Revolutionize structural engineering with AI-powered analysis and design. Transform blueprints into intelligent solutions in minutes. (Get started for free)
Quantum-Inspired Algorithms Revolutionize Turbulent Flow Simulations in CFD
Quantum-Inspired Algorithms Revolutionize Turbulent Flow Simulations in CFD - Tensor Network Algorithms Adapt to Solve Navier-Stokes Equations
Tensor network algorithms are proving to be a valuable tool in the quest to solve the complex Navier-Stokes equations that describe fluid motion. These algorithms, drawing inspiration from quantum mechanics, excel at capturing the intricate relationships within fluid systems, specifically when dealing with turbulence and flow patterns. One of their strengths lies in their ability to efficiently approximate fluid velocity within incompressible flows, relying on mathematical representations like matrix product states. Recent work has demonstrated that these algorithms, particularly when paired with powerful computing like GPUs, can generate swift and accurate simulations of turbulent phenomena, such as two-dimensional turbulence. Looking forward, the inherent adaptability of these algorithms makes them ideal candidates for implementation on future quantum computers, potentially leading to a dramatic leap in computational efficiency compared to current methods. This suggests that tensor networks might revolutionize the field of computational fluid dynamics in the years to come. While still nascent, these developments signal a noteworthy step towards solving some of the most challenging aspects of simulating fluid behavior.
1. Tensor networks offer a way to break down the complex, multi-dimensional information inherent in turbulent flow, allowing for a more streamlined representation of the intricate relationships described by the Navier-Stokes equations. It's like having a new lens for examining these challenging systems.
2. Unlike standard numerical methods, these tensor network approaches draw inspiration from quantum mechanics. This perspective might uncover previously unseen patterns within turbulent flow, patterns that traditional techniques might miss.
3. Applying tensor network algorithms to the Navier-Stokes equations can simplify the computational burden, making it possible to tackle simulations that would be too difficult with traditional methods. It's a refreshing change compared to the limitations of standard techniques.
4. What makes tensor networks flexible is their ability to adapt their structure based on the specific characteristics of the data. This built-in adaptivity helps them better grasp the complex nature of fluid dynamics.
5. Tensor networks can organize the complex features of fluid flow into a layered structure, encompassing both large-scale and small-scale behaviors. This hierarchical representation could lead to a much deeper understanding of the mechanisms that govern flow dynamics.
6. Initial simulations with tensor network algorithms have shown promise in exploring new frontiers in turbulent flow, suggesting the potential for breakthrough insights into irregular flow patterns. It's an exciting area with a lot of potential for new discoveries.
7. Tensor network methods could significantly reduce the time it takes to achieve stable solutions in simulations, which is highly advantageous for applications like aerospace engineering or other fields that demand fast fluid dynamics analyses.
8. The combination of machine learning and tensor networks holds particular promise. It might allow us to extract the governing equations of motion directly from data. This could potentially shift our reliance away from conventional, theory-driven models.
9. Implementing tensor network algorithms isn't without challenges. Getting suitable initial conditions and navigating the complexities of network design pose hurdles, but these difficulties also offer opportunities to develop more sophisticated numerical methods.
10. The Navier-Stokes equations are notoriously nonlinear, a property that makes them challenging to solve. Tensor networks, however, are well-suited to address this nonlinearity, opening up new paths to understanding the chaotic nature of turbulent flows. It could represent a powerful way to shed light on complex, unpredictable systems.
Quantum-Inspired Algorithms Revolutionize Turbulent Flow Simulations in CFD - QFlowS Platform Integrates Quantum Computing with CFD Tools
The QFlowS platform is a novel approach to computational fluid dynamics (CFD), integrating quantum computing into the simulation of turbulent flows. It focuses on utilizing hybrid quantum algorithms to tackle the complexities of the Navier-Stokes equations, particularly within Direct Numerical Simulations (DNS) of turbulent flows. The goal is to bridge the gap between quantum computing and practical applications in fluid dynamics, potentially revolutionizing how we approach complex flow problems.
QFlowS employs a quantum-inspired framework that leverages the strengths of both quantum and classical computers. Quantum computers are utilized for specific optimization tasks within the simulations, while classical computers manage the vast amounts of data generated. This hybrid approach is crucial in pushing the boundaries of CFD by potentially overcoming some limitations of traditional numerical methods.
By integrating these quantum-enhanced simulations into design workflows, researchers hope to refine aerodynamic design and potentially unlock new insights into complex flow behavior. This initiative represents a significant step towards developing innovative computational solutions specifically for challenges in fluid dynamics, an area where quantum computing might offer a distinct advantage. While still in its early stages, the QFlowS project demonstrates the growing interest in exploring how quantum computing can transform the field of CFD.
The QFlowS platform is a novel approach to fluid flow simulations, utilizing the principles of quantum computing (QC) to potentially speed up and improve the accuracy of simulations. This approach could be especially beneficial for real-time analyses where quick and precise results are paramount. Unlike classical simulation methods which may falter when encountering highly chaotic or turbulent flow, QFlowS aims to address these complex scenarios, suggesting the potential for a paradigm shift within computational fluid dynamics (CFD). This endeavor relies on the integration of quantum computing, hoping to leverage phenomena like entanglement and superposition to conquer the 'curse of dimensionality' – a persistent challenge in modeling complex fluid behaviors.
QFlowS employs a hybrid approach, skillfully blending classical and quantum computing components. This strategy makes practical use of current hardware capabilities while also maximizing simulation efficiency. It's a pragmatic approach, acknowledging that while pure quantum solutions are the goal, hybrid approaches may be the more attainable method for the near term. QFlowS is a compelling research initiative that aims to integrate QC into existing CFD tools and pipelines, possibly paving the way for completely new, quantum-inspired methods that could find application in a range of fields. By effectively modeling complex multi-phase flow interactions, QFlowS has the potential to address a wide range of problems across various disciplines, including aerospace, biomedical engineering, and more.
The architecture of the platform allows for future expansion and optimization. As advancements in quantum hardware and algorithms arise, QFlowS can seamlessly integrate them into its framework, maintaining its efficacy as CFD problems become increasingly complex. Some early explorations with the platform show promise for significant reductions in simulation time, which is crucial for industries that prioritize rapid prototyping and design validation. This interdisciplinary initiative brings together experts from physics, mathematics, and computer science to tackle long-standing hurdles in the field of fluid dynamics.
While there's excitement surrounding the potential of QFlowS, there's a certain level of healthy skepticism about its practical applications in real-world settings. However, the underlying premise suggests a novel approach to comprehending and predicting fluid behavior that might lead to breakthrough understandings in the future. The development of specialized quantum protocols to improve the integration of QC into CFD workflows is an important aspect of this research direction. Further exploration of novel algorithms specifically designed for the QFlowS platform could pave the way for a revolution in turbulent flow simulations within CFD, potentially surpassing limitations of traditional computational methods.
Quantum-Inspired Algorithms Revolutionize Turbulent Flow Simulations in CFD - Machine Learning Enhances Reactive Fluid Simulations in CFD
Machine learning is increasingly integrated into computational fluid dynamics (CFD) to improve the simulation of reactive fluid flows. This integration provides new avenues for enhancing simulation accuracy and efficiency. One key benefit is the improvement of turbulence modeling, which is crucial for capturing the complex and often chaotic nature of reactive flows. Machine learning can also speed up direct numerical simulations, which are a computationally intensive method for accurately simulating these systems. Furthermore, machine learning can generate reduced-order models, enabling researchers to simulate highly complex scenarios that traditional CFD methods would find challenging. However, hurdles persist, notably the ongoing need for more robust turbulence models and effective strategies to address the complexity of high-dimensional flow data. Despite these limitations, machine learning shows promise as a vital tool for further advancements in CFD, with a likely expansion of its role in reactive fluid simulations in the years ahead.
Machine learning is increasingly vital for improving computational fluid dynamics (CFD), particularly in handling the complex behaviors of reactive fluids. It offers a powerful way to extract patterns from data that predict how different fluids will react under varying conditions, potentially surpassing traditional CFD models that rely solely on established physics. By utilizing massive datasets and advanced algorithms, machine learning can substantially decrease the computational resources needed for reactive flow simulations, possibly achieving simulation times several orders of magnitude faster than conventional methods.
One of the intriguing aspects of machine learning in this domain is its inherent ability to adapt and refine itself. As new data becomes available, the algorithms automatically improve their predictive capabilities without requiring a complete redesign of the underlying model, offering a degree of flexibility not typically found in classical CFD approaches. Notably, machine learning can provide a more accurate representation of turbulent mixing phenomena, a notoriously challenging aspect of reactive flow modeling. This improvement can lead to significantly more precise simulations across a wide range of applications, such as chemical processing and oil extraction.
Rather than just adding to existing models, machine learning can revolutionize the way we simulate reactive flows. By deriving the governing equations directly from experimental data, it potentially offers a new lens through which to understand fluid dynamics, moving beyond solely applying pre-existing equations. However, this power comes with challenges. High-quality data is essential for training these models. Inaccurate or biased training datasets can lead to substantial errors in predictions, highlighting the critical need for meticulously collected and carefully curated data.
Machine learning models demonstrate a superior ability to handle the nonlinearities present in fluid flow, making them ideal for simulating the chaotic reactive systems found in many practical situations, such as combustion processes and chemical reactors. But a key drawback of this approach is the challenge of interpretability. It can be difficult to understand the reasoning behind a machine learning model's decisions, which can pose problems when ensuring compliance with safety standards or regulatory guidelines. This necessitates a careful balance between leveraging the power of these models and maintaining a level of transparency for critical engineering applications.
Integrating machine learning into established CFD workflows demands a shift in engineering perspectives and skillsets. Engineers must not only possess a strong foundation in fluid dynamics but also cultivate expertise in programming and data science to fully leverage these tools. There is significant potential in the emerging synergy between machine learning and quantum-inspired algorithms. Combining the capabilities of machine learning with quantum phenomena, like those explored within the QFlowS platform, might further enhance the speed and precision of reactive fluid simulations. This promising area could pave the way for a new generation of faster and more insightful simulations.
Quantum-Inspired Algorithms Revolutionize Turbulent Flow Simulations in CFD - Matrix Product State Algorithms Emerge for Large-Scale Turbulence Modeling
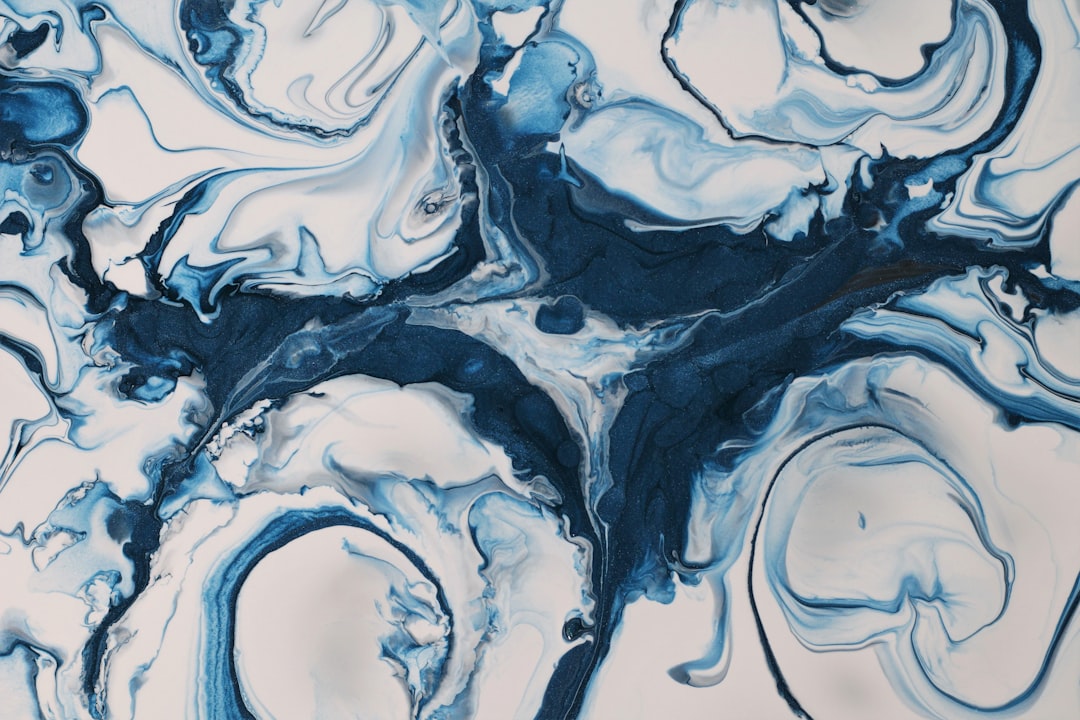
Matrix Product State (MPS) algorithms are showing potential for tackling complex turbulence in computational fluid dynamics (CFD). These algorithms, rooted in tensor networks, transform challenging differential equations like the Navier-Stokes equations into a more manageable format, allowing for a better grasp of the intricate interplay of factors within turbulent flows. Recent advancements in quantum-inspired methods have improved how simulations account for the relationships between different scales of turbulence, suggesting better accuracy, particularly for 2D turbulence. While the benefits of MPS haven't been fully realized for 3D turbulence yet, these methods present a new approach to modeling turbulence, blending classical methods with principles from quantum mechanics. The broad applicability of MPS in areas beyond fluid dynamics, such as modeling electronic structures, suggests a versatile tool with potential for further advancements in turbulent flow simulations and other fields. It is too early to fully understand the potential of this application but it is an interesting area of research. The concept of bond dimension, which influences the size of matrices in the MPS representation, also raises questions about how scalable these algorithms will be when implemented on actual quantum computers. Scaling will be one of the limitations of any future quantum computing applications related to turbulence.
Matrix Product States (MPS) algorithms, a type of tensor network method, show promise for handling the complexities of large-scale turbulence in computational fluid dynamics (CFD). Their strength lies in efficiently representing high-dimensional data, like the information contained within turbulent flows, by breaking it into simpler, interconnected parts. This approach offers a way around the limitations of standard methods, especially when dealing with intricate flow geometries.
The core of MPS's efficiency comes from its ability to compress complex data into a manageable form without losing key information. This is crucial for turbulence simulations, where the sheer amount of data generated can be overwhelming. Notably, MPS can model the interactions between turbulent fluctuations more effectively by drawing upon concepts like quantum entanglement, which describes how parts of a system remain connected even when physically separated.
Recent advancements show that MPS algorithms can now tackle both spatial and temporal correlations in turbulence. This means they can capture not only how turbulent structures are spatially related but also how they change over time, making the simulations much more realistic. Further, MPS methods are dynamically adjustable, allowing them to adapt their structure based on changes in the flow. This adaptability is beneficial for scenarios where conditions are constantly shifting, like those encountered in the design of aircraft or automobiles.
Interestingly, MPS-based methods are proving to be potentially more effective for three-dimensional turbulence simulations than traditional methods, which often face scaling issues. This could lead to a significant breakthrough in addressing the 'curse of dimensionality', a major challenge in CFD. The potential for reduced computational resource usage is also appealing, as it could decrease the cost and time required for simulations.
There's considerable interest in exploring hybrid models that combine MPS with machine learning techniques. The idea is to create more powerful predictive models of turbulence by leveraging the power of MPS to analyze historical simulation data. Researchers hope that through MPS, they can gain a deeper understanding of the chaotic nature of turbulent flows, potentially uncovering hidden principles or scaling laws that were previously obscured by standard models.
However, this transition from traditional CFD to MPS-based methods does present some challenges. One major hurdle is interpreting the results of these simulations. The nature of MPS, inspired by quantum mechanics, can lead to results that are not immediately intuitive. This demands careful consideration and necessitates the development of improved diagnostic tools to validate and interpret the outcomes, ensuring the applicability and reliability of MPS methods in practical engineering problems.
Quantum-Inspired Algorithms Revolutionize Turbulent Flow Simulations in CFD - Hydrodynamic Schrödinger Equation Framework for 3D Turbulent Flows
The Hydrodynamic Schrödinger Equation (HSE) framework offers a novel approach to simulating turbulent flows in three dimensions. It establishes a connection between the principles of fluid dynamics and quantum mechanics, suggesting new ways to understand and model turbulence. This framework leverages a quantum algorithm specifically designed to solve the HSE, providing a potentially powerful method to tackle the complexities of turbulent flow behaviors.
A key aspect of the HSE approach is its ability to potentially address the nonlinearities inherent in the Navier-Stokes equations, which describe fluid motion. The inherent challenge of simulating fluids using quantum techniques remains, but the potential for significant breakthroughs in understanding turbulence makes this a promising area of research.
While the current implementation of the HSE framework faces limitations, it marks a significant development in the application of quantum-inspired techniques to fluid dynamics. The framework encourages further investigation into the intersection of quantum physics and computational fluid dynamics, potentially revealing new insights into the nature of turbulence. This innovative method has the potential to impact how we approach fluid flow problems, particularly within the challenging realm of three-dimensional turbulence.
The Hydrodynamic Schrödinger Equation (HSE) framework offers a novel perspective on simulating 3D turbulent flows by drawing parallels between fluid dynamics and quantum mechanics. This approach, while intriguing, suggests a connection between classical fluid behavior and the probabilistic nature of quantum systems, prompting us to rethink the traditional separation of these fields. It's as if we can now look at turbulent flows with a quantum lens.
This perspective introduces the possibility of utilizing concepts like quantum superposition in fluid simulations. Superposition allows for simultaneous consideration of multiple flow states, which could be instrumental in understanding the complex, often chaotic, interactions inherent in turbulence. This is particularly appealing since classical models often oversimplify these interactions.
Interestingly, the framework reveals symmetries in turbulence, similar to those encountered in quantum mechanics. Understanding these symmetries might pave the way for streamlining turbulence simulations and potentially lead to more accurate predictions.
Further, the HSE allows for the inclusion of nonlocal interactions between fluid particles, a feature generally disregarded in traditional CFD methods. This could improve our ability to simulate how disturbances travel through a turbulent flow. However, despite these promising elements, there are hurdles related to scalability. Applying the HSE to high-dimensional turbulent flow scenarios remains a significant challenge due to computational constraints.
The HSE also holds the potential to inspire the creation of innovative numerical algorithms that leverage quantum computing. This could be a game-changer for solving difficult fluid dynamic problems that currently limit the capabilities of conventional CFD methods.
The ability to incorporate adaptive boundary conditions within the HSE framework is another key advantage. This flexibility is crucial for scenarios where the flow boundaries are constantly changing, like in aeronautical or hydrodynamic applications. Furthermore, the HSE could lead to refined turbulence closure models that provide more accurate descriptions of dynamics across various scales.
Intriguingly, there's a connection between the HSE and the field of statistical mechanics, suggesting that we can approach the understanding of turbulence through the lens of thermodynamics. This connection can potentially provide new insights into energy dissipation and scaling behavior in turbulent systems.
Moreover, the adoption of the HSE framework invites us to scrutinize the standard assumptions underpinning turbulence modeling. This critical approach could lead to the discovery of previously unnoticed phenomena and significant advancements in CFD.
Ultimately, the HSE framework is a stimulating area of research, but it's important to note the need for further development, particularly regarding scaling and computational feasibility for large-scale simulations. There's a lot to be discovered and explored, and this alternative perspective offers a potential path to better understanding and simulating turbulent flows.
Quantum-Inspired Algorithms Revolutionize Turbulent Flow Simulations in CFD - Quantum-Inspired Methods Address Complex Geometries in CFD Simulations
Quantum-inspired approaches are showing promise for improving the accuracy and efficiency of CFD simulations, especially in scenarios involving intricate geometries. Traditional CFD methods often struggle with the computationally demanding nature of turbulent flows, particularly when attempting to solve the complex Navier-Stokes equations analytically. Quantum-inspired techniques, which borrow concepts from quantum physics, aim to tackle these difficulties. Methods such as matrix product states (MPS) and hybrid quantum-classical frameworks provide a way to improve the scalability and computational efficiency of simulations. These improvements allow for better representation of turbulent flow patterns and interactions within complex domains. The faster and more insightful simulations resulting from these methods are beneficial to both research and practical applications in engineering. However, transitioning to these novel approaches still requires overcoming hurdles in scalability and ensuring that the resulting simulations are readily interpretable within conventional CFD workflows.
1. Quantum-inspired approaches, especially those leveraging the Hydrodynamic Schrödinger Equation (HSE) framework, view turbulent flow as a complex system with probabilistic elements, uncovering patterns and symmetries reminiscent of quantum mechanics that were often overlooked in traditional CFD methods. This shift in perspective might offer fresh insights into turbulence.
2. Integrating quantum principles into CFD simulations could allow us to model nonlocal interactions between fluid particles, which are usually disregarded in conventional models. This might lead to improved turbulence modeling, offering a more accurate representation of how disturbances propagate throughout the flow.
3. The adaptability of tensor network algorithms, a key feature absent in many classical methods, allows them to adjust their structure dynamically based on changing flow conditions. This could potentially result in more reliable simulations for turbulent systems that are constantly evolving, making them more useful in a wider range of scenarios.
4. Matrix Product States (MPS), a specific type of tensor network algorithm, can efficiently represent vast amounts of turbulent data by compressing intricate, multi-dimensional relationships into simpler formats. This remarkable efficiency potentially reduces the computational resources needed for these types of simulations significantly.
5. The adoption of quantum-inspired algorithms in CFD necessitates a re-evaluation of long-held assumptions about turbulence, potentially leading to a discovery of new scaling laws relevant to fluid dynamics. It could be a major change in the way we think about turbulence.
6. Initial studies employing MPS for turbulence modeling show promise for reducing computational costs and simulation times, a crucial advancement for industries relying on fast simulations for design and validation, such as aerospace or automotive design.
7. The exciting prospect of integrating machine learning with quantum-inspired turbulence simulations could enable models that not only simulate fluid behavior but also automatically extract governing equations from the data. This possibility has the potential to fundamentally alter how CFD analyses are performed.
8. The HSE framework's unique connection to statistical mechanics allows researchers to explore turbulence through the lens of thermodynamics. This could unlock new understanding about energy distribution and dissipation within turbulent flows—areas where traditional CFD methods might be lacking.
9. Despite their exciting potential, quantum-inspired approaches face significant hurdles related to scalability, particularly when dealing with turbulence in multiple dimensions. Continued research is necessary to improve and optimize these algorithms so they become more useful for real-world engineering applications.
10. The promise shown by hybrid quantum-classical approaches, such as those used in the QFlowS platform, emphasizes the importance of interdisciplinary collaboration. Combining expertise from physics, computer science, and engineering could significantly accelerate progress in turbulent flow simulations.
Revolutionize structural engineering with AI-powered analysis and design. Transform blueprints into intelligent solutions in minutes. (Get started for free)
More Posts from aistructuralreview.com: