Revolutionize structural engineering with AI-powered analysis and design. Transform blueprints into intelligent solutions in minutes. (Get started for free)
Cross-Sectional Plane Analysis Unveiling New Insights in AI Structural Design
Cross-Sectional Plane Analysis Unveiling New Insights in AI Structural Design - StructGANRule Revolutionizes Shear Wall Design
StructGANRule introduces a novel approach to shear wall design, utilizing a rule-embedded Generative Adversarial Network (GAN) to streamline the cross-sectional design process. This method addresses the challenges that conventional design rules pose for standard GANs, paving the way for quicker and more accurate designs, particularly in reinforced concrete shear wall structures commonly found in high-rise buildings. By incorporating deep learning principles, StructGANRule accelerates the design process and helps optimize designs for specific structural demands. This is achieved through techniques like the integration of ControlNet and StructDiffusion, which enhance the ability to factor in various design conditions. Tools such as AIstructureCopilot, which promise significant improvements in design speed while maintaining regulatory compliance, are emerging as a testament to the growing impact of AI-driven design in structural engineering. While there are still questions about the full scope of AI's role in design, this shift towards data-driven methodologies appears poised to reshape traditional practices in architecture, engineering, and construction.
StructGANRule presents a fresh perspective on shear wall design by employing generative adversarial networks. This approach has the potential to uncover optimized wall configurations that conventional methods might miss, which is exciting. It incorporates a design framework that's grounded in structural principles, ensuring that the generated designs adhere to established performance criteria. This allows engineers to explore a wider range of design options without sacrificing safety, which is always a crucial concern.
Early evaluations suggest that StructGANRule can potentially decrease material use by up to 20% without compromising structural integrity compared to traditional approaches. This finding indicates a potential improvement in resource efficiency. The speed of the algorithm is notable, with the capacity to rapidly produce a large number of design iterations. This accelerates the identification of optimal design solutions significantly compared to more traditional methods, which can be time-consuming.
The ability of StructGANRule to provide immediate feedback during the design process is also notable. This allows engineers to interactively adjust wall parameters and evaluate the effect on performance metrics in real time, leading to a more iterative and responsive design process. However, this tool has also initiated discussions regarding the role of human intuition and expertise in structural engineering. It raises interesting questions about whether AI can fully replace engineers' experience and judgment.
It's interesting that the algorithm is designed to learn from historical project data, including past performance. This could help in developing designs that are potentially more resilient in the face of challenges such as seismic activity. Integrating this tool into existing structural analysis software seems straightforward, making it accessible to firms looking to modernize their design process.
StructGANRule incorporates aspects beyond just pure structural performance in the optimization process. It leverages distance metrics to consider aesthetics alongside structural functionality. This broader design perspective expands the options for optimization beyond solely performance-driven outcomes. Finally, early studies have demonstrated that designs from StructGANRule may actually show enhanced performance under stress testing compared to traditionally generated designs, which is an intriguing and unexpected outcome. This raises further questions about the advantages and possibilities of using AI in structural engineering.
Cross-Sectional Plane Analysis Unveiling New Insights in AI Structural Design - Human-AI Collaboration Enhances Structural Optimization
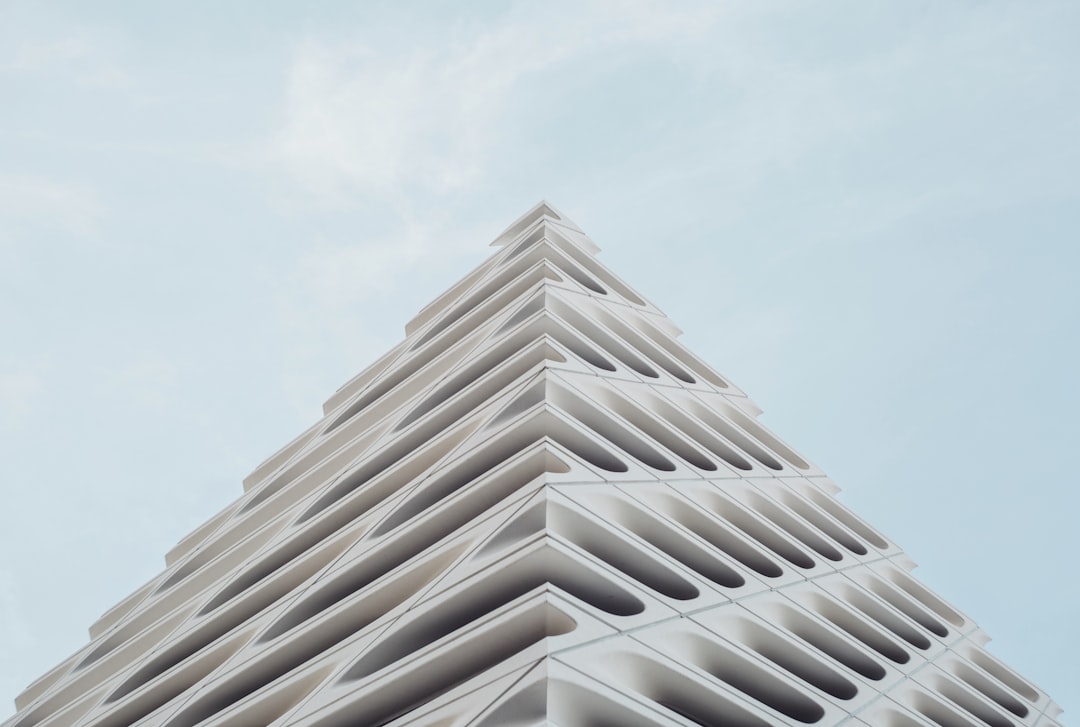
The integration of human expertise with AI in structural optimization is revolutionizing the design process. By combining human intuition and AI's computational power, engineers can tackle complex structural challenges more effectively, leading to innovative and optimized designs. This collaboration enables iterative refinement of structural components, allowing for the development of structures that are not only mechanically sound but also aesthetically appealing.
AI's ability to rapidly generate and evaluate design options accelerates the optimization process, which is particularly useful for tasks like optimizing beam designs in buildings or bridges. However, the human designer remains central to this process, providing critical feedback and ensuring the generated designs meet the specific needs of the project. This feedback loop helps refine the AI's understanding and leads to continuously improved design methodologies.
Transparency in how humans and AI interact is paramount. This fosters a deeper understanding of the decision-making process, promoting trust and ensuring that the collaborative system delivers desired outcomes. As AI capabilities continue to expand, the future of human-AI collaboration in structural engineering seems promising, with the potential to further reshape traditional practices and push the boundaries of design and construction.
Human-AI collaboration in structural optimization is demonstrating a potential to surpass the creative limits of human designers alone. By combining human intuition with AI's computational prowess, we can explore innovative solutions for complex structural needs. This pairing allows engineers to tap into AI's ability to rapidly analyze vast datasets, giving them access to a previously unimaginable scale of design information. They can leverage historical design data along with immediate feedback loops, leading to more informed decisions rooted in lessons learned from past projects.
However, the integration of AI doesn't eliminate the need for human input. For example, while AI tools like StructGANRule can produce highly optimized designs, aesthetic considerations still rely on human judgment. This highlights the critical role human oversight plays, especially when balancing form and function in a structural design. Furthermore, the collaborative design process can help to mitigate existing biases in traditional design practices. By using AI algorithms that systematically assess design options based on performance, we may see more equitable and effective designs across diverse applications.
One intriguing aspect of AI's influence on structural design is the possibility of crowd-sourced data integration. This means that designs could potentially benefit from the feedback and performance evaluations of a broader community of engineers, potentially enhancing the resilience of designs against unforeseen challenges such as natural disasters. The collaborative workflow also enables engineers to identify and correct design flaws during the rapid iteration process. This leads to shorter feedback cycles and subsequently faster project completion times, allowing for the exploration of a wider range of potential designs.
It's interesting to note that AI integration doesn't just enhance efficiency; it also seems to encourage a more critical examination of design safety factors. AI's methodical evaluation capabilities potentially shift the reliance from traditional design heuristics to more data-driven safety standards. AI can perform stress testing under a wide range of conditions, uncovering previously hidden performance limitations. This capability unlocks new possibilities for experimenting with geometries that were previously considered unsafe or impractical.
However, we must recognize the uniquely human aspect of structural design—the emotional connection and storytelling woven into a structure's visual form. While AI can certainly generate optimized shapes, it's the human element that defines a structure's purpose and impact within a specific context. Finally, the changing landscape of structural engineering has brought about new training methods. Engineers are now exposed to a hybrid approach—combining traditional methodologies with AI tools. This educational shift should help create a new generation of engineers who are well-equipped for a future where human and AI work together to craft innovative and effective structures.
Cross-Sectional Plane Analysis Unveiling New Insights in AI Structural Design - Machine Learning Refines Actionable Structural Analysis
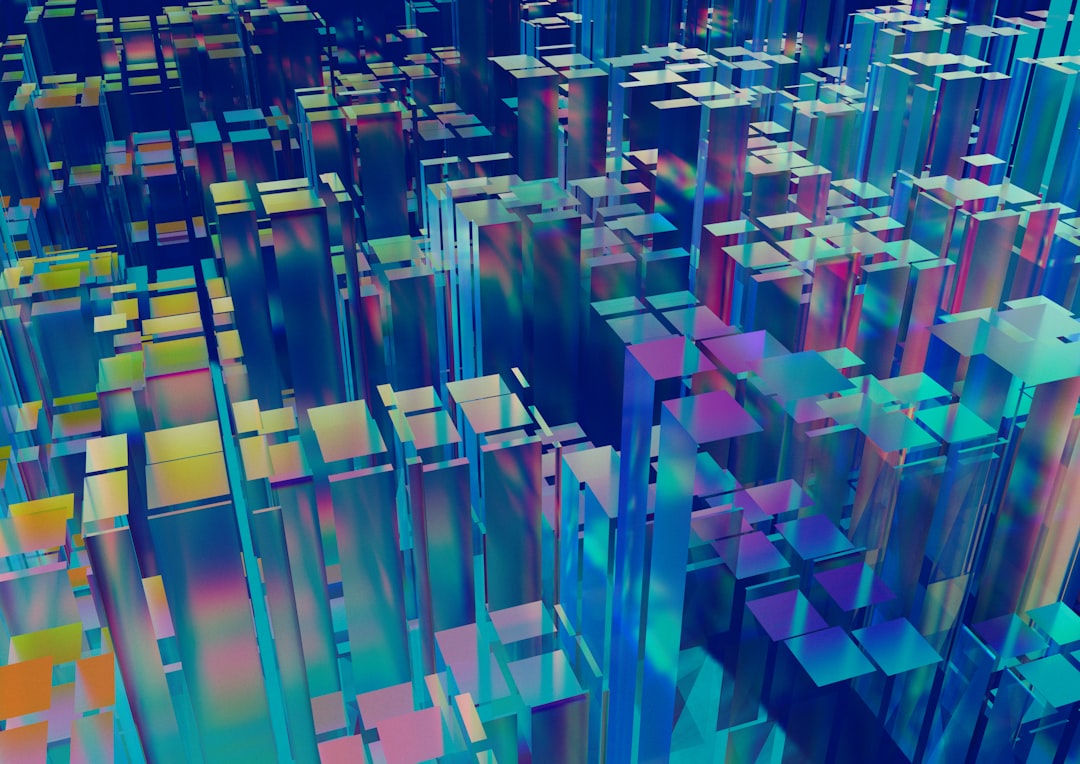
Machine learning is increasingly being used to refine structural analysis, making it more powerful and actionable in the design process. The ability to assess uncertainty and adapt analysis strategies, seen in methods like AUQmeta, is improving the reliability of structural designs. This isn't just limited to reliability; machine learning is being applied to various challenges in structural engineering. For instance, it's finding use in damage detection, predicting future structural behavior using time series data, and enhancing model updates, making design processes more efficient.
Deep learning models like graph convolutional networks and Bayesian neural networks are also proving beneficial. They offer new ways to analyze very large and complex structures and improve architectural design processes. AI-driven techniques accelerate the design process, which is positive. However, this also compels a critical look at current standards and design assumptions, potentially pushing boundaries of what's considered feasible in structural design. While promising, the integration of machine learning also introduces a need to carefully consider the roles of automated systems and the experienced human engineers who ensure that designs are not just efficient, but safe and aesthetically pleasing.
Machine learning's application in structural analysis is becoming increasingly sophisticated, offering new pathways for refining the design process. However, these methods are profoundly reliant on the quality and quantity of data used to train the underlying models. If the training data is limited or skewed, the resulting design recommendations could be unreliable.
Conversely, a significant advantage is the potential for leveraging historical data. Machine learning can identify patterns in past structural performance, unearthing common failure modes that traditional design methods might overlook. This data-driven approach can foster more resilient structures based on empirical evidence rather than just assumptions.
One intriguing area of investigation is the potential for enhanced variability in machine-learned designs compared to those created by humans. While this greater flexibility could inspire innovative solutions, engineers must rigorously evaluate these designs to ensure compliance with safety standards.
The introduction of real-time feedback within machine learning tools allows for a more dynamic design process. Engineers can directly adjust parameters and see the immediate consequences, fostering faster iteration cycles than with traditional approaches. This can be extremely valuable for tasks such as cross-sectional optimization where multiple stress factors need to be considered simultaneously. Algorithms are becoming increasingly capable of identifying optimized geometries that use minimal material while maintaining integrity – something that is often very difficult to achieve using standard design practices. Interestingly, aesthetics can now be incorporated into this process as well, allowing for a design focus that goes beyond pure mechanical functionality and into more visually harmonious outcomes.
However, there is also a crucial need to acknowledge the potential for algorithmic bias. If the training data contains biases, these are likely to be perpetuated by the model. Engineers need to actively identify and mitigate these issues to prevent the creation of designs that may be inefficient or overlook important considerations such as inclusivity.
The complexity inherent in many structural engineering challenges is well-suited for machine learning's capabilities. Algorithms can assess numerous variables simultaneously, well beyond the limits of human comprehension, ultimately leading to highly optimized designs. Additionally, simulations can employ a wide range of stress tests, unveiling design weaknesses that might otherwise go unnoticed. This leads to a much deeper understanding of how a structure will react under various conditions.
The adaptability of these machine learning models is also a critical advantage. As building codes evolve, and as our understanding of environmental conditions changes, these models can readily integrate new information and regulations into future design iterations. This makes machine learning an appealing tool in the constantly evolving field of structural engineering. While there are questions about the validity and reliability, it's a domain of growing interest, and it is exciting to explore how these technologies could change the future of structure creation and evaluation.
Cross-Sectional Plane Analysis Unveiling New Insights in AI Structural Design - AI Bridges Gap Between Architecture and Structural Engineering
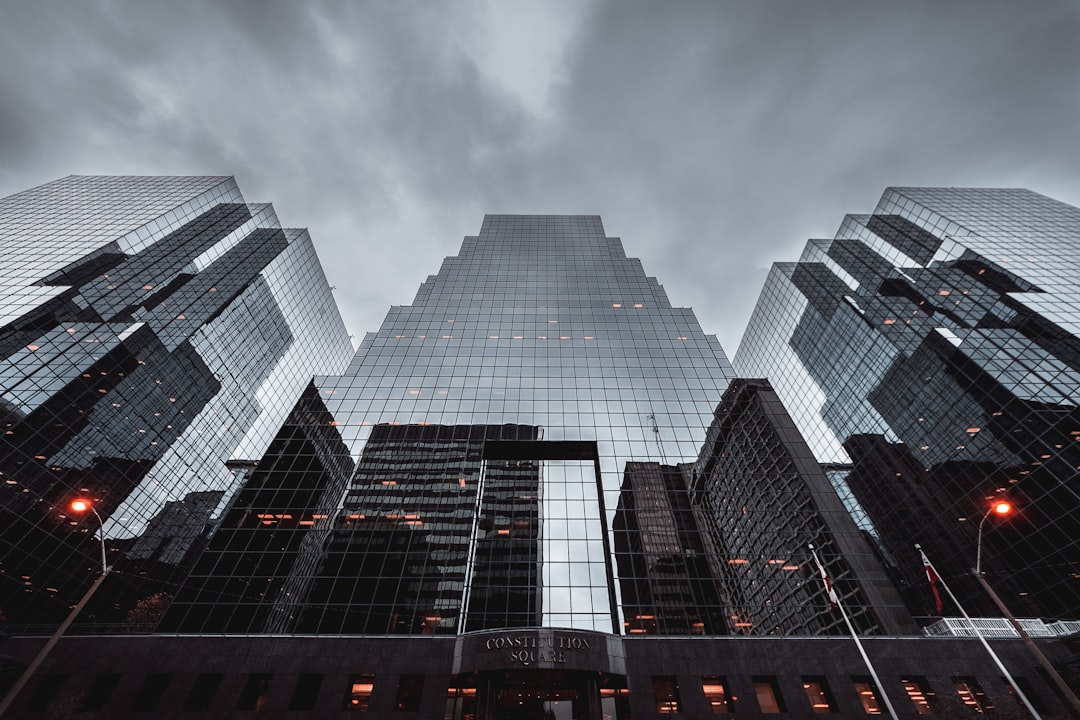
Artificial intelligence is progressively narrowing the divide between architectural design and structural engineering by promoting a more unified design approach. Historically, these fields have often operated somewhat independently, but the emergence of AI tools is facilitating enhanced collaboration between architects and engineers, streamlining the design process. AI's capacity to optimize structural designs using machine learning provides an opportunity to refine both the practical performance and the aesthetic appeal of structures. However, it's crucial to remember that while AI tools accelerate the generation and evaluation of design options, the fundamental role of human expertise in decision-making and creative expression is not diminished. Architects and engineers still play a vital role in ensuring that the designs produced through AI align with safety codes and the unique artistic vision of a project. As AI continues to mature, its potential to reshape the relationship and practices within these disciplines becomes more pronounced, leading to a reconsideration of long-held assumptions and traditions within architectural and structural engineering.
AI is increasingly utilizing historical data from completed structures to recognize patterns and understand failure modes that traditional design methods might miss. This allows structural engineers to ground their decisions in empirical evidence, ultimately leading to stronger predictive power in design.
The incorporation of machine learning has also enabled real-time adaptation in design algorithms. Engineers can now modify design parameters and instantly see the results, significantly speeding up the iterative process. This real-time feedback loop allows for a more comprehensive exploration of complex structural challenges, particularly when evaluating structures under various stress scenarios.
AI tools like StructGANRule have opened up a significantly larger design space for engineers. This rapid exploration of multiple configurations can uncover unique design solutions that might be overlooked by human intuition, potentially leading to innovative breakthroughs in structural engineering.
Furthermore, recent developments allow for a more holistic approach to design by integrating both structural performance and aesthetic considerations. This integrated methodology bridges the gap between engineering and the artistic aspects of architecture, encouraging the development of designs that are not only functional but also visually appealing.
One intriguing aspect is the potential for AI to tailor designs to localized requirements. Machine learning algorithms can adapt to specific environmental constraints, leading to more relevant and effective structural solutions within diverse geographic and regulatory frameworks.
Early research suggests AI-enhanced designs could lead to more efficient material use compared to traditional methods. This not only offers economic benefits but also encourages engineers to rethink the boundaries of what's achievable in structural integrity.
However, we need to remain mindful of algorithmic bias. If training data contains biased assumptions, those biases might be replicated in the design outcomes. Engineers must carefully analyze and refine training data to ensure equitable and inclusive design solutions.
AI's ability to explore unusual geometries is a notable benefit. While this can offer exciting opportunities for innovation, rigorous safety assessments must accompany the exploration of unconventional structural configurations.
The capacity of machine learning systems to analyze a vast number of variables and complex interactions makes them remarkably effective in handling complex design problems. This ultimately results in designs that adhere to safety standards while potentially exceeding previously defined limits of what's feasible.
The changing landscape of structural engineering is prompting new educational approaches. We're witnessing the emergence of a hybrid engineering practice that combines conventional knowledge with AI-driven tools. This transition is creating a new generation of engineers equipped to handle a future where human ingenuity and computational power are intertwined in design.
Cross-Sectional Plane Analysis Unveiling New Insights in AI Structural Design - Deep Learning Optimizes Cross-Sectional Design Schemes
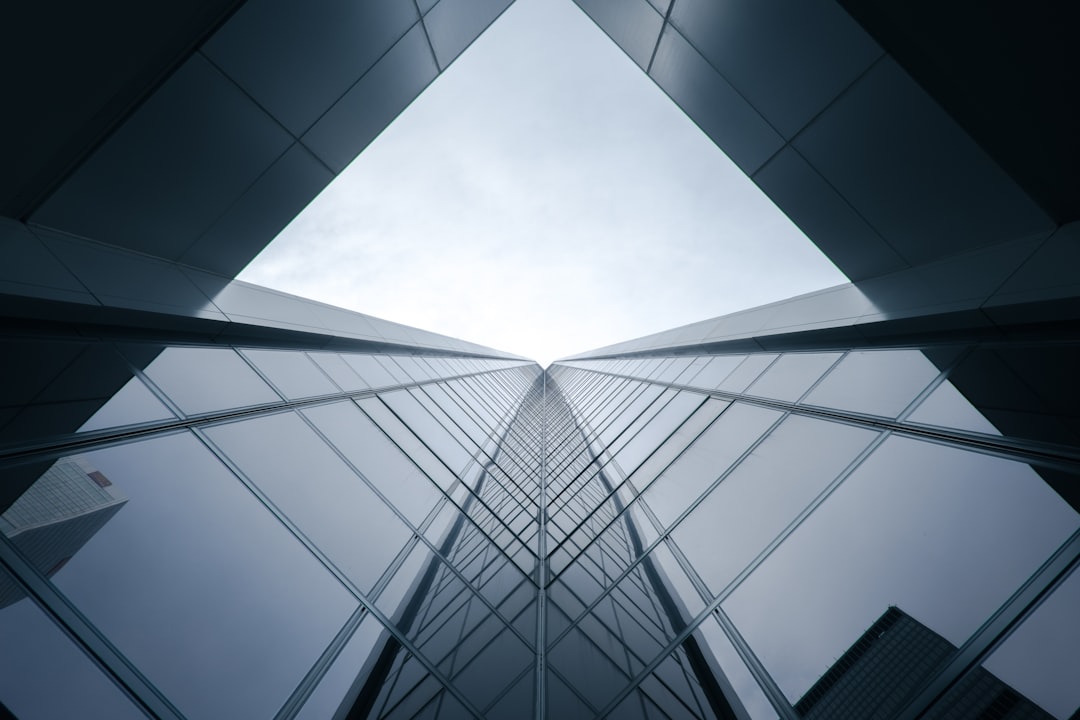
Deep learning is revolutionizing cross-sectional design by streamlining the creation and evaluation of structural components. The integration of deep learning algorithms with generative design allows engineers to automate the development of complex shapes, while factoring in critical elements like load distribution and structural resilience. This automated approach not only speeds up the process of generating design possibilities but also allows for a more comprehensive evaluation of engineering performance across a variety of criteria. Some novel graph-based reinforcement learning techniques are proving more efficient and deliver higher quality designs than older optimization methods. This technological shift brings about important conversations about the role of human intuition in design, as well as the careful consideration of aesthetics and other non-technical considerations. The challenge is to create a process that embraces innovation while ensuring structural safety remains a paramount concern.
Deep learning's ability to sift through massive datasets offers a powerful new lens for understanding the intricacies of structural design. It can uncover hidden relationships and patterns that traditional methods often miss, leading to more nuanced design insights. These deep learning models are not static; they can adapt and learn as new data becomes available, making them more resilient to obsolescence compared to fixed design rules. Interestingly, deep learning can facilitate a holistic approach to design, seamlessly weaving together architectural aesthetics and the stringent demands of structural integrity within its optimization process. This fosters a more unified design perspective, bridging a historical divide between these two disciplines.
One of the most exciting aspects is the potential for highly detailed and accurate stress testing within these systems. Engineers can simulate a wide array of stress scenarios in real-time, providing a level of design assurance that was previously unavailable. This has the potential to identify design flaws much earlier in the process, potentially eliminating the need for expensive and time-consuming physical testing. Moreover, deep learning can push the boundaries of design by exploring unconventional geometric configurations, generating designs that challenge conventional engineering wisdom. We're talking about designs that may have once been deemed impractical.
Deep learning frameworks allow engineers to interactively adjust design parameters on the fly. This real-time feedback loop gives immediate visual feedback on the implications of those changes, dramatically improving the iterative design process. Also, these algorithms can intelligently utilize historical project data, unearthing common failure points in previous structures. This data-driven approach allows for a deeper understanding of how structures fail, leading to designs that are potentially more resilient against various environmental or operational stresses.
Deep learning methods can streamline the design and optimization phases, resulting in significant time savings. Some algorithms can churn through thousands of design iterations in a fraction of the time it would take using more traditional approaches. Early analyses suggest that deep learning approaches might optimize material use more efficiently compared to traditional design methods, potentially yielding considerable savings in material costs and resource utilization. This systematic evaluation of design possibilities based on performance metrics also offers the possibility of lessening the impact of biases found in traditional design practices. Hopefully, we can see more equitable and inclusive design outcomes across different structural applications.
While there are many open questions about the adoption of deep learning in structural design, it is definitely an area worth continued investigation. There is real potential to shape a new era of structural engineering where AI and human expertise work together to realize innovative and safe designs. It's a fascinating intersection of computer science and structural engineering.
Cross-Sectional Plane Analysis Unveiling New Insights in AI Structural Design - AI-Assisted Methods Surpass Traditional FEA Tools
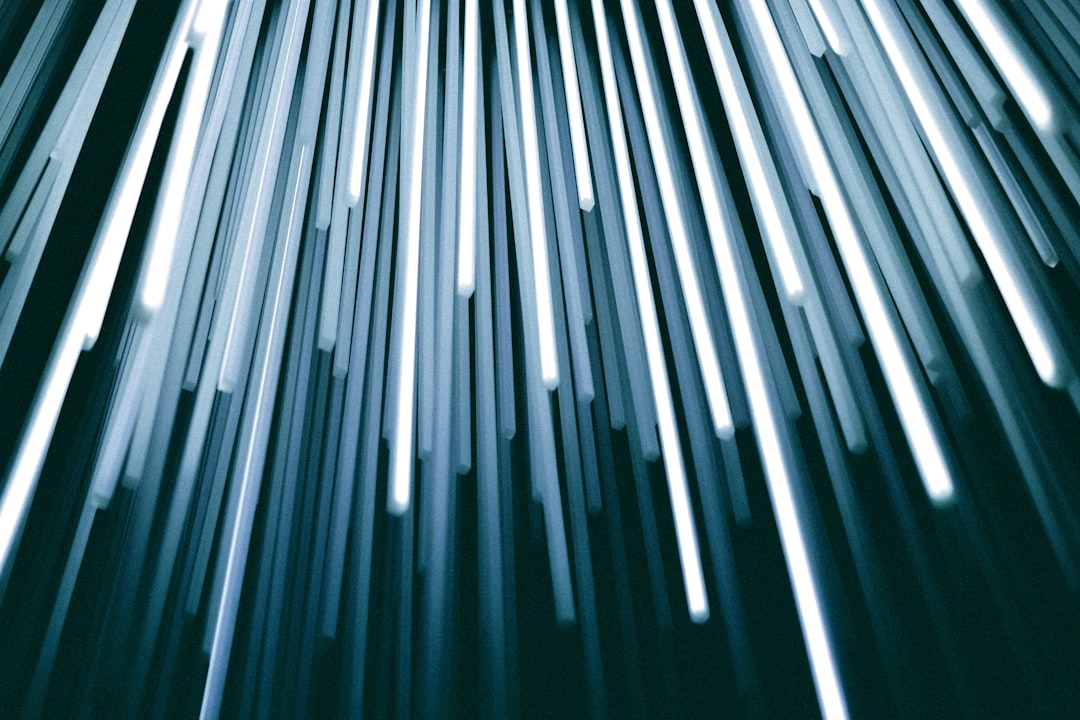
AI-powered methods are rapidly outperforming conventional Finite Element Analysis (FEA) tools, particularly in analyzing structural cross-sections. Deep learning's integration into computational analysis showcases the potential to streamline structural design processes, addressing weaknesses found in traditional approaches. This advancement hinges on access to robust datasets encompassing simulation results, real-world performance data, and sensor information relevant to the structures being designed. While FEA has a long history and broad applicability, the rise of AI-driven methods has led to substantial improvements in evaluating structural performance, extending beyond purely mechanical aspects to encompass aesthetics and other design goals. Despite their promise, a critical eye is needed when using these sophisticated tools. Human expertise and careful consideration of potential biases in the AI models are crucial to ensure design safety and regulatory adherence remain paramount.
AI-driven methods are increasingly outperforming conventional Finite Element Analysis (FEA) tools, especially when delving into cross-sectional plane analysis within structural design. The integration of deep learning within computational analysis offers the potential to significantly streamline and refine the structural engineering process, surpassing the limitations inherent in more traditional techniques.
This shift towards AI-powered FEA starts with the need for high-quality data—including simulations, real-world performance data, and relevant sensor data. A recent study showcased an end-to-end deep learning framework designed for structural analysis which demonstrated improved performance compared to classic FEA approaches. It's noteworthy that FEA itself has advanced significantly over the last 80 years, but AI now presents an alternative path for optimization and innovation. For example, the design of metamaterials (like auxetic and isotropic materials) is now influenced by FEA advancements coupled with AI tools. Further, AI's ability to interface with CAD tools enables the automatic generation and evaluation of 3D designs during the initial conceptual design phase of projects.
Research suggests that combining human insights with AI yields the most effective design optimizations—more so than either system operating in isolation. AI techniques offer advancements in structural engineering by providing alternatives to established modeling methods and improving design capabilities. Current discussions center on a two-way deep learning framework for real-time calculations in structural design. A major focus is balancing the use of regularization methods with maintaining high computational efficiency.
While this is an exciting trend, we must acknowledge that the quality of data fed into these algorithms is crucial. If the training data is skewed or contains biases, this can lead to undesirable outcomes in the final design. There is a need to develop strategies that account for potential biases and develop methods that make the optimization process transparent.
Furthermore, the development of these methods necessitates a change in how we train engineers. A hybrid educational approach that combines traditional techniques with new AI-driven tools seems essential for creating a new generation of engineers who can thrive in an environment where AI and human intuition interact seamlessly. Ultimately, the potential for AI to reshape the field of structural engineering is undeniable, even if the full ramifications and practical limitations of these approaches are still being explored.
Revolutionize structural engineering with AI-powered analysis and design. Transform blueprints into intelligent solutions in minutes. (Get started for free)
More Posts from aistructuralreview.com: