularly noteworthy because they address a long-standing issue—the challenge of reliably measuring Stokes parameters, especially when dealing with spatial variations and inherent noise within data. These advancements offer the potential to reshape structural analysis methods across various sectors, pushing the boundaries of both scientific discovery and industrial applications. It's still early, but the integration of polarization fraction measurement and AI holds the promise of profoundly altering how we understand and analyze structural features in materials and systems. While further development and refinement are needed, it's evident that this new technique could become a vital tool for future research and development.
A fascinating development in the field of structural analysis is the integration of AI with a new polarization fraction measurement technique. This approach utilizes deep learning to decipher polarization fractions, which are essentially indicators of structural properties like stress and strain. What's particularly noteworthy is the ability to measure these fractions in real-time and with spatial resolution, addressing a longstanding challenge in accurately quantifying Stokes parameters.
The core of this method lies in leveraging the connection between material stress and its unique polarization signature, offering a contactless way to monitor structural integrity. This opens up potential for diverse applications, ranging from traditional engineering materials like metals and composites, to more unexpected ones like biological tissues. However, it's crucial to acknowledge that environmental conditions like temperature or humidity can influence these polarization readings, highlighting the ongoing effort to standardize measurements and compensate for these factors.
Furthermore, this approach shows great potential for improving fault detection. It has been experimentally demonstrated that incorporating polarization fraction into AI analysis can significantly enhance the ability to find defects, and even predict material failure. While this advancement holds considerable promise, questions remain regarding the long-term reliability and consistency of these measurements across different material types and conditions. The upcoming release of data from projects like the Planck satellite, could significantly advance the precision of these techniques and further refine our understanding of polarization-based structural analysis.
In conclusion, this convergence of polarization fraction measurement with AI could be a game-changer. The capability of capturing intricate structural characteristics through subtle changes in polarization signatures offers a unique and potentially highly valuable analytical tool for researchers and engineers across various domains. The prospect of enhanced fault detection, predictive maintenance and a shift towards non-destructive testing methods for structural evaluation is quite promising, though rigorous validation and standardization are needed for wider adoption.
New Polarization Fraction Measurement Technique Enhances AI-Driven Structural Analysis - SPLASH Technology Automates Molecular Structure Analysis
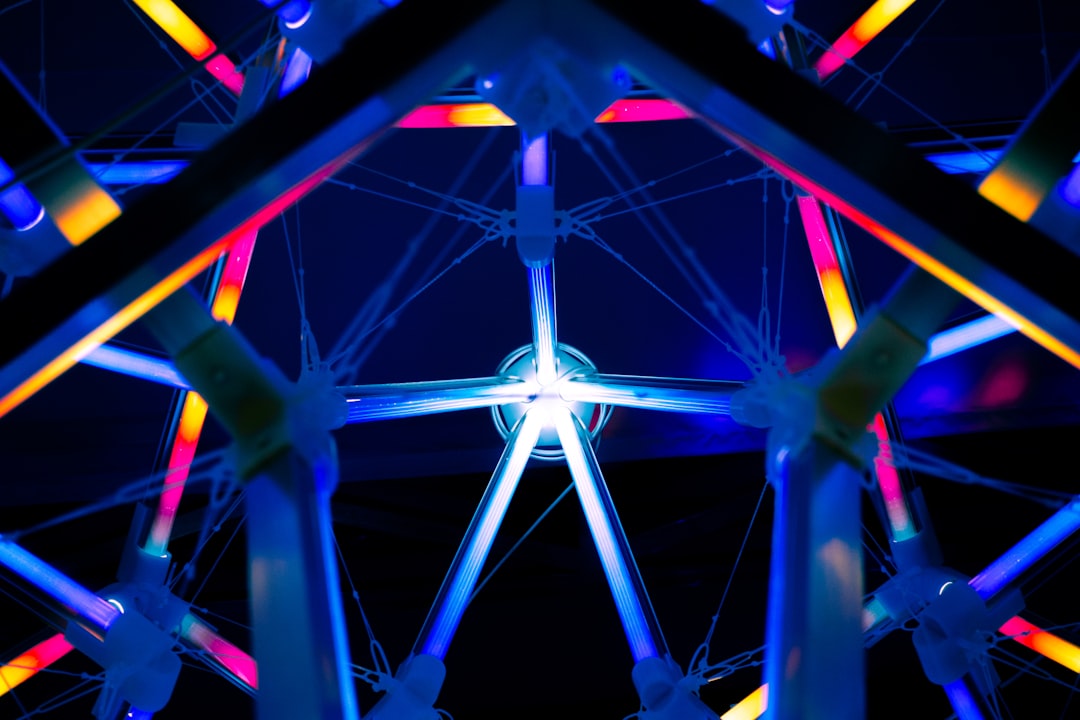
SPLASH Technology offers a novel approach to automating the analysis of molecular structures. It utilizes AI-driven techniques to expedite and refine the process of evaluating these complex structures. This automation is particularly relevant in fields like drug discovery, where understanding and optimizing molecular properties is paramount. The integration of advanced measurement techniques, such as those involving polarization fractions, further enhances the capabilities of SPLASH. These techniques aim to provide more precise and detailed information about a molecule's structural characteristics. While promising, the reliability and robustness of these AI-driven methods are still under development and need to be thoroughly validated. The goal is to create a system capable of handling diverse molecular structures and consistently providing accurate results. The ongoing work in this field will likely lead to further refinements in the SPLASH technology, making it a potentially valuable tool for researchers across chemistry, materials science, and beyond.
SPLASH technology employs AI-driven methods to automate the analysis of molecular structures, aiming for faster and more precise structural assessments. This approach focuses on leveraging polarization fractions – essentially, the unique polarization signatures linked to specific structural properties like stress and strain within molecules. A key aspect is the capability of non-contact analysis. By observing these polarization signatures, SPLASH can glean information about a material's structural behavior under stress without physically interacting with it, a significant step forward in non-destructive evaluation.
One of the significant hurdles SPLASH tackles is the presence of noise that often muddies polarization measurements. The algorithms embedded in SPLASH are designed to filter out this interference, improving the accuracy of Stokes parameter readings, which are vital for evaluating structural integrity. It's intriguing to see how SPLASH's applicability extends beyond traditional materials like metals and composites. The potential for studying biological tissues with this technique opens up exciting avenues in biomaterials research.
Rather than relying on simpler approaches, SPLASH analyzes the complete four-component Stokes vector. This comprehensive approach provides a more detailed picture of polarization states, giving it a greater sensitivity to subtle structural changes compared to traditional techniques. Integrating SPLASH into existing manufacturing processes could establish a dynamic quality control system, where structural integrity is monitored continuously during production. This idea of "in-line" structural health monitoring could be game-changing.
Beyond testing, SPLASH offers exciting possibilities for predictive analysis. Its deep learning algorithms hold the promise of forecasting material failures before they happen. This predictive capability could revolutionize maintenance strategies across various industries. However, it's essential to acknowledge that environmental factors like temperature variations can affect polarization readings. Researchers are continuously working on incorporating compensatory algorithms to improve the reliability of SPLASH across different conditions.
Preliminary studies indicate SPLASH's potential to redefine fault detection methods. The results suggest it may have a higher sensitivity for detecting defects compared to conventional methods, which could prove invaluable in applications where structural integrity is critical. Furthermore, the upcoming influx of data from satellite projects like Planck could significantly enhance the accuracy and refinement of SPLASH technology. This potential to leverage new, large-scale datasets to fine-tune polarization measurements could propel innovations in molecular structure analysis and understanding.
New Polarization Fraction Measurement Technique Enhances AI-Driven Structural Analysis - 2D Materials Enhance Optical Polarizer Efficiency
Two-dimensional (2D) materials are increasingly being utilized in optical polarizers due to their unique ability to absorb light differently depending on its polarization. This characteristic makes them well-suited for developing advanced polarizers. Researchers have explored various designs using 2D materials, leading to the development of polarizers like spatial-light, birefringent, and integrated waveguide devices. These 2D material-based polarizers have proven valuable in numerous applications, including spectroscopy and biomedical imaging.
Interestingly, the interaction between 2D materials and circularly polarized light generates intriguing optical effects like valley polarization and the photogalvanic effect. These phenomena, while still being explored, demonstrate the potential for further refining the optical characteristics of 2D materials. Moreover, techniques like electrolyte gating are being used to manipulate the properties of 2D materials, allowing for exploration of new physics and potential improvements in optical performance. These developments have implications for future optoelectronic devices. While 2D materials show great promise for enhanced polarizer efficiency, continued research is crucial to understand their full potential and tackle any limitations, such as how environmental factors might influence the accuracy of measurements.
Two-dimensional (2D) materials, like graphene and transition metal dichalcogenides (TMDs), have emerged as potential game-changers for optical polarizers. Their inherent properties allow for significant improvements in performance compared to traditional materials. For instance, they can achieve higher quantum efficiencies, which can lead to substantial improvements in polarization-based technologies.
The ability to produce extremely thin films, often just a single atom thick, is another key advantage. This means 2D-material based polarizers can be much lighter and require fewer resources, a compelling aspect for applications with strict size and weight limitations. Interestingly, these 2D materials are also highly tunable. By adjusting their structure or layering, we can control their band gaps, leading to more precise optical filtering. While conventional polarizers often work well within a limited range of wavelengths, 2D materials have shown the ability to operate efficiently across a wide spectrum, from ultraviolet to terahertz frequencies.
These materials also generally exhibit a higher tolerance to variations in the angle of incoming light. This characteristic is valuable in real-world optical systems, where perfect alignment is often difficult to achieve. However, challenges still exist. A crucial concern is their sensitivity to environmental factors like moisture. How these materials hold up over time in various atmospheric conditions remains a major question needing further research.
Further expanding their potential are the fascinating nonlinear optical properties they possess. The ability to manipulate and generate new frequencies of light opens the door for advanced polarizers and devices. Coupling them with existing nanostructures offers a path towards building highly functional yet miniaturized optical systems. It's intriguing to see how plasmonic effects, enabled by combining them with metallic structures, enhance light-matter interactions, possibly leading to increased efficiency and new ways of designing optical devices.
Looking ahead, the concept of stacking multiple layers of 2D materials to create three-dimensional polarization structures is a very promising avenue. This opens up the possibility of much more complex optical responses. Such systems could bring about exciting advancements in areas like optical sensing and imaging. While the field is still relatively young, 2D materials are demonstrating significant potential to revolutionize polarizer technology and expand the capabilities of polarization-based applications in a variety of scientific and engineering fields.
New Polarization Fraction Measurement Technique Enhances AI-Driven Structural Analysis - High-Dimensional Information Capture Through Advanced Sensors
The ability to capture high-dimensional information using advanced sensors represents a notable step forward in optical sensing and measurement. Recent work focuses on developing sensors that can record a wider range of optical properties beyond just light intensity. This includes capturing spectral information, polarization states, and even phase variations. These multi-parameter sensors, often based on innovative photodetector designs, create a much richer dataset for analysis. The benefit is a deeper understanding of the objects and materials being studied.
Incorporating these advanced sensors enhances the effectiveness of AI-driven structural analysis. By providing a more complete picture of the interaction of light with materials, these high-dimensional datasets allow for more accurate and nuanced analysis. Some researchers are exploring techniques like polarization multiplexing, using lasers in conjunction with holographic methods, to encode and transmit complex information from these new sensors. However, the complexity of the data generated by these advanced sensors presents its own challenges. The algorithms used for analysis must be able to extract meaningful information from multiple dimensions, while simultaneously accounting for environmental influences on the readings. Additionally, the sheer volume of data generated requires advancements in data storage and processing capabilities. Ultimately, the potential of these high-dimensional information capture techniques to transform how we understand and analyze materials and structures is evident, but addressing these challenges will be crucial for realizing their full impact.
Recent advancements in sensor technology have opened new avenues for capturing high-dimensional optical information, including intensity, spectrum, polarization, and phase. This ability to gather multiple data dimensions from a single measurement is revolutionizing how we analyze structures, particularly when combined with AI.
For instance, researchers have developed dispersion-assisted photodetectors that can reconstruct complex optical signatures with remarkably low errors, around 18.79 for spectral information and 1.47 for polarization. This suggests that we are gaining more detailed insights into the behavior of materials.
The use of polarization-resolved photodetectors, which can measure both light intensity and polarization states, offers richer information about material surfaces, revealing intricate features like shading and roughness. This level of detail is essential for a deeper understanding of material behavior and response under stress.
Interestingly, combining vertical-cavity surface-emitting lasers (VCSELs) with polarization multiplexed holography allows for the transmission of these high-dimensional datasets. Furthermore, integrating a microlens array and an imaging sensor onto a thin film creates an ultraintegrated high-dimensional imager that needs no alignment and can perform a complete measurement with just one acquisition. These ingenious approaches demonstrate the push for more compact and efficient systems.
It's exciting to see how these advances leverage emerging technologies in data transmission and storage to capture and process high-dimensional information that was previously beyond the reach of conventional optical methods. Researchers are also exploring the use of two-dimensional materials with unique crystal structures for developing more compact and efficient polarization-sensitive photodetectors. This is especially pertinent to infrared wavelengths, which are critical for heat-seeking technologies like thermal imaging and missile guidance.
These enhanced measurement techniques are crucial not just for applications in engineering materials, but also for the analysis of biological tissues and other previously unapproachable realms. As these methods evolve, it’s critical to address the sensitivity of polarization measurements to environmental factors like temperature and humidity. Ongoing research in this area focuses on building in better compensation for these factors to ensure that the measurements remain reliable across a wider range of conditions. While the promise of these advancements is significant, we need a continued focus on developing and standardizing these measurement techniques to ensure consistent and accurate results, and this will be critical as we expand the applications in diverse fields.
New Polarization Fraction Measurement Technique Enhances AI-Driven Structural Analysis - Light Polarization Measurement Applications Expand Across Industries
The use of light polarization measurement is expanding across a wide range of industries, driven by improvements in optical technologies. The development of very thin 2D materials and sensors capable of measuring many dimensions of light has greatly improved the accuracy and efficiency of polarization measurements. This has led to a broader range of applications, from communication systems to medical imaging. Furthermore, combining AI with these measurements is revolutionizing how we analyze structures, enabling real-time monitoring and the detection of defects in various materials. However, environmental conditions like temperature and humidity can still affect these measurements, and addressing these influences is a key area of ongoing research to ensure the reliability of polarization-based techniques for various industrial needs. This intersection of advanced technology and optical science hints at the development of even more sophisticated and reliable measurement systems in the years to come.
The field of light polarization measurement is expanding rapidly, with applications spreading across diverse industries. We're seeing a growing need to manage polarization in optical communications, imaging, and sensing, largely due to issues like polarization mode dispersion, which can distort signals. Interestingly, 2D materials are emerging as a new generation of optical polarizer materials. They show unique anisotropic absorption characteristics for different polarization states and could lead to far more efficient and compact polarizer devices. A recent development involves using ultrathin 2D metasurfaces to create holograms for directly measuring polarization, which could benefit fields like spectroscopy, sensing, and communication.
The integration of AI in structural analysis is gaining traction, especially with the introduction of these new polarization measurement techniques. The ability to analyze polarization fraction data, which are essentially indicators of stress and strain, can improve AI models' ability to predict and understand material behavior in diverse materials, including those found in biological systems. This could lead to improved methods of fault detection, which would be very helpful for safety-critical infrastructure.
However, polarization measurement can be challenging. Environmental factors, like temperature and humidity, can interfere with measurements. Researchers are actively developing methods to minimize these environmental effects and improve the reliability of data. Optical coherence tomography (OCT) is an interesting example of how polarization measurements are being combined with other techniques to provide more intricate, high-resolution imagery for structural analysis.
There's a lot of interest in on-chip technologies for polarization management, which is likely to open doors for applications in a wide range of fields. Silicon photonics, for example, has attracted significant attention due to its potential for use in light-based electronic devices. These developments in silicon photonics suggest innovation will continue in optical networking and signal processing. In addition to the use of 2D materials and metasurfaces, quantum-enhanced polarization techniques are developing rapidly. These techniques offer improvements in sensitivity and noise reduction, which is very important for detecting subtle changes in material properties.
The ability to measure polarization and the associated phase shifts is also showing promise. This dual-parameter approach could revolutionize a variety of polarization-dependent applications. The merging of AI with these polarization-based methods is leading to more powerful structural analysis techniques that can potentially lead to breakthroughs in material science and engineering, where understanding material failure modes is a key challenge. Ultimately, the advancements in polarization measurement, particularly with the help of AI, could be game-changing for many industries, though standardization and understanding environmental influences on measurement accuracy are crucial for the wider adoption of these powerful tools.
New Polarization Fraction Measurement Technique Enhances AI-Driven Structural Analysis - Deep Learning Algorithms Match Human Performance in Image Recognition
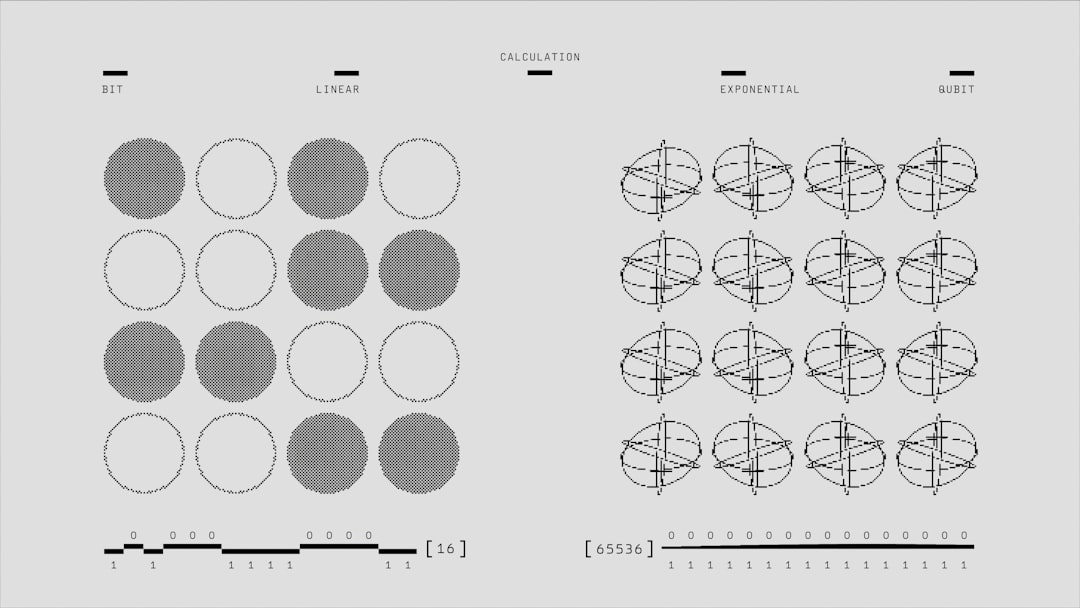
Deep learning algorithms have made significant strides in image recognition, often demonstrating performance on par with, or even exceeding, human capabilities across a range of tasks. This is especially evident in areas like medical imaging, where the algorithms' ability to identify diseases from medical images has shown great promise. Evaluating the effectiveness of these algorithms involves using metrics like accuracy, as well as the development of newer assessment methods like "minimum viewing time." These advancements underline the increasing sophistication of AI models in image processing. However, limitations still exist, especially when dealing with images that have been distorted or altered. There's still active research focused on overcoming these hurdles. The ongoing development of deep learning techniques and their integration into diverse applications, particularly in healthcare, warrants continued exploration and careful consideration of the broader implications.
Deep learning algorithms have shown impressive potential in image recognition, often achieving performance levels comparable to, or even surpassing, human capabilities in specific tasks. This is especially evident in situations involving complex patterns and large datasets. However, their reliance on extensive training data can be a limiting factor. When trained on limited or biased data, the algorithms can struggle to generalize their knowledge to new, unseen scenarios—a challenge that highlights a key difference compared to human adaptability.
The structure of these deep learning systems plays a crucial role in their performance. For example, convolutional neural networks (CNNs), specifically designed for image processing, have proven to be particularly effective at capturing spatial relationships within images. Their performance often outstrips traditional machine learning methods, thanks to their ability to effectively recognize localized patterns.
Interestingly, researchers have discovered that carefully designed “adversarial examples” can deceive these deep learning systems into misclassifying images. This finding underscores a vulnerability inherent in some of these algorithms, which is not typically present in human visual processing. This leads to questions about the robustness and reliability of deep learning systems in real-world scenarios.
The concept of transfer learning—where a model trained for one task is repurposed for another—is gaining attention as a potential way to reduce the need for extensive training. This intriguing concept parallels human learning abilities, but it's still uncertain how effectively this translates across diverse application domains. Additionally, there’s a notable sensitivity to subtle changes in image inputs. Even small alterations can lead to vastly different outcomes from deep learning models, which is a characteristic not typically seen in human visual systems.
This trend towards deep learning has led to a rethinking of the role of feature engineering in traditional machine learning. There is a growing consensus that "end-to-end" learning—directly processing raw image data—can sometimes outperform methods that rely on manually crafted features. However, this comes with a significant computational cost. Training these deep learning models often requires significant resources, which can be a significant barrier in certain fields that value efficient and resource-conscious solutions.
Although deep learning algorithms have achieved remarkable accuracy in image recognition, it’s important to remember that they often lack the capacity for genuine understanding. While they might excel at detecting patterns and features, they frequently fall short when it comes to comprehending the broader context or semantic meaning of an image. This gap demonstrates a fundamental difference between machine and human visual information processing.
On the other hand, these algorithms can process images at a speed that is far superior to human capabilities. This high processing speed is critical in applications such as real-time surveillance and medical imaging, where rapid interpretation and decision-making are crucial for effective outcomes.
Overall, deep learning algorithms are steadily improving in image recognition tasks. These advances offer potential benefits in a variety of fields, but there's still a lot we need to understand about their strengths and limitations. As the field evolves, it's crucial to continue exploring their potential while carefully considering their vulnerabilities and the challenges they pose compared to human visual cognition.
Revolutionize structural engineering with AI-powered analysis and design. Transform blueprints into intelligent solutions in minutes. (Get started for free)
More Posts from aistructuralreview.com: