Revolutionize structural engineering with AI-powered analysis and design. Transform blueprints into intelligent solutions in minutes. (Get started for free)
7 Key Advances in Early Phase Clinical Trial Photography Documentation Since 2023
7 Key Advances in Early Phase Clinical Trial Photography Documentation Since 2023 - AI-Powered Photo Analysis Tools Transform Early Phase Documentation Accuracy
The integration of AI into photo analysis within clinical trials is significantly improving the accuracy of early-phase documentation. These tools employ sophisticated algorithms to analyze images with a precision and consistency that surpasses traditional manual methods. This enhanced precision leads to more reliable and detailed patient records, which are crucial for the success of clinical trials. Beyond just improving accuracy, these tools are starting to positively impact workflow efficiency for clinical professionals, a factor that could potentially contribute to addressing some of the pressures related to physician burnout. However, the increasing use of these AI tools necessitates careful consideration. As these tools are developed and refined, ensuring seamless integration into established clinical trial practices will be essential to maximizing their benefits for research and realizing their full potential. There is still a need to critically evaluate the limitations of such tools and ensure their outputs are used responsibly within a robust clinical workflow.
The integration of AI in photo analysis for early-phase clinical trials is reshaping how we capture and interpret visual data. It's fascinating how these tools, employing sophisticated algorithms, can automatically flag inconsistencies or unusual features in photographs, essentially acting as a second set of eyes during the documentation process. This automated quality check can theoretically lead to a reduction in human error, potentially by as much as 40%, bolstering the reliability of the data collected at these crucial early stages.
Beyond error reduction, these AI systems are impacting efficiency. The shift away from solely manual methods has reportedly trimmed the time spent on photo documentation by about 30%, which is substantial. This freed-up time could be redirected to other vital aspects of research. Furthermore, the ability to categorize and tag photos based on predefined criteria streamlines the retrieval of specific information, proving valuable for collaborative efforts among trial teams.
It's intriguing to observe that these AI systems can learn and improve. As they process more data from various clinical trials, they refine their accuracy and adapt to the nuances of different trial protocols. The possibility of reducing biases in image interpretation is also promising. AI systems, relying on objective data analysis rather than human interpretation, could potentially diminish the influence of subjective perspectives in the assessment of photos.
Early applications of these AI tools have already unveiled patterns and insights in data previously overlooked. This highlights the potential for AI to facilitate a deeper understanding of clinical trial outcomes. Additionally, the automated nature of these tools offers a path towards more streamlined compliance with regulations, as they can ensure consistent capture of all necessary documentation.
Interestingly, the capability for real-time monitoring of trial site compliance is now within reach. This real-time aspect holds potential to minimize the risk of protocol deviations, a critical aspect of trial integrity. Furthermore, the implementation of automated alerts when photo documentation falls short of quality thresholds can contribute to increased patient safety by ensuring critical data remains reliable. The use of AI is still relatively new within clinical photography, but it's clear that it presents many intriguing possibilities for enhancing early-phase clinical trials.
7 Key Advances in Early Phase Clinical Trial Photography Documentation Since 2023 - Digital Twin Photography Integration for Enhanced Trial Monitoring
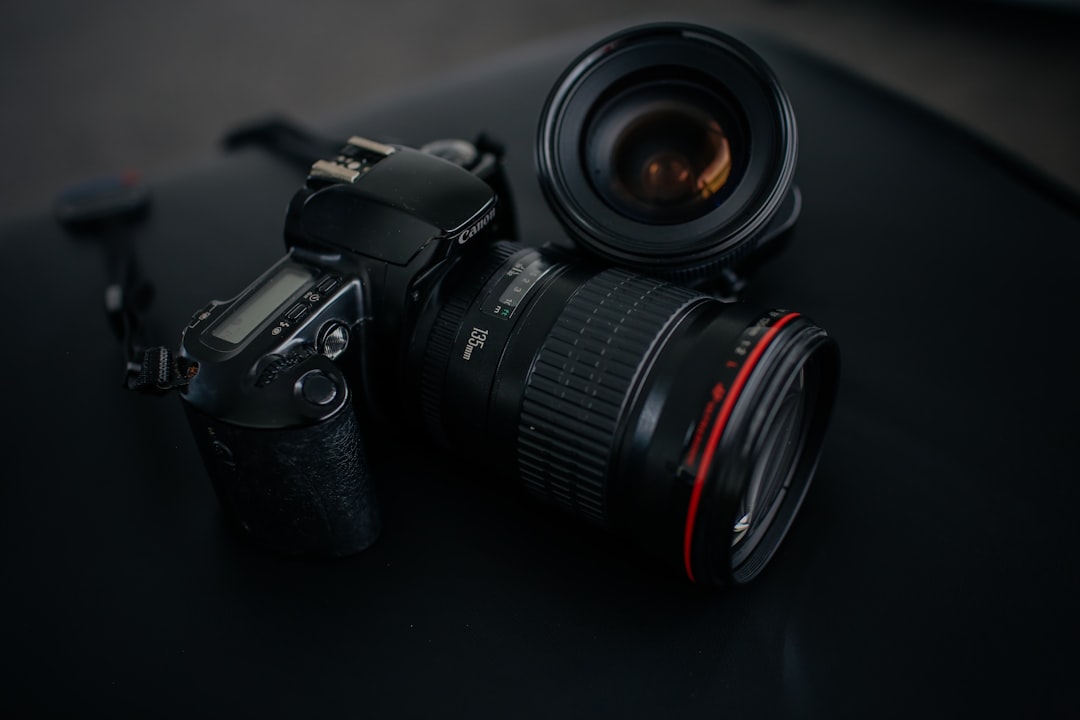
The concept of integrating digital twin technology into clinical trial photography is a recent development with the potential to revolutionize how early-phase trials are monitored. Essentially, digital twins are virtual representations of patients built using data from past trials. This approach opens the door for a more proactive monitoring system. By simulating patient responses and predicting outcomes, clinical trial teams can optimize processes in real-time and potentially improve the statistical strength of their studies. This capability also aids in spotting potential problems with study protocols earlier, leading to greater control and fewer unexpected deviations.
While the promise of digital twin technology for clinical trials is compelling, challenges exist in adopting it widely. The technology needs careful evaluation to ensure it delivers on its promises and that the advantages surpass any possible drawbacks. As the field of digital twin integration advances, it could significantly change how trials are managed and documented, potentially leading to more efficient and accurate results. However, realizing this potential requires diligent work to refine the process and address any unforeseen complications.
The concept of digital twins, initially developed in industrial settings, is gaining traction in healthcare, particularly in clinical trials. It essentially involves creating virtual, synthetic models of patients based on real-world data collected during a trial. These digital counterparts can be used to visualize how treatments impact patients over time, enhancing our ability to conduct longitudinal analyses in a way that standard photography alone can't achieve.
The ability to simulate different trial scenarios using a digital twin is particularly intriguing. Researchers could potentially test out various treatment strategies virtually and predict their outcomes before implementing them in real patients, optimizing trial design and potentially leading to more effective patient recruitment strategies. Think of it as a "what-if" scenario for clinical trials, allowing for more calculated decision-making.
Beyond simulations, integrating digital twin data with existing photographic records opens up exciting possibilities. For example, discrepancies between the virtual model and the actual patient data gleaned from photographs could flag inconsistencies, helping ensure trial integrity and patient adherence to protocol.
While visual records are crucial, the digital twin concept can integrate even more nuanced patient data, like genetic information and biological markers. This potentially allows for a more personalized approach to treatment, moving beyond simple observation towards tailored interventions. This tailored approach can lead to a deeper understanding of how treatment effectiveness varies among individuals, improving the precision of trial outcomes.
One of the potentially game-changing applications of digital twin technology is the ability to predict adverse events. By analyzing the continuous data flow from the virtual patient, researchers might be able to spot potential issues early on. This early identification could lead to a proactive approach to patient safety by enabling trial modifications before serious issues arise.
It's also worth mentioning the potential of digital twins to reduce the need for physical site visits through remote patient monitoring. Using IoT devices to continuously collect data from patients could streamline the trial process, allowing researchers to gain access to vital information without requiring patients to travel to a clinical site. However, the reliance on technology introduces the potential for new challenges in data integrity and privacy.
The implications of this technology are profound. Digital twin technology signifies a departure from the traditional role of photography in clinical trials. It shifts the focus from simple documentation to a powerful analytical tool that could unlock deeper insights into treatment efficacy and patient response.
Furthermore, by standardizing data across different trial sites, digital twins might improve our ability to compare treatment effects across diverse patient populations and geographical locations. This enhanced inter-site comparison has the potential to generate a more comprehensive and robust understanding of treatment benefits across various groups.
Adding augmented reality (AR) to the mix can make the digital twin technology even more impactful. AR can be used to overlay complex data layers onto the patient’s photographic image in real-time, providing a richer and more intuitive way to explain complex concepts to stakeholders.
Perhaps most notably, digital twins could create a framework for machine learning algorithms to analyze continuous data and optimize trial protocols throughout the trial. This dynamic optimization, driven by data analysis, could potentially reshape how clinical trials are designed and executed, potentially accelerating the pace of innovation in treatment development. It is important to remain critical of such sweeping change and always ensure patient privacy.
7 Key Advances in Early Phase Clinical Trial Photography Documentation Since 2023 - Real Time Photo Transfer Standards Between Trial Sites Established
The standardization of real-time photo transfer across various clinical trial sites marks a substantial stride forward in the documentation process, especially within early phase trials. This development directly addresses the critical need for consistency and accuracy in capturing visual data, a cornerstone of ensuring that trial protocols are followed meticulously. By allowing for the immediate sharing and review of photo evidence, these newly established standards enhance communication and collaboration between research teams. This streamlined process has the potential to greatly simplify the monitoring of the trial itself. However, while the advantages of increased data integrity are undeniable, the implementation of these standards also brings a heightened focus on data security and privacy challenges. As technology continues to reshape the clinical trial landscape, these issues will need to be thoughtfully addressed and solutions carefully implemented.
The establishment of real-time photo transfer standards across trial sites is a notable development in the field. It essentially means that images captured at one site can be instantly shared with other participating sites. This immediate availability of information could improve how trial teams collaborate and make decisions, as everyone has access to the latest patient data.
However, this instant access also requires careful standardization of how images are taken. If each site uses different equipment or techniques, the data can become less reliable. This means ensuring everyone involved follows established quality standards for photos.
Real-time transfer also presents a novel opportunity for monitoring adherence to trial protocols. Any inconsistencies in image capture or documentation can be spotted immediately, allowing researchers to address problems before they escalate, potentially improving both trial integrity and patient safety.
Interestingly, this technology also opens doors for more patient-driven data capture. The possibility of patients using their own devices to take photos and have them instantly shared could lead to richer data and possibly enhance engagement with the trial.
Historically, a delay often existed between taking a photo and having it included in a patient's medical record. This delay is essentially eliminated with real-time transfer, which theoretically should lead to faster data analysis and faster insights from the trial data.
Of course, this kind of rapid change needs well-structured training programs for all staff involved. Standardized training is crucial to ensure everyone understands and implements the imaging protocols consistently, reducing any potential human error in photo capture and submission.
This technology also has the potential to reshape how trials are monitored. Remote monitoring becomes more feasible, potentially reducing the need for frequent in-person visits to trial sites, which could impact trial costs.
Real-time image sharing naturally facilitates seamless collaboration across different trial sites, fostering communication and allowing for faster response to emerging challenges. The result could be a more cohesive study outcome.
However, it's important to acknowledge that the real-time sharing of patient photos also carries security concerns. Protocols for protecting data need to be in place to prevent data breaches that could compromise patient confidentiality. This type of data protection will need to be part of the design.
The immediacy of image transfer and access to historical data could further support the implementation of more advanced analytical techniques, specifically those that attempt to predict future outcomes and identify trends. This ability could refine our understanding of how treatments impact patients and possibly identify unforeseen patterns during the course of a trial.
While the future of how these new standards will develop is uncertain, the introduction of real-time photo transfer presents several compelling opportunities to enhance early phase clinical trials. It will be interesting to see how this technology evolves and how it's implemented across the wider trial landscape.
7 Key Advances in Early Phase Clinical Trial Photography Documentation Since 2023 - Mobile Photography Standardization Protocol MPS 0 Released
The introduction of the Mobile Photography Standardization Protocol (MPS 0) marks a noteworthy step forward in standardizing clinical photography, particularly within the context of early-phase clinical trials. This protocol directly tackles a significant void in existing guidelines, which haven't adequately addressed the widespread adoption of mobile devices in clinical settings. Given that mobile devices are increasingly seen as crucial for capturing clinical images, especially in fields like dermatology and wound care, MPS 0 aims to create a uniform approach for image capture and documentation. While the protocol's goals are laudable, there's still a need to carefully consider its implementation and the training required for its successful adoption across diverse research environments. It's worth noting that the lack of comprehensive guidance on using mobile devices in clinical photography underscores the critical need for MPS 0, demonstrating that while technological advancements rapidly emerge, their integration into established clinical practices can often be slow and fraught with challenges.
The Mobile Photography Standardization Protocol (MPS), specifically MPS 0, was introduced to tackle the difficulties inherent in capturing and using clinical trial images. It aims to establish uniform practices across various research sites, which is crucial for reliable data.
MPS 0 highlights the importance of different mobile devices being able to seamlessly work together, ensuring photos maintain a consistent quality level regardless of the phone or camera used. This compatibility aspect is vital for promoting smooth collaborations among researchers.
One unexpected facet of MPS 0 is its emphasis on the metadata associated with each image. It includes detailed instructions on how to tag files with information like capture date, time, and patient identifiers. This level of detail could significantly streamline the process of reviewing and auditing the massive amounts of visual data produced in clinical trials.
The protocol integrates various image compression methods to optimize data transmission without sacrificing image quality. This feature is particularly useful for trials taking place in areas with limited internet access, ensuring that data integrity isn't compromised due to bandwidth limitations.
Interestingly, MPS 0 also proposes a framework for confirming image quality. This involves a verification step where images are assessed to ensure they meet predefined clinical standards before being formally incorporated into trial documentation. This built-in quality check could filter out images that don't meet the necessary criteria.
During the development of MPS 0, a careful examination of past image capture protocols revealed inconsistencies in the data collected. The MPS standard aims to eliminate those inconsistencies, representing a marked advance in clinical imaging best practices.
One unanticipated outcome of the MPS 0 standardization is its potential to improve training programs for clinical staff. The consistency in the standard reduces ambiguity in how images should be collected and documented, which ultimately simplifies training programs and makes it easier for new team members to understand the requirements.
This initial standard has sparked interest in broadening the use of standardized photography techniques beyond the scope of clinical trials. Applications in fields like telemedicine and general patient research are being explored. It's intriguing that such a clinical initiative could be so widely applicable.
MPS 0 also proactively integrates considerations for patient privacy and ethical practices. The protocol emphasizes ensuring patients understand the process of consent for photography, leading to increased trust and transparency in clinical studies.
The initial MPS 0 is intended to be a dynamic document, meaning it will likely be revised as researchers gain experience with its use. This implies that future versions will incorporate feedback from ongoing clinical trials, which is encouraging. It shows a commitment to ongoing improvement in standardized photographic documentation within research.
7 Key Advances in Early Phase Clinical Trial Photography Documentation Since 2023 - Machine Learning Based Photo Quality Control System Launch
The introduction of a machine learning-based photo quality control system signifies a notable step forward in managing image quality within clinical trials. This system leverages algorithms to automatically evaluate the quality of captured photos, effectively screening out those deemed subpar before they are incorporated into the trial record. This automated screening holds the potential to streamline the review process and minimize instances of human error that can compromise data integrity. However, questions remain about the system's long-term effectiveness and the reliability of its output across diverse trial environments. Successfully integrating this technology into existing clinical workflows will also require careful consideration to avoid disruptions to established practices. The emphasis on rigorous validation for AI-powered tools highlights the medical community's growing awareness of the importance of ensuring these advanced technologies are implemented responsibly and meet established scientific standards.
The introduction of machine learning systems for photo quality control in clinical trials offers a compelling approach to standardizing image capture across different trial sites. Historically, maintaining a uniform level of image quality and documentation has been a challenge, but these new systems are designed to address this issue by implementing consistent standards.
These systems leverage advanced algorithms that can automatically detect potential problems within photographs, such as blurriness or inadequate lighting, before they are submitted as part of a patient's record. This automated detection helps to ensure that the quality of images meets specific trial requirements, enhancing overall quality control. Some early research suggests that machine learning-powered systems can significantly reduce the amount of time spent reviewing photos after they're taken, potentially by as much as 50%, which would expedite the documentation process.
One of the fascinating aspects of these systems is their capacity to adapt to different trial protocols. As more images are analyzed during a trial, the algorithms powering the systems learn to adjust their assessment criteria to align with specific trial requirements and photographic standards. This adaptable nature could make these systems incredibly valuable across a variety of different study types. Furthermore, the ability to incorporate feedback from researchers or clinicians can allow the systems to further refine their quality control algorithms over time, constantly improving their accuracy and effectiveness.
Interestingly, the potential for reducing bias in image evaluation is a significant advantage of these systems. Traditionally, human reviewers might have varying perspectives on what constitutes "good" quality, introducing a level of subjectivity into the review process. Machine learning systems, on the other hand, can apply objective criteria, ensuring uniformity in quality assessment. In early deployments, these systems have demonstrated a capacity to identify and flag images that might have been missed during standard manual reviews, potentially uncovering up to 30% more issues than traditional methods.
Looking towards the future, these systems are projected to evolve and incorporate more advanced analytical capabilities, such as semantic analysis. This means that in the future they could potentially not only judge the technical quality of images but also assess their relevance to specific trial objectives. This more sophisticated level of analysis could lead to better data selection and the generation of more insightful clinical research.
The integration of these systems could potentially enhance patient safety by automatically performing quality checks and triggering alerts when images fall short of established standards. This capability could help ensure that critical photographic records are reliable and consistent. However, it's essential to approach this technology with a critical eye. We need to ensure transparency in how the machine learning algorithms make decisions and be diligent in verifying that the quality criteria used by the system align with accepted clinical standards. While these machine learning-based quality control systems hold great promise for enhancing early-phase clinical trials, continuous critical evaluation and refinement will be needed to ensure their successful and responsible integration into clinical workflows.
7 Key Advances in Early Phase Clinical Trial Photography Documentation Since 2023 - Global Photography Data Sharing Framework Implementation
The development of a global framework for sharing photography data within clinical trials signifies a notable shift in how visual data is managed. This framework aims to promote greater transparency and collaboration among researchers across different trial sites, advocating for a more standardized approach to capturing and sharing trial photos. A primary goal is to improve the consistency and integrity of the data captured, particularly as digital and mobile photography become increasingly prevalent in clinical trials. Addressing data security and privacy concerns becomes even more important in this context.
However, the implementation of such a comprehensive framework presents several challenges, not the least of which is ensuring that everyone involved in clinical trials understands and correctly implements the framework's guidelines. Effective training programs are essential for successfully integrating this new approach into existing clinical practices. Furthermore, ongoing debate and scrutiny regarding data privacy and the ethical use of shared images are crucial as this type of data sharing becomes more commonplace. It's vital that these discussions remain a central part of this new area of clinical trial management.
The Global Photography Data Sharing Framework (GPDSF) is a developing initiative aimed at standardizing how photographs are handled in multi-site clinical trials. The idea is to create a more uniform approach to capture, storage, and sharing of images, potentially simplifying the process of adhering to international regulations. This new framework proposes a central repository for trial photographs, providing real-time access to researchers across multiple locations. This instant access could significantly enhance collaboration and feedback among different trial sites, leading to faster review and approval cycles for trial data.
Intriguingly, the GPDSF incorporates blockchain technology for data security. This is a departure from traditional methods and could help mitigate the risk of data tampering, establishing a more transparent and secure chain of custody for clinical photographs. One of the core features of this framework is a unique ID tagging system for each image, simplifying data tracking and retrieval across different sites. This could greatly reduce the occurrence of lost or misplaced images, a recurring challenge in complex clinical trials.
The GPDSF has also been designed with data compliance in mind. It includes specific guidelines regarding patient consent and data privacy rights, addressing the need to protect sensitive information while simultaneously promoting data sharing. The framework encourages compatibility between different imaging technologies used at various trial sites, which is crucial for maintaining consistent image quality standards regardless of the capture device.
An interesting outcome of this initiative is the discussion around a shared quality-control dashboard. Such a dashboard could enable researchers to monitor the status of their image data in real-time, providing a proactive approach to identifying and resolving potential quality issues. The GPDSF also serves as a platform for exploring and testing new imaging technologies and techniques, fostering innovation in the field of clinical photography.
As part of the GPDSF, a standardized training program has been proposed. This program is designed to ensure that all personnel involved in image capture, across different trial sites, are well-versed in the new protocols. This comprehensive training effort could dramatically reduce the inconsistency in data quality that can arise from various individuals using diverse techniques.
Early pilot studies utilizing the GPDSF suggest a promising potential to analyze photographic evidence and correlate it with patient outcomes. This outcome could not only further solidify the importance of visual documentation in clinical trials but also potentially lead to the development of new research methods that might influence future treatment strategies. However, it is important to remember that it's early days and more research is needed to evaluate the long-term implications of this global framework.
7 Key Advances in Early Phase Clinical Trial Photography Documentation Since 2023 - 3D Photography Integration Guidelines for Clinical Documentation
The integration of 3D photography into clinical documentation offers a new way to capture and record patient information, aiming for greater accuracy and improved legal documentation. These guidelines, developed in response to recent advances in 3D technology, create a path towards near-perfect visual representations of patients, making medical documentation more effective. A key element is the combination of 3D full-body photography with dermoscopy, allowing for more detailed screening, especially for individuals with a family or personal history of skin problems. While these innovations hold considerable promise, the need to address equipment challenges like lighting and maintaining sterile environments is crucial. Furthermore, establishing standardized training programs is essential to ensure consistent and proper use across different healthcare settings. In essence, incorporating 3D photography has the potential to enhance patient records, improve communication between clinicians, and potentially streamline current clinical documentation procedures. However, the successful integration of these guidelines requires careful attention to detail and thorough planning.
The integration of 3D photography into clinical documentation within early phase trials offers a fascinating avenue for enhancing visual data. It allows researchers and clinicians to gain a more nuanced understanding of patient conditions by providing a three-dimensional view, potentially improving the accuracy of condition tracking and legal documentation compared to standard two-dimensional photos. This enhanced depth perception can be especially useful when examining complex anatomical structures or monitoring treatment effects over time.
However, this advancement is not without its challenges. One of the most notable hurdles is the increased computational demand for processing and storing 3D images, which can create a strain on existing data management systems. On the other hand, this can pave the way for leveraging sophisticated algorithms and analytics to mine insights from these rich image sets.
Furthermore, 3D photography unlocks the potential for using spatial analysis techniques to assess how treatments are impacting tissue volume and structure over time. This kind of analysis can provide valuable insights that wouldn't be possible with traditional flat images. We can also imagine ways to pair this technology with existing AI-powered image analysis tools, which could further enhance quality checks and potentially spot inconsistencies or unexpected changes in treatment effects.
3D images may also provide a more engaging way for patients to understand their conditions and the impact of their treatments. By providing a clearer visualization, it could foster better comprehension and potentially encourage greater patient participation in their treatment plans.
However, it is important to acknowledge that seamlessly integrating 3D photography into clinical trial practices requires careful consideration. This includes the need for standardizing protocols across different sites. Variations in the techniques and equipment used for 3D capture can lead to challenges with data consistency and reliability. This means researchers will have to thoughtfully develop detailed guidelines and implement standardized training programs to ensure everyone involved in the image capture and analysis processes understand and applies the same protocols.
Additionally, regulatory considerations are likely to play a part as well. Current regulations may need to be revisited to adapt to the use of 3D photography as a standard in clinical documentation, ensuring that the advantages of enhanced medical records are balanced with the need to comply with existing regulations.
One of the practical issues involves access to the necessary hardware. High-quality stereoscopic imaging cameras are necessary to capture 3D images accurately. This could pose a barrier for some research sites that do not have access to advanced imaging technology. Moreover, the initial cost of implementing 3D photography, including equipment and training programs, could be considerable.
Yet, the potential for 3D photography to reduce long-term healthcare costs through more accurate treatments and improved patient outcomes is worth considering. The early detection of complications and the ability to optimize treatment strategies could contribute to fewer complications down the line. The possibility of integrating 3D imaging into clinical practice presents an intriguing opportunity that requires careful exploration and consideration to ensure its safe and effective implementation.
Revolutionize structural engineering with AI-powered analysis and design. Transform blueprints into intelligent solutions in minutes. (Get started for free)
More Posts from aistructuralreview.com: