AI-Assisted Structural Inspections The Future of Certified Code Enforcement Officers
AI-Assisted Structural Inspections The Future of Certified Code Enforcement Officers - AI Integration in OSHA Safety Training for Structural Inspections
The incorporation of AI into OSHA safety training for structural inspections signifies a pivotal shift in how construction safety is approached. This integration is driven by the need for more effective risk management and compliance with safety protocols. AI tools like computer vision and predictive analytics are being leveraged to achieve these aims. Beyond just efficiency gains, the primary goal is to address the industry's persistent problem of high injury and fatality rates. The development of technologies such as exoskeletons and drones for hazard identification is changing the landscape of safety training, pushing towards a more anticipatory model for worker safety. While the potential benefits are clear, it's crucial to continually evaluate how these AI tools are implemented and their overall efficacy in achieving a truly safer work environment. This ongoing critical analysis is vital as AI's role in construction safety evolves.
The integration of AI into OSHA safety training for structural inspections is an interesting area of development. AI algorithms can analyze a massive amount of historical inspection data, uncovering patterns and anomalies that might escape human observation, which can help refine safety assessments. The ability of AI to predict structural failures with increased accuracy compared to traditional methods could be a game-changer for proactive maintenance strategies. This could potentially lead to significant cost savings in construction projects, and a notable reduction in risks to worker safety.
Integrating AI into OSHA training programs could transform how inspectors learn. Scenario-based simulations that recreate real-world situations are now possible, providing a safe environment for inspectors to hone their responses to hazardous situations without physical risk. AI can significantly reduce the time it takes for new inspectors to achieve proficiency in safety practices compared to traditional methods. This can also apply to older buildings where AI can assess the structural integrity against historical records, aiding compliance with modern safety standards during inspections.
Furthermore, AI-powered tools can provide instant feedback during training exercises, identifying errors in real-time, allowing trainees to immediately adjust unsafe practices. This type of immediate feedback, combined with the ability of AI to analyze worker behavior, can lead to a better understanding of common safety breaches. This allows for the development of training that specifically targets weaknesses and improves the retention rate of safety protocols.
The marriage of AI and virtual reality in OSHA training is an emerging field. It provides inspectors with immersive, realistic inspection environments while providing immediate guidance. This interactive approach seems to be effective at increasing trainee engagement and comprehension of safety procedures. While the potential benefits of these advancements are substantial, it's also vital to acknowledge the inherent challenges in incorporating AI into training. Ensuring the reliability and fairness of AI-driven feedback mechanisms and avoiding potential biases are critical areas that need to be carefully considered. However, the potential of AI to enhance structural inspection safety is undeniable and it will be crucial to observe how it continues to transform the field.
AI-Assisted Structural Inspections The Future of Certified Code Enforcement Officers - Data-Driven Decision Making for Code Enforcement Officers
Data-driven decision-making is becoming increasingly important for code enforcement officers as they face the growing challenges of ensuring compliance with regulations. By utilizing historical data and AI algorithms, code enforcement officers can uncover patterns and anticipate potential violations more effectively. This can lead to a more proactive approach to enforcement, reducing the time needed to identify and address safety hazards. However, the increasing use of AI in this context raises important questions about transparency. It is crucial to understand how AI algorithms arrive at decisions and the underlying data they use, ensuring fairness and accountability. There's also the potential for bias in these systems, which needs careful monitoring and mitigation. Moving forward, successfully implementing data-driven approaches will necessitate a delicate balance between using the power of data and AI to enhance decision-making and addressing the ethical implications to build and maintain public trust. Additionally, proactive outreach and education programs can play a vital role in fostering a culture of compliance and building stronger relationships between code enforcement and the communities they serve, ultimately contributing to a safer environment for everyone.
AI's ability to analyze vast amounts of data is transforming how code enforcement officers operate. By training AI models on historical inspection data, we can potentially identify recurring patterns and predict future violations, which is valuable for resource allocation. For instance, studies suggest that a significant portion of repeated code violations arise from predictable patterns, enabling more targeted enforcement strategies.
Data-driven approaches can also improve the accuracy of structural assessments. While the field is still evolving, early studies hint at a significant increase in identifying potential safety issues compared to traditional methods. This could potentially lead to a reduction in both injuries and structural failures.
The use of machine learning to analyze visual inspection data like videos and photos is another exciting avenue. AI algorithms can be trained to automatically detect code violations, though the accuracy rates are still being optimized. However, the potential for increased efficiency in compliance monitoring is substantial, potentially freeing up officers for more complex tasks.
Looking beyond individual inspections, the use of data analytics can streamline code enforcement operations. This could involve optimizing permitting processes and reducing project delays by addressing compliance issues proactively. Geographic Information Systems (GIS) are another promising tool, enabling officers to identify areas with high violation rates, optimize inspection schedules, and potentially even reveal unexpected trends within specific neighborhoods.
Moreover, integrating data across different departments can improve communication and collaboration, fostering a more efficient and responsive code enforcement process. Interestingly, studies have also shown that agencies utilizing data-driven approaches may experience an increase in public trust, likely due to improved transparency and accountability. This trust can translate into a smoother relationship between enforcement agencies and communities.
There are clear potential benefits in terms of mitigating financial risk as well. Predictive models suggest that proactive data-driven inspections can lead to a decrease in litigation related to code violations. This potential cost saving is a powerful incentive for agencies to consider embracing data-driven techniques.
Of course, data-driven techniques need to be accompanied by appropriate training. Code enforcement officers need to understand the data and how it's being used in order to leverage it effectively. Building data analytics into training programs allows officers to develop more nuanced and effective approaches to interpretation of codes and regulations.
Furthermore, one unexpected benefit is that the consistent use of data can shed light on potential systemic issues. For example, analysis might reveal disparities in compliance rates across different neighborhoods or communities, prompting deeper investigations into the root causes of the issues. This information can be utilized to design more targeted and effective intervention strategies.
While the benefits of integrating AI into code enforcement appear promising, it's crucial to carefully consider the potential ethical implications and ensure transparency and fairness in decision-making processes. Continued research and careful implementation will be key to harnessing the true potential of data-driven methods while preserving the core values of community trust and equity.
AI-Assisted Structural Inspections The Future of Certified Code Enforcement Officers - Drone and AI Synergy in Critical Infrastructure Assessment
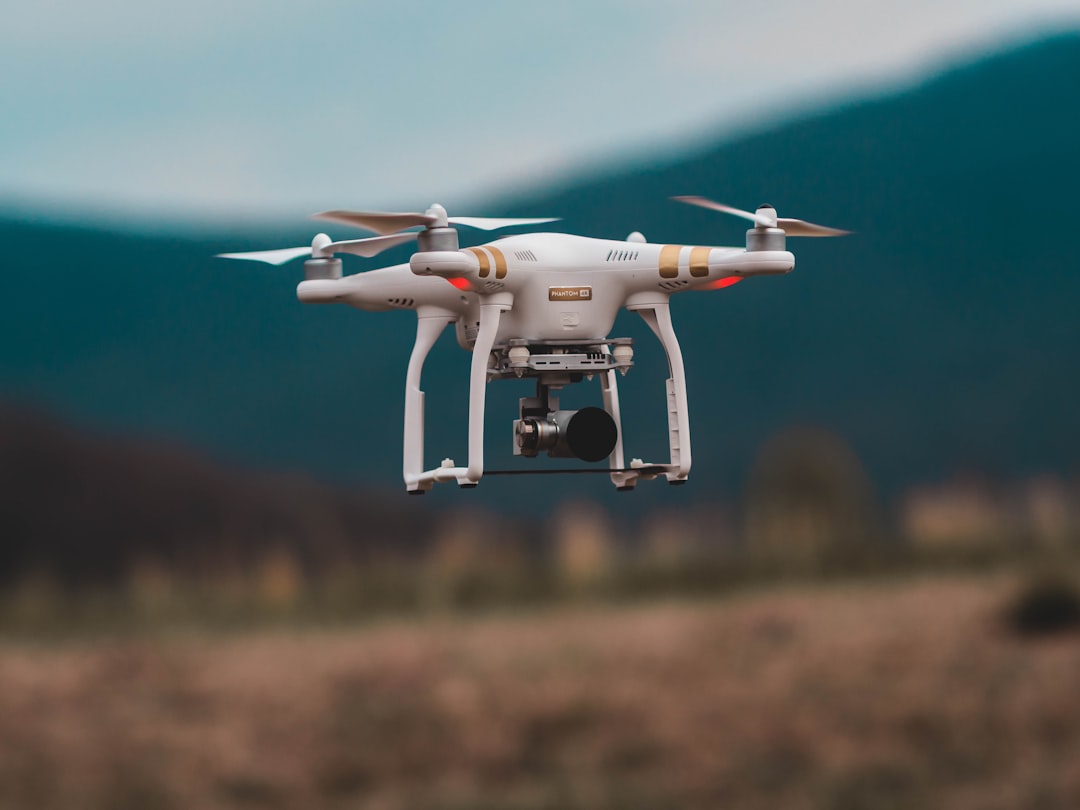
The combination of drones and artificial intelligence is transforming how we assess the condition of critical infrastructure, especially when it comes to structural inspections. As many critical structures like bridges age and show signs of wear and tear, drones equipped with AI capabilities offer a way to do comprehensive and timely inspections that can be difficult or impossible using older methods. These drone-AI systems are making it easier to identify and understand different elements of the structures, which is helping improve the quality of data used for things like Building Information Modeling (BIM) and predicting when maintenance will be needed. Despite these improvements, it's important to acknowledge the challenges associated with relying on AI, including the accuracy of the data and the potential risks of trusting automated systems too much. As certified code enforcement officers become more involved with this new technology, it's critical that they are able to evaluate drone-AI systems in a way that increases safety and effectiveness of infrastructure inspections while maintaining high ethical standards.
The synergy between drones and artificial intelligence (AI) is revolutionizing how we assess the health of critical infrastructure, especially bridges. Drones, or Unmanned Aircraft Systems (UAS), are becoming increasingly valuable for inspection tasks because of their speed and maneuverability. This is especially important given the aging infrastructure we're dealing with. A significant portion of America's bridges, many built in the postwar era with an expected lifespan of 50-100 years, are showing signs of wear and tear. Ignoring these issues can have severe consequences, as maintenance costs skyrocket the longer repairs are delayed.
The combination of drone-captured imagery and AI analysis offers a powerful approach to infrastructure inspection. It allows for rapid, automated recognition of objects within construction sites, which is pushing the development of advanced Building Information Modeling (BIM) further. Moreover, AI integration facilitates real-time analysis during inspections, enabling drones to autonomously navigate, assess damage, and even avoid collisions. This fusion of technologies represents a step change in infrastructure assessment, moving towards more thorough and reliable data collection.
In essence, drones are becoming a standard tool for analyzing structural damage in civil engineering projects. They can quickly and accurately determine the condition of infrastructure, helping us move away from less efficient traditional methods. We might even see a future where certified code enforcement officers work more closely with drones and AI to improve safety and compliance monitoring across the board.
However, research into this area is still relatively new. It's still worth pondering how these techniques may affect the way we currently do things. Further research is needed to better understand the long-term effects of employing AI and drone technologies for infrastructure assessment and whether the associated costs and potential biases are truly mitigated. Yet, given the need for reliable, affordable, and timely infrastructure inspections, it's an avenue that demands careful exploration and consideration.
AI-Assisted Structural Inspections The Future of Certified Code Enforcement Officers - Digital Twin Creation Through AI-Powered Photogrammetry
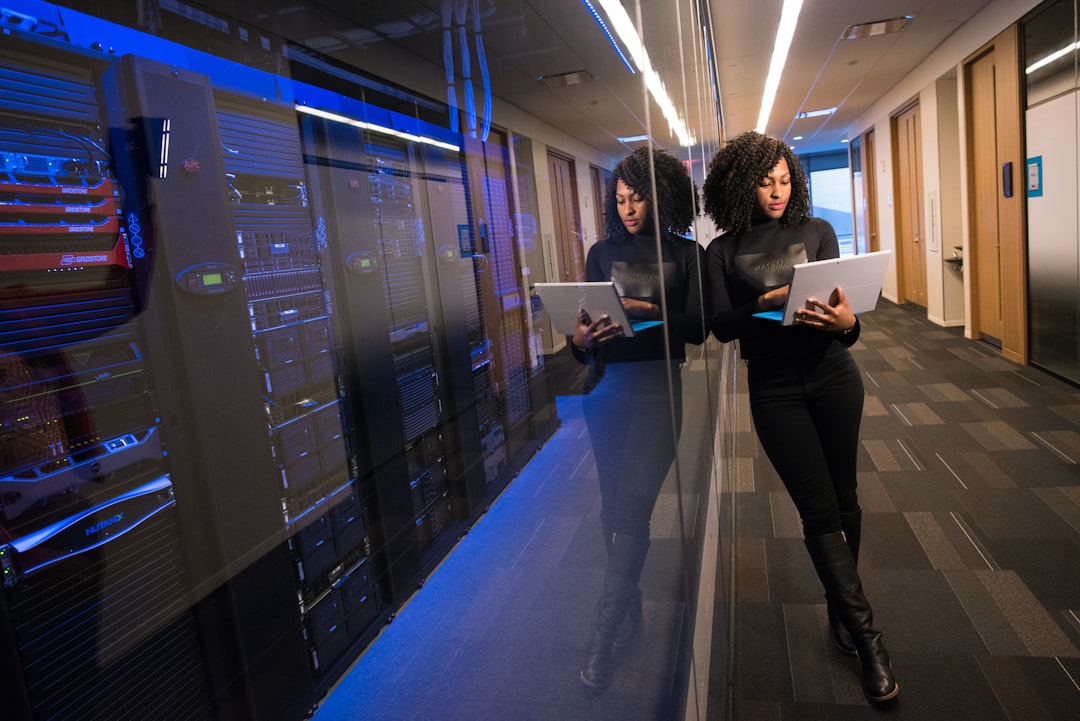
Creating digital twins using AI-enhanced photogrammetry offers a powerful new approach to understanding and managing physical structures. This method involves generating highly accurate 3D models of buildings or infrastructure using AI to process photographs. These digital twins are not static representations; they adapt over time, incorporating new data and insights about the structure's condition. This dynamic approach allows for ongoing monitoring and historical comparisons, enabling quicker identification of potential problems like deterioration or damage.
The integration of AI significantly elevates the precision and detail of these digital twins compared to traditional modeling techniques. This is especially important for complex structures where a comprehensive understanding of every component is vital. By employing AI to analyze and process data from various sources, including photographs, these digital twins can provide a far more comprehensive and detailed representation of the physical world than would be possible using just traditional 2D images or basic 3D modeling. The ability to understand structural behavior and predict potential maintenance needs through these models holds immense promise for improving the safety, longevity, and efficiency of structural inspections. While still a relatively new field, this approach to digital twin creation has the potential to revolutionize how we interact with and maintain our physical environment. There are still questions to consider about the accuracy and reliability of the models, but the initial results are promising.
Digital twins, especially those built using AI-powered photogrammetry, are becoming quite interesting in the world of structural inspections. One fascinating aspect is the level of precision they offer. We're talking about millimeter-level accuracy in recreating the physical structure virtually. This detailed representation is a significant step up from older methods and offers a much richer foundation for inspection planning and maintenance strategies.
Another intriguing characteristic is their dynamic nature. Unlike static models, AI-generated digital twins can be updated constantly as new data comes in. This creates a living snapshot of the structure's condition rather than just a static image from a certain point in time. This ongoing evolution is vital because it helps us track changes over time.
This ability to analyze the change in a structure's condition over time is also very beneficial. By comparing photos or scans captured at different times, the digital twin can reveal the progression of issues. This isn't just insightful; it can help engineers predict when maintenance may be needed based on past degradation trends.
Further, we can integrate machine learning into the process, enabling the system to detect anomalies in the digital twin that might indicate unexpected or problematic behavior. Detecting issues proactively like this has the potential to prevent failures and allows for a proactive maintenance schedule rather than responding after a problem has already arisen.
Beyond the technical details, digital twin outputs are quite user-friendly. Complex data about a structure can be visualized in 3D, making it much easier for engineers to interpret and share results with others involved in the project. This enhanced visualization is important for improving communication, facilitating better collaboration, and ultimately making the inspection process more transparent.
We can also imagine a future where digital twins are closely integrated with IoT sensor networks that are capturing data about a building's environment. This creates a broader understanding of how external factors like temperature or humidity might influence the structural integrity over time.
Interestingly, the digital twin concept extends beyond civil engineering and construction. Aerospace and manufacturing industries are beginning to experiment with this idea as well, which expands the applications far beyond the initial use cases of structural inspections.
It's also notable that digital twins could improve the effectiveness of regulatory compliance. By creating a visual, readily understandable depiction of structural data, it might become a crucial tool during audits or compliance checks by certifying agencies.
The potential to lower costs is a significant driver for the increased interest in digital twins. By enabling continuous monitoring and insightful analysis of structural integrity, digital twins can shift maintenance strategies from reactive repair to predictive maintenance, saving money in the long run.
Finally, the inherent collaborative nature of the digital twin promotes communication and information sharing among various parties. Architects, engineers, and inspectors can all access the same accurate data, creating a more unified approach to ensuring structural integrity and compliance. This level of collaboration has the potential to prevent costly miscommunication and delays, ensuring projects meet the highest standards of safety and quality.
AI-Assisted Structural Inspections The Future of Certified Code Enforcement Officers - AI-Guided Robotic Systems for Building and Bridge Inspections
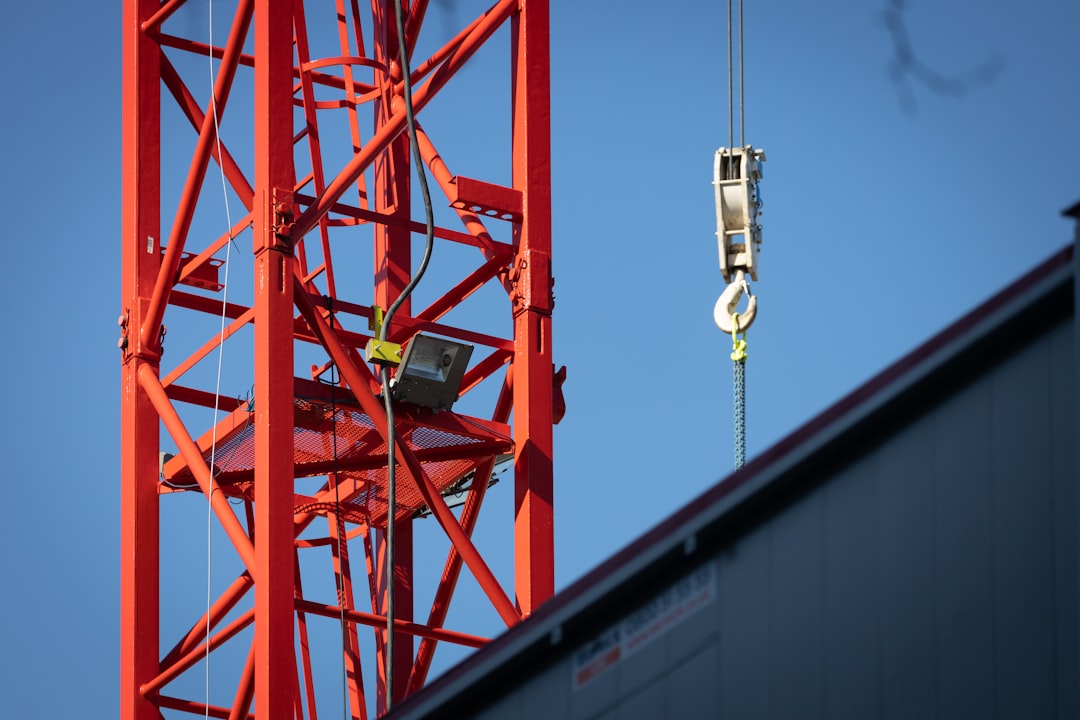
AI-guided robotic systems are revolutionizing the way we inspect buildings and bridges. These systems, equipped with advanced computer vision and machine learning, can automatically identify structural issues like cracks in concrete. This is a crucial development given the increasing number of aging structures that are prone to failure. The robots can autonomously inspect structures, particularly in areas that are hazardous or hard to reach for human inspectors, making the inspection process more efficient and safe. These robotic systems offer the advantage of more frequent inspections compared to traditional manual methods, leading to a more proactive approach to infrastructure maintenance. However, as with any emerging technology using AI, the accuracy and reliability of these systems are still under development. It's essential to carefully evaluate how these systems are used and whether they fully mitigate potential risks to ensure the highest possible standards of structural safety. Ultimately, the use of AI-guided robotic systems has the potential to significantly reshape building and bridge inspection practices by moving towards a future of more data-driven and proactive maintenance strategies.
Researchers at Drexel University have developed a system where AI guides robots to identify concrete cracks during inspections using computer vision and machine learning. This development addresses the increasing concern about structural failures in our aging infrastructure, where many structures are degrading faster than they can be maintained. The system is designed to boost the efficiency of inspecting buildings, bridges, and roads, something that's becoming crucial in our aging built environment.
The core of this system lies in deep learning algorithms that allow robots to autonomously carry out structural safety checks. It combines visual inspection techniques with advanced machine learning to systematically assess and identify structural damage. This approach aims to proactively address damage and avoid catastrophic failures. By utilizing robots for inspections, the system can access hard-to-reach or hazardous areas, leading to more comprehensive evaluations of structures.
One of the main benefits of these AI-guided systems is their ability to carry out inspections more frequently than traditional manual methods. This continuous operation allows for greater oversight of structural health. The initiative is born from the need for more sophisticated methods of infrastructure monitoring as cities continue to age. The research out of Drexel's College of Engineering highlights how AI and robotics are dramatically improving the field of infrastructure inspection.
The broader implications of implementing this technology could involve increased safety and sustainability in maintaining our built structures. While the technology shows promise, it's important to carefully consider the associated costs, and how reliable the system truly is when deployed in different contexts. This is a complex field with many variables and unknowns still to be addressed before widespread adoption, but the potential for improving how we assess infrastructure appears to be quite significant. The research in this area is ongoing, and it will be interesting to see how these systems evolve in the coming years.
AI-Assisted Structural Inspections The Future of Certified Code Enforcement Officers - Machine Learning in Structural Performance Prediction and Damage Detection
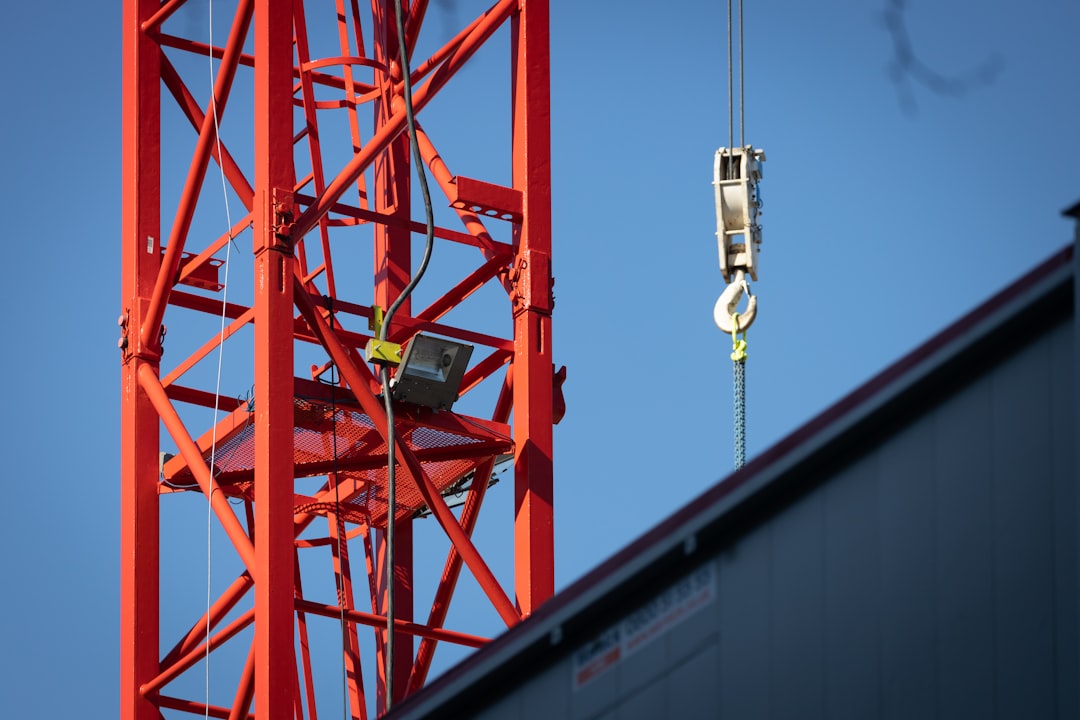
Machine learning is increasingly vital in predicting how structures will perform and identifying damage. These techniques, like deep learning and support vector machines, are helping us quickly assess the health and integrity of structures. For instance, they can use data from a limited number of sensors or images to give us real-time information on things like cracks or strain. This has the potential to transform maintenance from a reactive approach to a more proactive one, addressing issues before they become significant problems. However, as we rely more on these AI methods, we need to critically examine how reliable they really are. The accuracy of the predictions, along with how the systems respond to changes in the data over time, need careful consideration. This careful review is essential as we navigate this shift towards AI-driven structural monitoring, ensuring the reliability and safety of our infrastructure. The evolution towards AI-integrated structural assessment represents both progress and challenges for how we oversee and care for the built environment.
Machine learning techniques, like support vector machines and deep learning, are being explored for detecting structural damage. This approach holds potential for dramatically speeding up assessments compared to traditional methods that rely heavily on human inspectors. Interestingly, these AI approaches are showing the ability to predict structural issues with surprising accuracy, sometimes exceeding 90%, a significant leap over human-based estimations. This increased accuracy leads to more reliable and faster identification of potential problems in structures, allowing for potentially quicker fixes.
Integrating artificial intelligence into structural health monitoring is becoming a key area of research. This allows engineers to more rapidly and effectively assess safety and the integrity of vital infrastructure. The ability to analyze various types of data, like thermal imagery or acoustic emissions, offers a more holistic understanding of a structure's condition compared to looking at isolated data points. This multi-faceted approach potentially allows for better understanding of a structures' health overall.
The applications of machine learning in structural engineering are quite diverse. We see it used in areas like design, analysis, fire resistance, and even in the evaluation of concrete mixtures. It's fascinating to consider how AI can improve our ability to understand complex interactions within building materials. For instance, some studies show that deep neural networks can predict strain fields from limited sensor data, making it possible to identify crack damage in real-time. This area is still relatively new but offers an intriguing possibility for more efficient and continuous monitoring of structures.
Researchers are also developing image-based machine learning approaches for damage detection. This is essentially creating a kind of AI-powered visual inspection tool. This is important because it can enhance the ability to find defects, potentially leading to better, more consistent inspections. This area is also exploring the idea that AI may help automate structural maintenance, particularly addressing limitations in current human inspections.
However, as with any AI system, there are areas needing careful attention. The stability of the data over time is one important concern. Researchers are specifically looking at the "data drift" issue, where machine learning models can become less accurate if the type of data changes over time. This can be particularly problematic for classifying bridge damage states as the bridge ages and undergoes various types of wear and tear. This is a concern for any model used for assessing infrastructure conditions.
To improve model performance, methods like principal component analysis (PCA)-based anomaly detection are being researched. The way different AI models are set up, their hyperparameters, is also important to get the best possible results. This kind of fine-tuning is also crucial for improving how well machine learning models work in assessing structural health.
Ultimately, it's still too early to know the full impact these techniques will have on the roles of certified code enforcement officers. However, the improvements in AI-assisted technology will likely impact the nature of their tasks and future responsibilities. The goal is to improve accuracy, efficiency, and reliability in structural inspections, so the way inspections are done is likely to change. There is also the potential that AI will lead to new methods and expectations related to building safety and compliance that code enforcement officers will need to be trained in. While it remains an open question, it's apparent that these technologies are evolving quickly and code enforcement officers are likely to see a shift in the kinds of inspections and monitoring they do.
The development of AI-assisted inspections is aimed at enhancing the monitoring of infrastructure, striving for greater efficiency, accuracy, and reliability. It's an interesting area with lots of potential, but we also have to be cautious about relying too much on automated systems without fully understanding their strengths and weaknesses. Careful monitoring and validation will be crucial for ensuring that AI technologies are used in a way that makes our built environment safer.
More Posts from aistructuralreview.com: