Revolutionize structural engineering with AI-powered analysis and design. Transform blueprints into intelligent solutions in minutes. (Get started for free)
New Advancements in Additive Manufacturing Software A 2024 Analysis of Simulation and Workflow Integration
New Advancements in Additive Manufacturing Software A 2024 Analysis of Simulation and Workflow Integration - AI-Driven Optimization in Additive Manufacturing Software
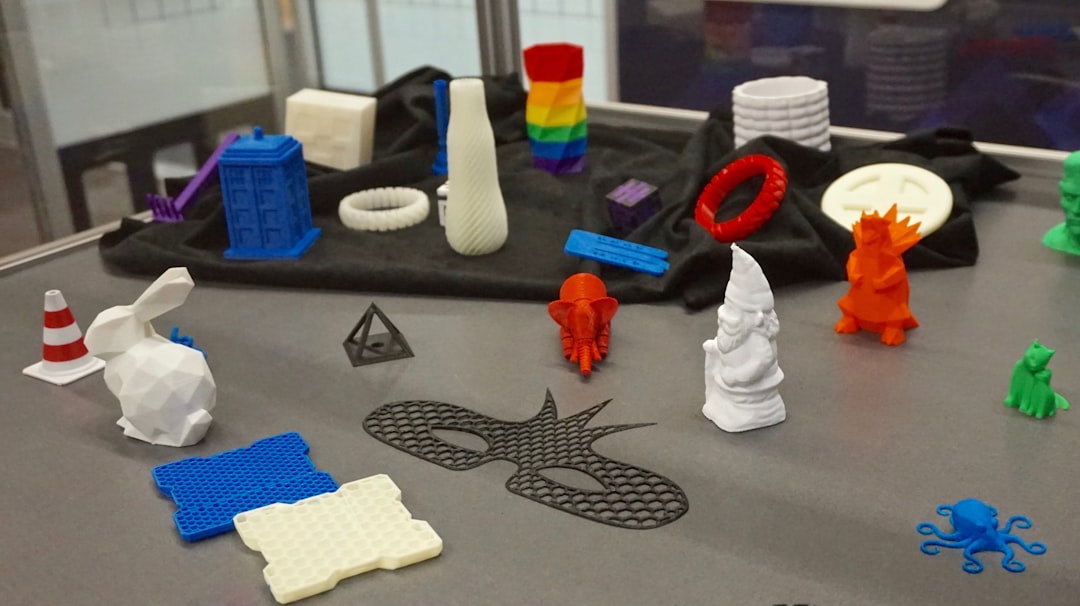
AI is increasingly influencing how additive manufacturing (AM) operates, especially when producing customized parts in smaller batches. By incorporating AI, manufacturers can precisely adjust the printing process to achieve higher-quality products. However, this advanced capability necessitates skilled designers and operators who can effectively manage the intricate aspects of AI-driven AM. The field of AI algorithms for AM is a dynamic one, with current research hinting at significant future advancements that could streamline and improve the manufacturing process. We are seeing the impact of these AI-driven improvements in various sectors, including healthcare, aerospace, and construction, showcasing the wide range of potential for AM technologies. Despite the positive trends, the integration of AI in AM also brings challenges. A critical aspect to address is ensuring the technology's development prioritizes human needs, and there's a clear need to invest in training a workforce prepared to utilize these sophisticated tools to their full potential.
AI is increasingly being integrated into additive manufacturing software, enabling optimization in ways previously unimaginable. Sophisticated AI algorithms can rapidly explore countless design variations, significantly accelerating the optimization process, especially for intricately shaped parts. This speed is a substantial improvement over traditional methods relying solely on human engineers.
Furthermore, the integration of AI allows for real-time adaptability during the printing process. Sensors embedded in the printing system feed data to the AI, which can then adjust printing parameters on the fly, resulting in higher quality final products. The ability of AI to anticipate potential failure modes in printed parts based on learned patterns from historical data is a noteworthy advancement. This predictive capability can help identify weaknesses before the part is printed, saving both time and materials.
AI-driven generative design has the potential to revolutionize part design. By exploring an expansive design space, the software can arrive at structures that are both lighter and stronger than traditional designs, often in ways a human engineer may not intuitively discover due to design complexity. This is not limited to material distribution; AI algorithms can also optimize the print process itself, including factors like print speed and layer height. This translates into significant improvements in both cost and production time.
Beyond design, AI can enhance the predictability of additive manufacturing processes. Advanced simulations driven by AI are able to forecast thermal profiles and stress distribution during the print, leading to better predictions of potential distortion or defects in the final product. This data, in turn, informs the development of closed-loop control systems that can dynamically adjust printing conditions to maintain quality.
This cycle of data-driven improvement is made possible by machine learning algorithms that learn from previous prints, continuously refining the design process and improving the efficiency of additive manufacturing workflows. The role of human input is also evolving within this AI-centric environment. While some optimization aspects are automated, engineers are still vital in providing strategic oversight, including incorporating user feedback into the design loop. This highlights a critical trend – the evolving role of engineers in additive manufacturing, moving from solely creating designs to a more strategic, oversight-driven approach that leverages the strengths of AI. However, for this vision to truly be realized, the skilled workforce needed to leverage AI effectively in AM must be developed.
New Advancements in Additive Manufacturing Software A 2024 Analysis of Simulation and Workflow Integration - Integration of Real-Time Process Monitoring Tools
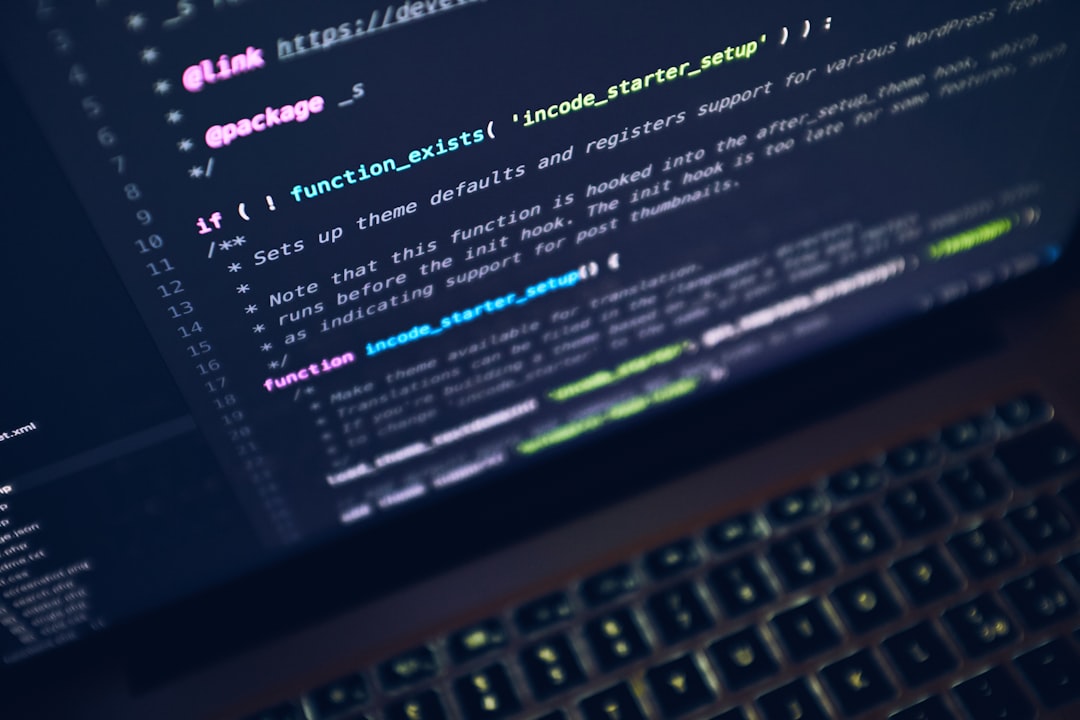
The incorporation of real-time process monitoring tools is increasingly vital in additive manufacturing, primarily aimed at enhancing both efficiency and ensuring high-quality output. Software capable of monitoring the process as it unfolds offers instantaneous feedback, letting manufacturers monitor production progress and review historical process data via user-friendly dashboards. Furthermore, the concept of digital twin ecosystems for additive manufacturing is gaining traction, paving the way for advanced remote management and the capacity for more sophisticated monitoring and testing. The expanding adoption of these monitoring approaches tackles a central issue in industrial additive manufacturing—uncertainty in part quality during production. This enhances the reliability and functionality of parts produced, fulfilling the rising demand for stringent quality controls. However, despite the demonstrable progress in this area, various obstacles persist. Ongoing work is required to develop and refine data standards for a more unified and seamless industry landscape, making the integration of these monitoring tools more universally applicable.
The integration of real-time process monitoring tools within additive manufacturing is gaining traction, particularly as a method to address the inherent challenges of this technology. While AM offers the potential to create intricate and lightweight parts that are difficult or expensive to produce via traditional manufacturing, particularly for rapid prototyping, it also introduces uncertainties that impact production consistency and quality. For example, AddUp has developed software for their FormUp machines that delivers real-time updates, creating a history of process data displayed on an accessible dashboard. This is part of a larger movement to develop a fully digital ecosystem that promotes both the monitoring and remote management of AM processes.
The need for these tools is amplified by the inherent "qualitative uncertainty" of AM processes, a significant hurdle when aiming for industrial-scale production. Monitoring tools, such as those that measure process states and component properties during production, are necessary to create more reliable output. There's ongoing research at the National Institute of Standards and Technology (NIST) into standard ways of representing and storing data related to AM, including modelling, simulations, and databases, alongside efforts to validate data integration and exchange. These efforts hopefully will enable smoother implementation of monitoring tools across different AM systems.
In-situ monitoring, however, is not simply about collecting data. The data gathered is ideally used to improve various aspects of the production process. The development of advanced monitoring techniques is integral to the overall reliability and quality of AM-produced parts. This, in turn, fuels the desire to leverage data in new ways, including using machine learning algorithms to uncover patterns and improve AM workflows. For instance, machine learning algorithms are increasingly used to enhance design and fabrication processes, providing a fresh approach to material science and structural engineering.
Furthermore, the broader trend toward automation in manufacturing has naturally been adopted by the AM industry. By integrating automated control tools, manufacturers can seek improvements in performance, output, and resource efficiency. Real-time data is increasingly important for the quality control necessary to drive productivity gains. This includes tools for digitizing data, leading to more efficient use of materials and energy.
The connection of these individual advancements towards a more complete AM workflow is crucial. The goal is seamless integration across the entire lifecycle, from design to production and even after-production analysis. We see recent advancements in AM software focused on streamlining the connections between the various stages of the AM process. It is anticipated that these advancements will lead to a more automated future, but concerns remain regarding the preparation of the workforce to oversee and maintain this technological future.
New Advancements in Additive Manufacturing Software A 2024 Analysis of Simulation and Workflow Integration - Advanced Material Simulation Capabilities for Complex Geometries
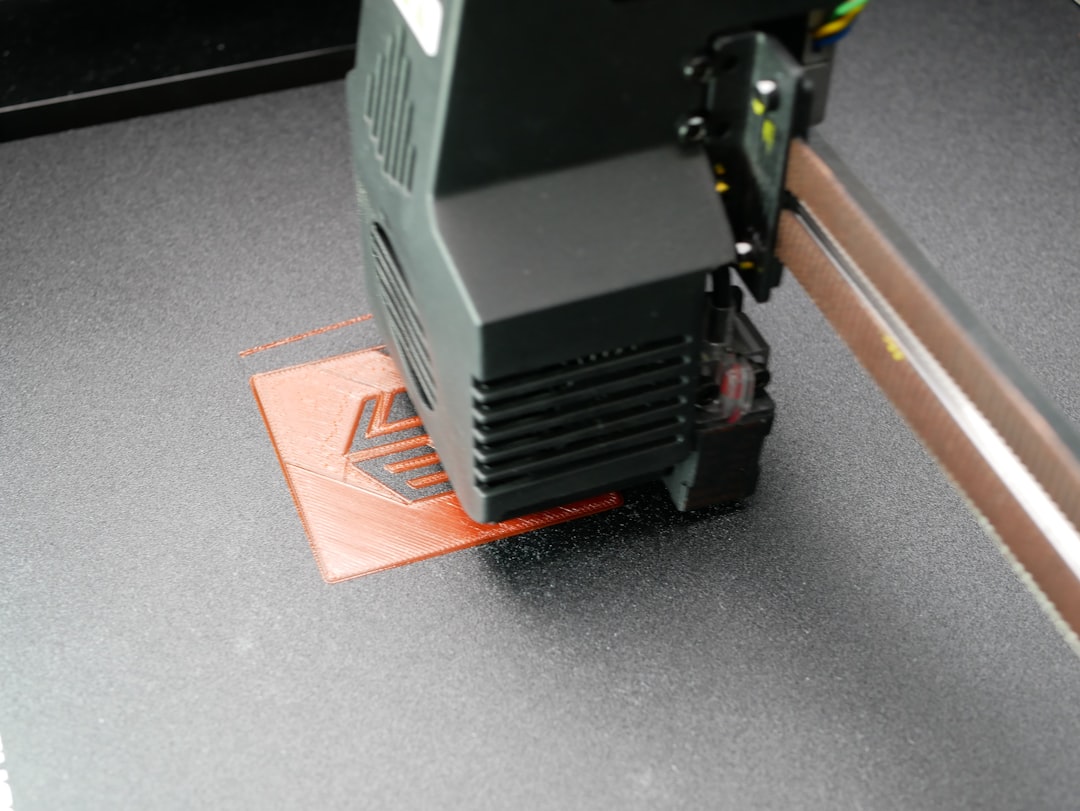
Additive manufacturing, also known as 3D printing, has opened up possibilities for creating intricate geometries that are beyond the reach of traditional manufacturing processes. However, this increased complexity brings challenges in terms of quality control and understanding how these parts will perform in real-world scenarios. Developing advanced simulation capabilities is essential to tackle these challenges, particularly for materials with complex geometries.
One major hurdle is that performing advanced simulations on complex geometries often requires substantial computing power. Simulation times can vary widely, ranging from a few hours to several weeks, highlighting a need for innovative approaches, such as improved algorithms or utilizing cloud-based computing, to make the process more efficient. On the plus side, these detailed simulations offer a wealth of information about how different materials respond under various conditions like stress and heat. This is especially important for materials like alloys and composites that can exhibit unpredictable behavior.
Simulation accuracy is significantly enhanced with dynamic mesh techniques. These techniques adjust the simulation mesh in real-time as the parts are being printed. This level of adaptability helps in achieving more accurate predictions of stress and thermal distributions—crucial for managing the complexities inherent in intricate geometries. Furthermore, we're seeing a trend towards multi-physics simulations that combine various aspects like thermal, structural, and fluid dynamics. This holistic approach is critical for a comprehensive understanding of how changes in one area affect the overall integrity and performance of the part.
There's a growing trend to integrate machine learning into the simulation process. By leveraging historical data, these machine learning-driven models can predict potential issues and refine design choices before the parts are ever physically printed. This translates into reduced prototyping, leading to less material waste and potentially faster turnaround times.
Another application of advanced simulation is in topology optimization. Topology optimization enables engineers to design components that are as strong as possible while being as light as possible. This approach frequently identifies design solutions that wouldn't be apparent through conventional methods, paving the way for more innovative support structures and optimized part design.
Different industries are beginning to adopt specialized simulation tools to meet their individual needs. For example, medical device developers may focus on simulations that predict biomechanical responses, whereas aerospace engineers would be more concerned with aerodynamic performance. These applications show the versatility and adaptability of these tools to specific use cases.
By embracing these simulation techniques, we can significantly reduce the need for physical prototyping, leading to lower research and development costs. This, in turn, allows engineers to quickly iterate through different design options, which is beneficial for keeping up with evolving market needs. Interestingly, simulations can also be utilized for predictive maintenance of additive manufacturing equipment. By modeling wear and tear under different operating conditions, we can optimize maintenance schedules and minimize unplanned downtime.
In essence, a sophisticated simulation ecosystem enables a continuous improvement loop. By incorporating real-time data and analyzing the results from the printed parts, engineers can continually refine the simulation parameters. This leads to more accurate simulation models that better represent the material behaviors and the additive manufacturing process itself. While challenges remain in terms of computational demands and algorithm refinement, the ongoing advancement of simulation tools is showing clear promise in navigating the complex world of additive manufacturing.
New Advancements in Additive Manufacturing Software A 2024 Analysis of Simulation and Workflow Integration - Streamlined Workflow Management Systems for Increased Efficiency
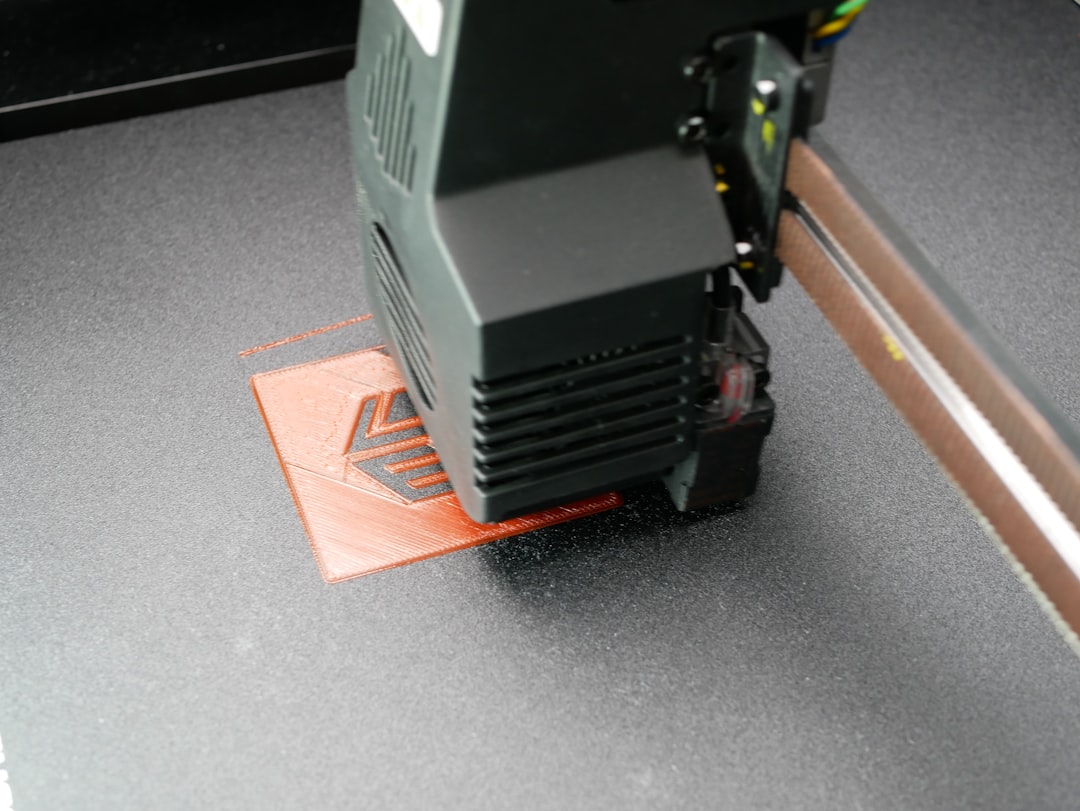
The increasing complexity of additive manufacturing (AM) in 2024 necessitates streamlined workflow management systems to optimize production and maintain efficiency. Software solutions like Fabpilot and Oqton are emerging, offering features like 3D file analysis, real-time monitoring, and integration with factory floor operations. These tools are designed to address the inherent unpredictability of the AM process, helping ensure consistent, high-quality output even as manufacturers scale up production. The promise of these systems is significant, but their complex nature requires a workforce capable of utilizing their full potential. There's a growing need for training initiatives to equip individuals with the skills necessary to manage and interpret the vast amounts of data generated by these systems. As a result, the role of engineers and designers within AM is evolving, shifting from a hands-on approach to a more strategic, oversight-oriented approach. This underlines the importance of human oversight within increasingly automated manufacturing processes. While these systems show significant promise, the challenge remains in developing and sustaining a qualified workforce to adapt and succeed in this new landscape.
The evolution of additive manufacturing (AM) software in 2024 is characterized by a growing emphasis on streamlined workflow management. While AI-driven optimization and real-time monitoring are improving individual stages of the AM process, we are now seeing systems that aim to connect those stages seamlessly. These new systems aim to provide insights across various phases, from design to production and beyond. One interesting development is the increased use of AI within workflow management. Not just for monitoring, but to analyze gathered data and offer suggestions that guide engineers towards better decisions faster. This integration also offers the possibility of reducing wasted effort due to unnecessary repeated tasks.
Another trend is the focus on real-time collaboration and integration. By bringing together information from design, production, and quality assurance onto a single platform, engineers across different departments have access to the same information. This is a step toward addressing the long-standing issue of information silos in manufacturing. This real-time aspect is helping address a key challenge in AM: managing the inherent uncertainties of the process.
One promising feature in these new workflow management systems is the integration of algorithms that predict potential production problems. By identifying failure points early on, it's hoped that manufacturers can intervene and avoid material waste, and ultimately cut down on costs. This approach of preemptively troubleshooting also connects to an increasing need for automated compliance checking. This automated monitoring helps ensure that AM processes meet industry standards and regulations, while lightening the load on engineers who are already juggling a plethora of tasks.
Visual representation of data has also seen improvements. Customized dashboards give engineers a clear view of performance indicators in real-time, allowing them to identify inefficiencies promptly and address them. The introduction of simulation tools to model and predict workflow bottlenecks is another innovation with promise. This proactive approach allows for better planning and resource allocation.
Modern workflow systems are beginning to integrate with a wider array of sensors through the internet of things (IoT). This broader connectivity helps track machine health and overall process efficiency. With access to this level of detailed data, more timely maintenance strategies are possible, maximizing uptime and preventing unexpected shutdowns. It's interesting to note how a lot of these newer workflow systems also incorporate machine learning capabilities. These features leverage past production data to improve future performance. The potential for self-optimization within AM processes is significant, though it will be critical to evaluate whether this results in less need for engineers or a shift in their roles.
These new workflow management tools are frequently cloud-based, which is a boon for engineers needing to collaborate remotely. It makes it much easier to access information from different locations and ensure that projects keep moving forward without disruption. Some interesting research suggests gamification within workflows might be an innovative approach to motivate participation and drive productivity. However, it is worth carefully considering the potential impact of such elements on a workplace environment.
While these advancements appear to lead towards a more automated future for AM, it will be critical to address the concerns around adequately training the workforce to leverage the full potential of these advanced systems. It remains to be seen how well these innovations manage the evolving needs of the industry as a whole.
New Advancements in Additive Manufacturing Software A 2024 Analysis of Simulation and Workflow Integration - Enhanced Cloud-Based Collaboration Features for Global Teams
Additive manufacturing, especially when applied to intricate designs and global collaborations, is increasingly reliant on cloud-based platforms for effective teamwork. The ability for teams scattered around the world to work together on projects in real-time is becoming a critical component of the process. We're seeing improvements in cloud-based software which enable multiple individuals to collaborate on projects simultaneously, such as through design platforms or project management tools. These improvements reduce the need to constantly switch between programs, resulting in a smoother workflow and hopefully higher productivity. This increased reliance on cloud-based collaboration offers new ways for teams to work together on complex projects, but also raises concerns. For example, hybrid work setups rely heavily on effective digital communication tools, and there's a growing need for training people on how to best use these tools to foster effective teams. There is a potential for the use of these cloud-based tools to create challenges in maintaining the social aspects of collaboration, which are essential for creating an engaged and productive workforce, especially across multiple time zones and locations. Despite these issues, the push toward more dynamic, real-time cloud collaboration is a trend that is likely to continue, reshaping the ways teams work in additive manufacturing in the future.
Cloud-based collaboration tools are increasingly important for global engineering teams working on additive manufacturing projects. They facilitate real-time access to design changes and simulations, potentially shortening product development cycles significantly. For example, the use of machine learning within these platforms can analyze design iterations across multiple users, uncovering patterns that might be missed by an individual engineer. This ability to pool knowledge and rapidly evaluate designs could revolutionize the optimization process.
Furthermore, the cloud allows access to powerful simulation tools that were previously restricted to those with expensive local computing resources. This could democratize access to cutting-edge technology in developing regions, fostering innovation on a broader scale. The development of communication tools built into the software, enabling annotation and feedback on 3D models, further enhances collaboration and reduces misunderstandings.
These platforms commonly include version control systems that help track changes made by multiple users, decreasing redundancy and confusion when evaluating design choices. Additionally, predictive analytics are becoming more common, with some software now able to forecast potential manufacturing issues before they happen. This is a huge step towards preemptive problem-solving and can save considerable time and resources. It is interesting to see how cloud-based collaboration has also prioritized cybersecurity, ensuring the protection of sensitive data, which is crucial for intellectual property and defense applications.
Improvements in network bandwidth and stability are further allowing data-intensive simulations to be done in real-time during collaboration sessions. This is a game-changer for engineers needing quick feedback on design modifications. Some tools even support augmented reality, allowing teams to visualize 3D designs in their real-world context, sparking better communication and linking digital models with the intended physical application.
While these advancements are promising, it's worth considering whether these gains in collaboration lead to a decrease in the need for highly skilled engineers or if they instead shift the role of the engineer towards oversight and strategic decision-making. The development of the next generation of engineers who can manage the complexity of these systems and maintain human oversight is essential for their successful integration into AM workflows. While the potential for a 40% reduction in product development times is exciting, it's a reminder of the challenges that remain in transitioning to an increasingly automated manufacturing landscape.
New Advancements in Additive Manufacturing Software A 2024 Analysis of Simulation and Workflow Integration - Improved Data Analytics for Quality Control and Production Insights
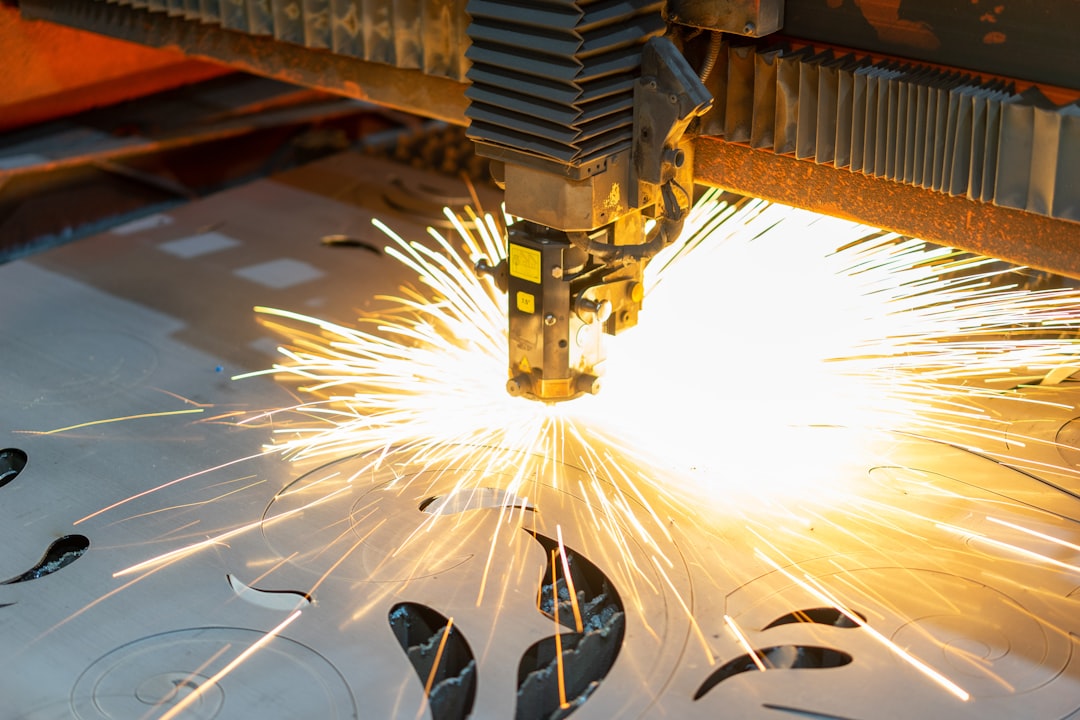
Additive manufacturing in 2024 is seeing a shift towards a data-driven approach, particularly in the realm of quality control and production insights. This change is spurred by the increasing complexity of products, thanks to more customized designs and the growing integration of electronics and software. These developments can lead to unusual and sometimes unpredictable failure patterns, making traditional quality control methods less effective.
Companies are now leveraging data analytics to address this, with the aim of converting large quantities of data into actionable insights. Tools like predictive analytics, powered by AI and machine learning, are being used to anticipate potential issues before they impact production, leading to better decisions and a noticeable improvement in product quality. This is especially useful for dealing with failures that aren't easily understood through traditional means.
While the potential benefits of these analytics tools are evident, there are some concerns that need to be addressed. The increasing complexity of data necessitates a highly skilled workforce that can both manage the data and translate it into insights that are beneficial for improving production. There is a definite need for training and education to ensure the industry has individuals who can fully leverage these new tools. This transition, while promising in its potential to optimize production, does necessitate a change in how organizations operate, moving towards a more data-centric culture that allows them to benefit from these tools in a sustainable and valuable way.
The manufacturing landscape is increasingly driven by data, with a growing emphasis on using analytics to improve productivity, product quality, and sustainability. This is especially true in additive manufacturing, where the ability to customize and produce unique parts has also led to complex and sometimes unpredictable failure modes. Traditional quality control methods are struggling to keep pace with these intricacies, as products now often incorporate intricate electronics and software that can lead to intermittent and difficult-to-diagnose failures.
This challenge is amplified by the digital transformation sweeping across manufacturing. Machines, humans, and products are now interconnected, generating a massive amount of data. Companies are realizing the value of this data for quality control, hoping to translate it into actionable insights that improve production processes. However, simply having data isn't enough; establishing data quality initiatives, like creating a specialized team to manage data quality, is crucial. Without it, we'll see a significant barrier to utilizing AI effectively in manufacturing.
This pursuit of data-driven manufacturing aligns with the broader Industry 4.0 concept. Manufacturing analytics are using operational data and insights from machines to enhance productivity and improve decision-making. The ability to collect data in real time, along with the rise of big data analytics, is also changing the way we manage production and distribution, both crucial functions for successful supply chain operations.
The potential of artificial intelligence (AI) is also being explored. Predictive analytics, which utilize AI methods, offer the ability to identify potential defects early in the process. This helps manufacturers improve decision-making around product quality, potentially leading to fewer faulty parts. Research into the burgeoning Quality 4.0 field has highlighted the need for more research into machine learning and the development of predictive quality control methods. The gaps in our current understanding of how to effectively use these advanced technologies are being researched. This suggests that while we have seen progress in utilizing data, we are still in the early stages of figuring out how to truly maximize its potential in manufacturing.
The use of digital twins – virtual replicas of production environments – is a developing area. By modeling the entire production process virtually, it becomes possible to run simulations and predict potential quality issues before they happen on the physical production floor. This is particularly promising for industries where defects are costly and complex, such as aerospace or medical devices. Although digital twins can provide a more in-depth picture, we still face challenges in seamlessly connecting these digital models with the physical world in a way that generates accurate and timely predictions. The potential of digital twins is considerable, but realizing that potential hinges on addressing the complexity of bridging the gap between the real and virtual worlds.
It remains to be seen how effectively these data-driven approaches can transform additive manufacturing in the long run. There's a risk that focusing too heavily on the technology may overshadow the importance of human expertise. It's critical that as the technology matures, we consider how to integrate it with the knowledge and skills of the workforce, not replace it entirely. While automation holds a significant promise, we must ensure the development and training of individuals who can understand and utilize these tools effectively. Only then can we truly realize the full potential of data-driven manufacturing, without creating new unintended problems in the process.
Revolutionize structural engineering with AI-powered analysis and design. Transform blueprints into intelligent solutions in minutes. (Get started for free)
More Posts from aistructuralreview.com: