7 Spring 2025 Engineering Internships That Combine AI and Structural Analysis Experience
7 Spring 2025 Engineering Internships That Combine AI and Structural Analysis Experience - Google AiGraph Team Rebuilds Structural Analysis Models in Mountain View CA
The Google AiGraph team, based in Mountain View, California, is undertaking a significant project to revamp existing structural analysis models. Their goal is to improve the speed and effectiveness of structural engineering by leveraging the power of artificial intelligence. This involves creating AI-driven tools capable of simulating complex structures with greater accuracy and efficiency than conventional methods. A key aspect of their approach is combining established finite element models with AI algorithms. This hybrid approach could ultimately create more streamlined and efficient workflows within structural engineering, leading to more advanced analytical processes. This push by the AiGraph team underscores a larger trend of integrating AI into the field, which is likely to continue shaping future educational and employment opportunities for engineers interested in this rapidly evolving area. It remains to be seen how quickly and broadly these advancements will be adopted, but the potential impact on the field is undeniable.
It seems the Google AiGraph team in Mountain View is deeply involved in reimagining structural analysis. Their efforts are centered around creating more accurate models using a fusion of machine learning and topology optimization. Interestingly, they've moved beyond static analysis, focusing on real-time simulations which would allow engineers to predict how a structure might behave under different loads during the design phase, a huge shift from traditional post-construction evaluations.
The team’s foray into quantum computing is noteworthy, suggesting a potential to greatly speed up the design process. Their approach to data seems sound, using previous projects as a learning source through transfer learning – in essence, their models learn from past experiences. The collaboration with academic institutions also appears promising, particularly the development of methods to handle intricate geometries and material variations that traditional methods might struggle with.
It’s also encouraging that the Google team has set out to validate their AI-powered approach through comparison with traditional finite element analysis. Ensuring the accuracy and reliability of AI models is crucial. However, some questions arise about the degree of interpretability built into their models. Understanding how and why the AI arrives at its decisions fosters trust and transparency, aspects crucial for wide adoption within engineering.
Their exploration of deep reinforcement learning in structural design is also an intriguing avenue, with the potential to create adaptive structures capable of responding to variable forces – an area that could transform future infrastructure projects. While promising, it's also critical to assess the long-term implications of such advancements and ensure that potential downsides or unforeseen consequences are considered along the way.
7 Spring 2025 Engineering Internships That Combine AI and Structural Analysis Experience - Tesla Austin Factory AI Structural Integrity Monitoring Development
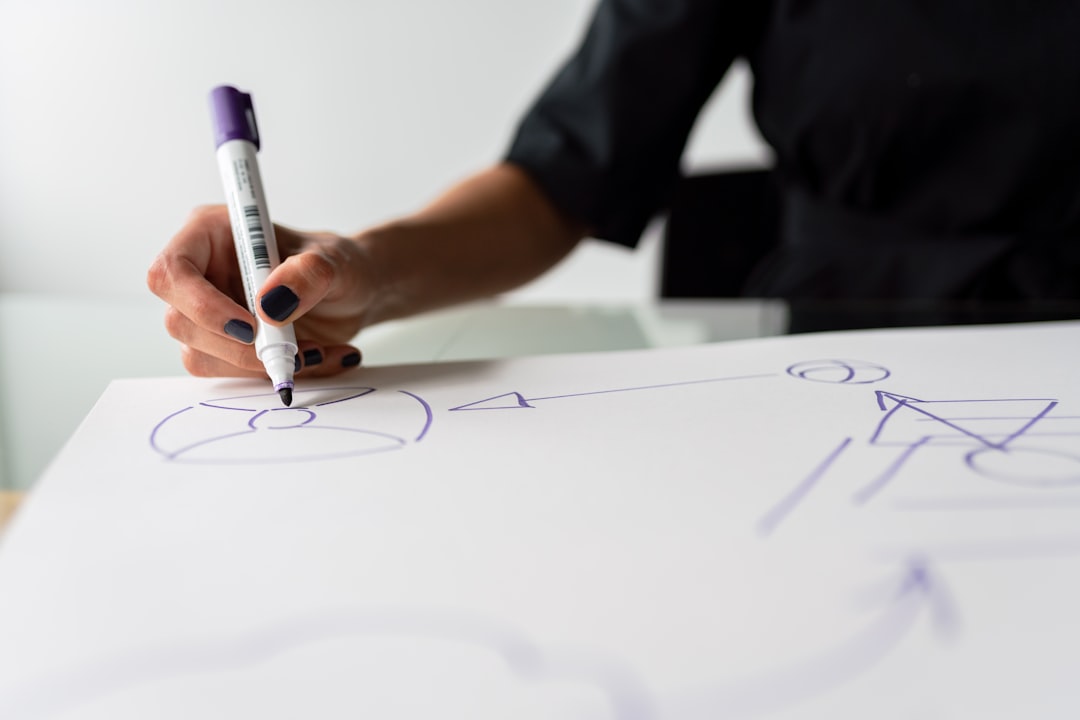
Tesla's Austin factory is developing AI systems to monitor the structural integrity of the facility. This project demonstrates a growing trend of applying AI to improve structural engineering practices. By analyzing large datasets, the goal is to create more efficient and cost-effective designs, potentially leading to better material usage and reduced operational downtime. This AI system is designed to predict and address potential issues before they arise, which is especially crucial for a factory as large and complex as the Tesla Gigafactory in Texas. This approach is meant to ensure the ongoing structural health of the massive facility.
Tesla's internships are positioned to give aspiring engineers hands-on experience with these developments. The company emphasizes creating an inclusive environment and provides support for interns through mentorship and various events. While this initiative is focused on a specific project at a Tesla facility, it reflects a broader shift towards incorporating AI into the design and monitoring of structures. This emphasis on AI within structural engineering could lead to novel design approaches and better long-term performance in a wide variety of structures. However, it is worth noting that while AI presents many opportunities, its potential impacts and limitations need to be carefully considered to ensure its proper and beneficial integration.
Tesla's Austin Gigafactory represents an interesting case study in applying AI to structural integrity monitoring. They've installed a dense network of sensors throughout the facility, aiming for real-time detection of any changes in stress or strain. This continuous monitoring approach is a departure from traditional, periodic inspections, potentially reducing the risk of unexpected structural issues.
The AI aspect involves predictive analytics, using historical data to anticipate potential problems before they occur. This predictive capability can optimize maintenance schedules and help ensure the factory's long-term structural health. Interestingly, they've integrated autonomous drones into the mix, using them for visual inspections of hard-to-reach areas. This approach boosts inspection efficiency and data quality.
The AI algorithms themselves are built on machine learning principles and adapt based on the specific conditions at the Austin facility. This dynamic adaptation is crucial because the factory's structural loads and usage patterns are constantly changing. They've also developed a way to combine data from various sources—environmental, structural health, and even machinery operations—to build a comprehensive picture of the factory's state. This data fusion is then integrated directly into the factory's production workflows, providing a real-time feedback loop for both efficiency and safety.
One intriguing aspect is how the Austin factory benefits from the larger Tesla network. They are leveraging data from other factories to enhance the AI models, potentially accelerating the development and validation of these monitoring systems. It's also notable that the validation process involves simulating extreme operating conditions, which is more realistic than the controlled environments often used in traditional structural testing.
Tesla is also beginning to explore new materials like composites and smart materials in their construction. The monitoring system needs to be flexible enough to handle the data coming from these materials, beyond traditional steel and concrete. The collaboration between engineers and data scientists is crucial here, showcasing a blurring of traditional boundaries in structural analysis. While this focus on AI-driven monitoring offers potential benefits, it's important to consider the long-term implications and the need for transparency in how the AI models reach their conclusions. The interpretability of these complex AI systems is crucial for trust and wider adoption within the structural engineering field.
7 Spring 2025 Engineering Internships That Combine AI and Structural Analysis Experience - SpaceX AI Powered Bridge Design Analysis at Cape Canaveral Launch Complex
SpaceX is recruiting engineering interns for Spring 2025, with a particular focus on AI-driven design analysis at their Cape Canaveral launch facilities. These internships will involve working on structural analysis projects that aim to optimize designs for better performance and easier production, using AI to complement traditional engineering methods. Since Cape Canaveral is a central hub for SpaceX's launch activities, this internship provides a unique opportunity to tackle challenges relevant to both Earth-based and space-related engineering. The interns will contribute to pushing the boundaries of aerospace engineering by applying AI to real-world design problems. While utilizing AI in this manner holds the promise of improved efficiency and results, it's important to acknowledge the need for careful consideration of the reliability of AI models, especially when they're making significant engineering decisions. Aside from the practical experience, interns can expect a number of benefits and the chance to work at the cutting edge of a quickly changing field.
SpaceX's work at Cape Canaveral involves using AI to analyze the design of bridges used during rocket launches. This approach is tapping into a massive dataset of past launches, allowing for more precise predictive modeling of how these structures will hold up during future launches. Instead of relying only on pre-launch assessments, AI is facilitating real-time monitoring of the bridge's health, showing how different parts respond to the dynamic forces present during a launch. This is a shift from the traditional approach that only focuses on static loads.
The AI algorithms built for this project specifically tackle the challenge of understanding how the bridge deals with dynamic forces like vibrations and thermal changes—elements that are often not a major focus in classic engineering assessments. Interestingly, the use of AI simulations can potentially replace a lot of the time-consuming and resource-intensive physical testing that's traditionally done to check fatigue and stress levels in these bridges. Cape Canaveral itself provides a very complex testing ground for structural analysis since the variety of rocket types and payloads creates unique challenges for the bridges. The AI tools are specifically designed to factor in these variables, like different weights and launch dynamics.
The AI system continues to learn as more launches happen at Cape Canaveral, continuously updating its models and making the design process more flexible. This ongoing learning capability allows the AI to improve its long-term predictions, making the overall system more adaptable. It even helps engineers prepare for unexpected events, like severe weather, and understand how these events could affect the bridges' structural integrity.
One of the key aspects of this AI system is how it encourages collaboration between engineers and data scientists, which is a shift from more traditional, siloed design processes. This AI-driven approach stands in contrast to the older methods of bridge design, which often mainly focused on fixed loads. The methods being developed at Cape Canaveral for bridge design could potentially set the stage for future infrastructure projects in the aerospace field, showing us how AI can refine traditional structural engineering practices, enhancing both safety and efficiency in various applications. It's uncertain how rapidly these advancements will impact the field, but it suggests a possible future where traditional methods are blended with machine learning in exciting new ways.
7 Spring 2025 Engineering Internships That Combine AI and Structural Analysis Experience - Boeing Seattle ML Bridge Health Monitoring System Implementation
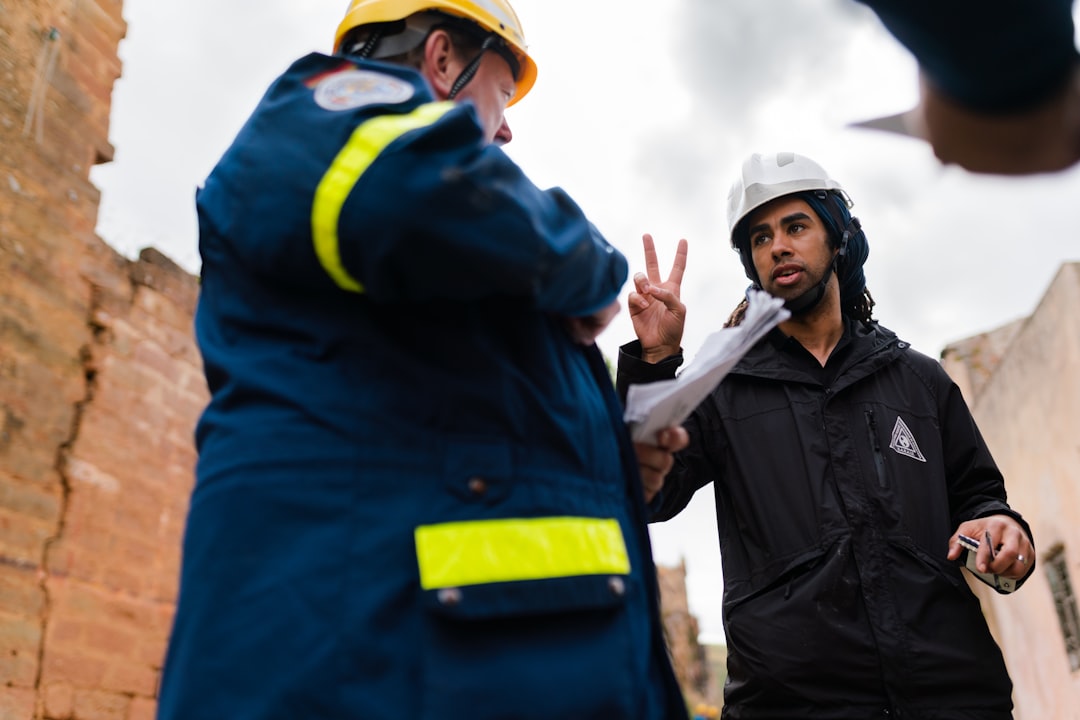
Boeing's Seattle initiative to implement a machine learning-based bridge health monitoring system is a good example of how AI is increasingly being used to keep infrastructure safe. This project focuses on developing a system that uses sophisticated sensors and data analysis to find potential structural problems in bridges very quickly. By creating a kind of "digital twin" for railway bridges, Boeing hopes to improve the ability to predict when maintenance is needed and to improve safety measures, ultimately aiming for the long-term structural integrity of these vital assets. This approach emphasizes the importance of using data-based methods in engineering. It also highlights the need to be attentive to the ongoing challenges of maintaining our aging infrastructure. The extent to which Boeing's work influences future engineering practices and the role of AI in maintaining infrastructure is still developing, but it's a noteworthy area to observe. It will be interesting to see how this influences design and upkeep procedures going forward.
Boeing in Seattle is working on a bridge health monitoring system that uses machine learning. This system aims to automatically detect structural issues in bridges much faster than traditional manual inspection methods. By analyzing a large amount of data from past bridge assessments, it can predict potential failures, allowing for a proactive approach to maintenance rather than just reacting to problems after they occur.
The system relies on sensors placed throughout the bridge to gather data like strain, acceleration, and temperature. This gives a holistic view of the bridge's health under diverse loads and weather conditions. One intriguing feature is how the machine learning models can learn from new data, continuously refining their predictions over time, similar to the advancements seen in fields like airplane health monitoring.
Boeing's approach is a good example of collaboration between civil engineers and data scientists. This ensures the machine learning insights are practical and easy to incorporate into existing bridge management processes. Another important aspect is that the system is designed to be interpretable. Engineers can see how the model makes decisions, increasing confidence in the reliability of its results.
The long-term goal is to shift bridge safety from a reactive to a preventive model. This is expected to reduce costly repairs and lower the risks of failures that could impact public safety. Boeing has also designed the machine learning algorithms to specifically handle the kinds of bridges and materials they use. This shows that a custom-tailored solution might be better than a one-size-fits-all approach in bridge monitoring.
The use of the latest sensor technology is key because it allows for the continuous collection of high-quality data. This data directly improves the accuracy of the machine learning models and their ability to predict future issues. However, some challenges remain, such as the need for robust cybersecurity to protect the collected data and the development of thorough testing procedures to verify the reliability of the machine learning models in real-world situations. While promising, ensuring this system's accuracy and security under the ever-changing conditions of a bridge are areas that need continued focus.
7 Spring 2025 Engineering Internships That Combine AI and Structural Analysis Experience - Lockheed Martin Denver AI Material Testing and Structural Optimization
Lockheed Martin in Denver is exploring how artificial intelligence can improve material testing and structural design, particularly in aerospace. They've set up what they call the "AI Factory" with the intention of using AI to make engineering processes more efficient and effective. This involves bringing engineers, data scientists, and software developers together to fine-tune the way materials are used in structures by leveraging real-time data. The ongoing development of AI techniques in structural engineering has the potential to revolutionize how we design and build things, potentially pushing past older methods. This research is part of Lockheed Martin's bigger goal of making better security solutions in the 21st century, which suggests the need for engineers to become proficient in AI-driven design strategies.
While this initiative seems promising, it is important to scrutinize the implications of using AI in mission-critical applications like aerospace engineering. There may be unforeseen challenges with incorporating AI algorithms into the design and testing of advanced structures, especially when it comes to understanding the decision-making processes within the algorithms themselves. Even if this approach shows promise, it may take time before its applications become widespread. The success of incorporating AI into structural engineering will depend on the level of transparency, reliability, and validation of these AI models.
Lockheed Martin's Denver facility isn't just about building airplanes; they're also pushing the boundaries of how we understand materials and design structures using artificial intelligence. Their work focuses on how material behavior changes under stress, providing insights that traditional methods often miss.
The Denver team's use of AI in material testing is quite interesting, allowing them to adapt experiments on the fly. This means engineers can explore a wider range of variables and combinations, potentially leading to brand new material and structural designs, especially for demanding aerospace applications. It's fascinating how they've incorporated generative design into the process, creating a flood of design options in a short amount of time compared to older methods. This changes the way engineers even think about solving problems.
They've also developed advanced simulation tools that use AI to predict how novel materials will perform under extreme conditions. This gives a more realistic picture of how things might behave in the real world before they are ever built, which is a huge step forward. They've partnered with universities, focusing on understanding "smart materials" that change their characteristics depending on their surroundings. This could pave the way for dynamic structures that react and adjust as needed, a major departure from our current static designs.
One of the unique things they're doing is incorporating real-time data analytics directly into material testing. Instead of relying on past data and static calculations, they can tweak the structure during tests based on immediate feedback. This is quite innovative, allowing for a far more responsive design process. The AI helps them sort through huge datasets from previous tests, uncovering failure patterns that may not have been evident using standard methods.
Denver's team also leverages multi-physics simulations, taking into account multiple factors at once (thermal, fluid, and mechanical aspects) to build a holistic picture of how a structure will function. This is a level of understanding that's hard to achieve with traditional tools. There's a push to ensure AI-driven designs are not only high-performing but also easy to manufacture, making sure cutting-edge designs can actually be built with existing technology. They're also exploring the implications of autonomous material testing with robots equipped with sensors and AI algorithms that conduct experiments with minimal human involvement. This is raising questions about the future role of engineers and how they interact with the generation and interpretation of experimental data. It's definitely a field to keep an eye on.
7 Spring 2025 Engineering Internships That Combine AI and Structural Analysis Experience - NVIDIA Santa Clara Structural Digital Twin Development and Training
NVIDIA's Santa Clara location is offering engineering internships in Spring 2025 that focus on building and training with structural digital twins using artificial intelligence. These internships cover a range of engineering areas, like software and AI, and aim to give students real-world experience with the latest technologies. The internships are designed to connect students with projects that have an impact on industries like gaming, robotics, and healthcare. NVIDIA is pushing inclusivity by providing a 12-week program that incorporates technology and culture immersion, particularly for underrepresented groups. The goal is to educate and equip students with skills that blend AI and structural analysis in an effort to develop innovative approaches to complex problems. This internship initiative shows how AI is likely to play a much larger role in shaping the future of structural analysis and engineering in general, while providing a useful entry point for talented and motivated students to participate in this change. While this presents promising opportunities, the long-term impact and implications of these AI-driven methods are still developing and it's important to watch closely for both positive and negative outcomes.
NVIDIA's Santa Clara facility is focusing on developing and training structural digital twins, an area ripe with potential for improving structural analysis. Their approach involves highly detailed, real-time simulations that can adapt to changing conditions. This means engineers can get a much better understanding of how a structure might perform under different circumstances, making design choices more informed.
Central to this effort is the use of NVIDIA's own GPUs, which allow for lightning-fast computations. This is vital for handling the massive amounts of data that go into complex structural simulations. The team is also incorporating physics-based models into the digital twin framework. These models try to mimic real-world behavior in terms of stress, strain, and vibration, hopefully making assessments of reliability and safety more accurate.
Big data plays a large role, as the digital twin technology relies on large amounts of information. This includes both historical performance data and real-time data from the structure itself. The models use this data to learn and adapt over time, a key feature for structures that experience a lot of variability in loads or conditions. It's interesting that NVIDIA is actively working with academic institutions on these projects, which suggests a two-way street of knowledge exchange and innovative approaches.
They are also involved in training AI models for structural analysis using real-world datasets. This dual-pronged effort of creating the simulation and simultaneously training AI for it suggests a commitment to building a robust and realistic system. Machine learning, specifically neural networks, is being used to boost the performance of the digital twin models, especially in dealing with complex, nonlinear relationships within the data.
One of the goals is to create digital twins capable of providing real-time health monitoring of structures. This approach shifts from reactive maintenance (fixing problems as they arise) to preventative maintenance (anticipating and addressing potential problems). This aspect highlights the potential of the technology to help optimize the lifespan and safety of engineered structures.
The Santa Clara team is promoting a collaborative approach by bringing together engineers, data scientists, and software developers. This multidisciplinary approach is probably important given the complexity of the digital twin technology. It's also encouraging that the model has a mechanism for continuous improvement – it can adapt and learn from new data, which is essential in a field as complex as structural engineering.
These initiatives from NVIDIA are noteworthy. Their ability to simulate complex structures using AI and real-time data creates opportunities for structural engineers. It's an area worth watching, as this approach could transform traditional design and assessment processes, moving beyond conventional methods towards more data-driven and proactive approaches in the future. However, as with any AI-driven system, it's important to ensure the reliability and transparency of the methods.
7 Spring 2025 Engineering Internships That Combine AI and Structural Analysis Experience - Autodesk San Francisco Generative Design for Infrastructure Projects
Autodesk's work in San Francisco focuses on applying generative design to infrastructure projects. This involves using sophisticated algorithms to create numerous design options based on specific requirements set by engineers. The process then evaluates these designs for practicality and performance, refining them to meet specific limitations. One example demonstrated how Autodesk's Civil 3D software and Dynamo, a visual programming tool, can be used together to design a rail maintenance facility. This showcases how the technology can enhance the computational aspect of design and make it easier to automate repetitive tasks. By incorporating AI tools such as Autodesk Fusion, the process becomes faster and can handle complex design constraints, including factors related to sustainable infrastructure. This evolving field of generative design is also increasingly emphasizing the creation of designs that consider environmental factors and help meet goals related to net-zero emissions. This could fundamentally change engineering processes over the coming years.
Autodesk's generative design tools in San Francisco are being used to explore new ways of designing infrastructure projects. It's essentially a way to use advanced computer programs to create many different design options based on what engineers tell them they need. This process involves generating a lot of options, checking how well they'd work in the real world, and then making tweaks to make them better considering various limits, like how much material can be used or how much the structure can bend.
One study showed how these tools could help design a rail maintenance facility. They used a combination of Civil 3D software and Dynamo, a visual programming tool, to get the computer to come up with ideas. It seems this way of designing could potentially automate some of the repetitive tasks that engineers do, as well as help them make sense of complex data sets. They're also aiming to boost creativity by generating ideas engineers might not think of themselves.
Autodesk Fusion is a key part of this, as it's a specific AI tool that gives users more freedom to explore many different designs as long as they meet a certain set of engineering rules. They've actually used these techniques on a fairly large scale—like 15,000 designs for crucial water and power infrastructure—which highlights that it's more than just a concept. The hope is that this kind of design can improve the efficiency and effectiveness of testing different designs, ultimately making building infrastructure faster and more sustainable.
It's not just about design though. AI seems to play a key role in managing the whole project, including timelines, keeping everyone on the same page, and keeping sustainability goals in mind. More recently, these generative design processes have been focused on making infrastructure projects that are more environmentally friendly. It's expected that Autodesk's use of AI will likely change how designs are made in the AEC industries over the next 10 years.
One of the big things that comes up here is the ability to run many more design variations very quickly. The software can do in hours what traditional engineering might take months to do, giving engineers a better chance to see a wider range of possibilities. The software seems to be fairly good at using materials effectively, which means less waste and still keeps the structures strong. These design techniques have been used in real-world projects like tunnels and bridges with designs that are sometimes unconventional, which is interesting.
There is also this ability to take past projects and use that data to help make decisions on new ones. That could help them avoid issues or places where structures fail more often. The whole process is built to encourage collaboration among different fields like engineering, architecture, and construction, which seems to help get everyone on the same page from the very start. And they've also improved the way computer simulations work to better understand how things might behave under loads that change with time, like wind or earthquakes.
Autodesk tries to make the software easy to use, so that people don't need to be expert programmers to get the benefits of generative design. Another plus is that the cost of a project may go down since it can spot potential problems much earlier. The ultimate goal here is to be able to design infrastructure that can adapt to things that happen in the future, which is a crucial consideration when we're thinking about things that are supposed to last a long time.
More Posts from aistructuralreview.com: